The Role of AI in Revolutionizing Electronics Innovation
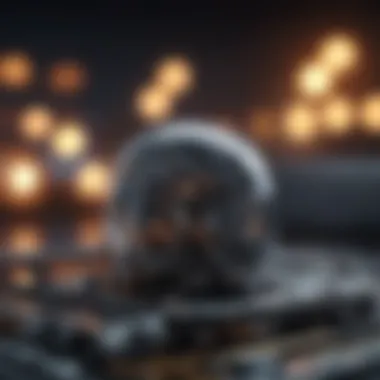
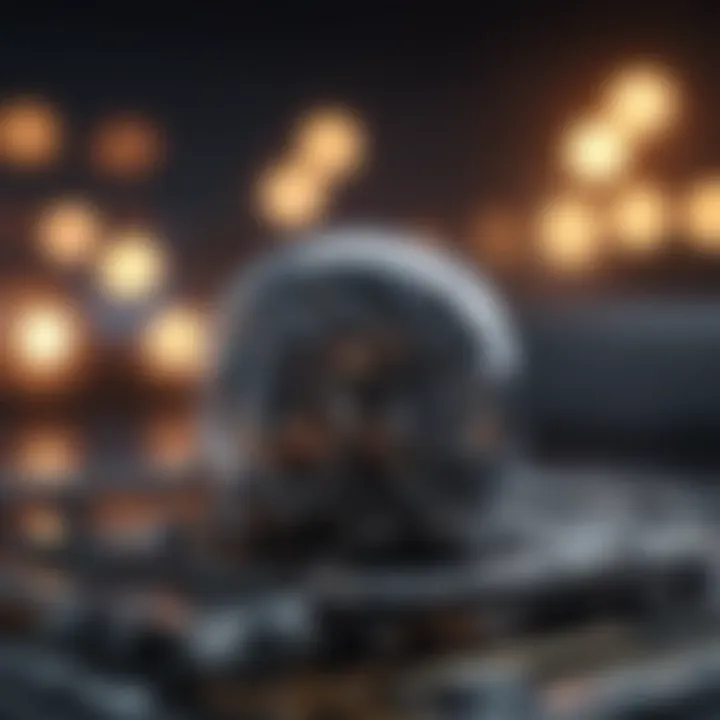
Intro
Artificial Intelligence (AI) is increasingly becoming a focal point of interest in the electronics industry. As new technologies emerge, the integration of AI is transforming not just individual devices but the entire landscape of electronic systems. This section provides an overview of the critical role AI plays in electronics, particularly in enhancing functionality and user experience. The evolution of this relationship is noteworthy, as AI technologies penetrate market-centric customizations and smart functionalities.
Over recent years, numerous industries have begun using AI to analyze data effectively and make informed decisions. The electronics sector is no exception. Key players are integrating AI to drive innovation and enhance efficiency in devices ranging from smartphones to smart home systems. By examining how these technologies interrelate, we gain insights into future trends and possibilities.
An exploration of AI applications showcases its impact through various electronic innovations. From machine learning algorithms that optimize performance to automated systems ensuring real-time processing, AI's contributions are remarkable. The ethical considerations surrounding these advancements also play a significant role, requiring careful thought on the implications for privacy, security, and overall societal impact.
In summary, this article seeks to unravel these complexities and bring forth a comprehensive understanding of how AI functions within the electronics realm.
Prelude to Artificial Intelligence in Electronics
Artificial Intelligence (AI) has emerged as a pivotal technology in the electronics domain. Its significance is not only in enhancing device functionalities but also in creating smarter systems that learn and adapt to user behaviors. By integrating AI into electronics, manufacturers can achieve a higher level of efficiency and performance, which is essential in a rapidly evolving market.
AI tools can process immense amounts of data to refine operations, establish predictive models, and optimize decision-making processes. This ability to leverage data is fundamental, particularly as devices grow more complex and interconnected. AI innovations lead to reduced manufacturing costs, improved reliability, and faster response times in various applications, from consumer gadgets to industrial machinery.
Considerations such as energy consumption, data privacy, and ethical implications are important when applying AI in electronics. It’s vital for stakeholders to address these issues to harness AI's full potential responsibly.
Defining Artificial Intelligence
Artificial Intelligence is a branch of computer science focused on creating systems capable of performing tasks that traditionally require human intelligence. This includes learning from experience, recognizing patterns, understanding natural language, and solving problems. AI systems can be classified generally into rule-based systems, machine learning, and deep learning.
By defining AI clearly, we set the stage for understanding its applications in electronics. The evolution of AI has enabled it to become more efficient and effective in various sectors, particularly in analyzing trends and making adjustments in real time.
The Intersection of AI and Electronics
The relationship between AI and electronics is transformative. Electronics devices have become integral parts of daily life, from smartphones to smart thermostats. At this intersection, AI enhances the functionality of these devices through features such as voice recognition, image processing, and predictive analytics.
- Connectivity: AI enables devices to communicate and share data, which is crucial for the Internet of Things (IoT) applications.
- Personalization: AI algorithms can analyze user behavior to tailor responses and improve user experience.
- Efficiency: AI optimizes processes, leads to energy savings, and minimizes downtime in industrial applications.
This convergence is setting new standards for what electronic devices can achieve, making them not just tools but intelligent assistants.
Understanding Machine Learning and Deep Learning
Artificial intelligence, specifically machine learning and deep learning, plays a crucial role in the evolution of electronics. Both areas contribute fundamentally to the ability of devices to learn from data and make decisions autonomously. These technologies enhance electronics in various ways, making systems smarter, more efficient, and capable of solving complex problems.
Fundamentals of Machine Learning
Machine learning encompasses algorithms and statistical models that allow computers to perform specific tasks without being explicitly programmed. It relies on data patterns and inference to make predictions. This capability is essential in electronics to improve user experience and optimize performance. For example, machine learning is the backbone of features like predictive text in smartphones and recommendation systems in media streaming services.
The primary steps in machine learning include:
- Data Collection: Gathering relevant data from diverse sources.
- Data Preprocessing: Cleaning and organizing the data to make it suitable for analysis.
- Model Training: Feeding the data into an algorithm to enable it to learn from the patterns.
- Model Evaluation: Testing the model on unseen data to ensure it generalizes well.
- Deployment: Implementing the model into a system for real-time predictions.
The benefits of integrating machine learning into electronics are vast. It can lead to improved efficiency, smarter functionalities, and can even predict user behavior for personalized experiences. However, considerations around data privacy and algorithm bias must be addressed continuously as this technology matures.
Deep Learning Techniques Explained
Deep learning is a subset of machine learning that employs neural networks with multiple layers. This structure allows for more complex processing of data and enables the extraction of high-level features. In electronics, deep learning is pivotal in image and speech recognition technologies, making devices smarter and more user-friendly.
Key techniques in deep learning include:
- Convolutional Neural Networks (CNNs): Primarily used for image processing, allowing for advancements in facial recognition and object detection.
- Recurrent Neural Networks (RNNs): Effective in handling sequential data, highly useful in applications like natural language processing.
- Transfer Learning: Involves taking a pre-trained model and adjusting it for a specific task, which is efficient for applications with limited data.
Deep learning delivers significant advantages, such as superior accuracy in pattern recognition and the ability to handle vast amounts of data. Still, it requires considerable computational power and often relies on large datasets for training.
The integration of machine learning and deep learning into electronics represents a pivotal shift toward creating devices that can adapt and improve based on user interaction and environmental changes.
Applications of AI in Consumer Electronics
Artificial intelligence plays a pivotal role in transforming consumer electronics. Its integration into devices not only enhances functionality but also revolutionizes user experience. The adoption of AI results in smarter devices that learn from user behavior, adapt to needs, and provide personalized solutions. This section delves into key areas where AI is redefining consumer electronics, examining smartphones, home assistants, and wearable technology.
Smartphones and AI Integration
Smartphones have increasingly integrated AI to improve performance and user interaction. Features such as virtual assistants, camera enhancements, and predictive text are examples of AI applications within smartphones.
- Virtual Assistants: Siri, Google Assistant, and Bixby help users perform tasks using voice commands. They make it easier to access information hands-free.
- Camera Optimization: AI algorithms analyze the scene in real-time, optimizing settings for better photo quality. These optimizations include adjusting lighting and enhancing image clarity.
- Predictive Text and Emoji Suggestions: The AI learns from user typing patterns, making suggestions that cater to individual preferences, thus speeding up communication.
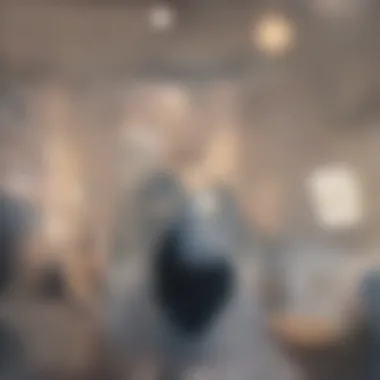
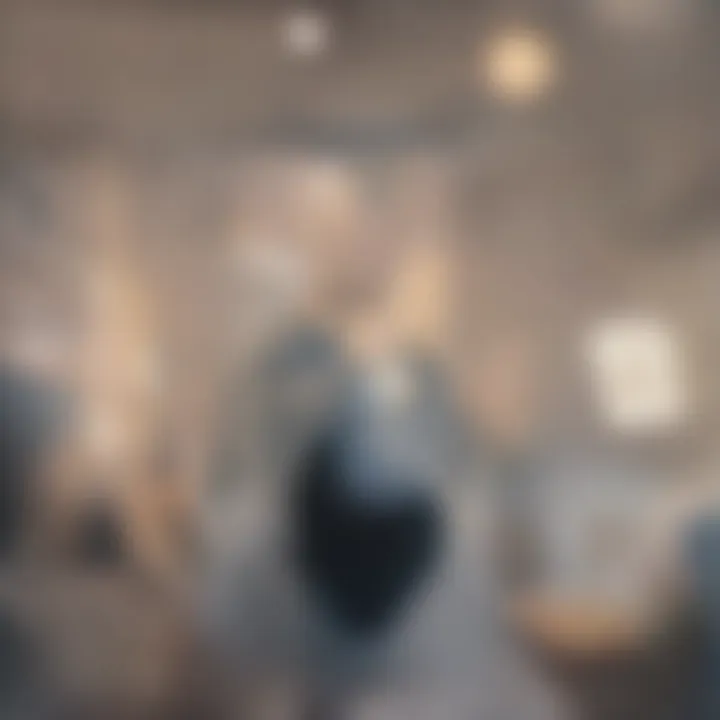
These functionalities contribute to a more seamless user experience, allowing smartphones to feel intuitive and responsive while fostering user engagement.
AI in Home Assistants
Home assistants, like Amazon Echo and Google Home, epitomize the convenience of AI in daily life. These devices rely on machine learning to process commands and streamline tasks.
- Voice Recognition and Natural Language Processing: Home assistants improve in understanding different accents and variations in speech. This allows for more accurate responses to user queries.
- Smart Home Integration: They can control smart home devices, enabling users to manage lighting, security, and temperature efficiently. Users can create routines, automating many daily tasks.
- Personalized Recommendations: Over time, these devices learn user preferences, offering tailored music, news updates, or reminders. The more they interact with users, the better they get at predicting their needs.
Such advancements exemplify how AI transforms homes into intelligent environments, greatly enhancing convenience and connectivity.
Wearable Technology and AI
Wearable technology is another stronghold for AI applications. From fitness trackers like Fitbit to smartwatches like the Apple Watch, AI enhances health monitoring and overall functionality.
- Fitness Tracking: AI processes data from heart rate monitors and accelerometers to provide personalized health insights. For example, suggesting exercise routines based on previous activity levels.
- Health Monitoring: Some wearables can detect irregular heart rhythms or other health issues, alerting users to seek medical attention. The predictive capabilities of AI contribute to proactive health management.
- User Engagement: Many wearable devices learn from user habits, delivering notifications or reminders at optimal moments, thus encouraging healthier lifestyle choices.
Ultimately, AI in wearable technology supports a more informed approach to personal health and well-being. It fosters a culture of engagement and awareness among users, with significant implications for long-term health outcomes.
"Artificial Intelligence is not just a tool; it’s a partner in shaping the future of consumer electronics."
AI Enhancements in Industrial Electronics
Artificial Intelligence is profoundly impacting industrial electronics, offering numerous enhancements that advance efficiency, safety, and productivity. The integration of AI in this field is not merely an enhancement; it has become a key driver in the evolution of manufacturing processes and systems.
Automation and Robotics
The utilization of AI in automation and robotics represents a significant leap forward in industrial capabilities. These technologies help businesses optimize their operations by streamlining workflows and minimizing human error. With the deployment of AI-driven robots, industries can achieve higher precision in tasks ranging from assembly to quality control.
AI systems analyze data and learn from experiences, which enables them to adapt to new situations. For instance, in a production line, a robot equipped with AI can adjust its actions based on real-time feedback, improving overall efficiency. Moreover, the intelligence built into these systems allows for seamless communication between machines, ensuring better synchronization of tasks.
"The true potential of AI in automation lies in its ability to analyze vast amounts of data quickly, facilitating informed decision-making that drives productivity."
Incorporating AI in automation also allows for greater flexibility. Manufacturers can rapidly adjust to changes in demand or adapt to new product lines without significant downtime. This adaptability is a crucial advantage in a market where consumer preferences can shift rapidly.
Predictive Maintenance Solutions
Predictive maintenance is another area where AI has shown significant promise in industrial electronics. Traditional maintenance methods can lead to unplanned downtime, which is costly for businesses. In contrast, AI-enabled predictive maintenance uses data from various sensors and machinery to forecast when a machine will likely fail. This proactive approach minimizes disruptions by scheduling maintenance before a failure occurs.
By analyzing patterns in machine behavior, AI algorithms can identify anomalies that human operators might overlook. This results in not only reduced maintenance costs but also extends the lifespan of equipment. Industries using these predictive solutions tend to experience up to a 30% reduction in maintenance costs and a dramatic decrease in equipment failure rates.
Impact on Electronic Design and Development
The integration of artificial intelligence (AI) in electronic design and development significantly elevates the capabilities of engineers and designers in the industry. This impact is multifaceted, touching on the efficiency of design processes, the ability to predict performance outcomes, and the optimization of resource use. Furthermore, AI technologies offer new methodologies that enhance creativity and innovation in product development.
AI-driven design processes enable engineers to analyze vast amounts of data quickly. This allows for better decision-making during product design phases, leading to streamlined workflows and reduced time-to-market for new devices. With AI tools, designers can generate alternative design options rapidly, which can be evaluated for feasibility and performance. Such improvements not only contribute to faster development cycles but also enhance the overall quality of the final product.
Moreover, the use of AI in design processes facilitates predictive analysis. This means that designers can foresee potential issues in the design before they occur. By simulating different scenarios and outcomes, AI tools can help mitigate risks associated with failures in the design phase. This capability is particularly important in industries where safety and reliability are critical.
AI-Driven Design Processes
AI-driven design processes represent a paradigm shift in how electronic products are conceptualized, created, and refined. Traditional methods often rely on linear approaches, where each phase must be completed before moving on to the next. However, AI allows for more iterative and concurrent processes. This iterative approach fosters innovation by encouraging continuous feedback and refinement.
Some of the key benefits of AI-driven design processes include:
- Enhanced Collaboration: AI tools facilitate better collaboration among teams by providing real-time data sharing and communication.
- Increased Accuracy: By using AI algorithms, designers can improve the accuracy of their designs, which can result in fewer prototypes needing to be built and tested.
- Cost Savings: AI reduces the need for extensive manual labor and material in designing and prototyping, thus saving costs.
Furthermore, integrating AI into design processes encourages the exploration of innovative materials and methods. Engineers are finding ways to incorporate sustainable practices into their designs, focusing on eco-friendly materials and energy-efficient designs. This shift is beneficial not only for the environment but also for companies’ bottom lines, as consumers increasingly favor sustainable products.
Generative Design Principles
Generative design principles leverage AI to explore a broad range of design solutions based on specified constraints and parameters. Unlike traditional design approaches, where designers manually create solutions, generative design allows software to autonomously generate options that meet predetermined goals.
The process typically involves the following steps:
- Input Parameters: Designers input goals, constraints, materials, and manufacturing methods into the generative design software.
- AI Simulation: The software uses algorithms to simulate numerous potential designs, factoring in the input constraints and objectives.
- Evaluating Outcomes: Once the simulations are complete, designers can review the options produced, analyzing their strengths and weaknesses.
This approach opens up possibilities for more efficient designs that might not be intuitive to human designers. The end result is not only a more innovative product but also a significant reduction in design time and resource usage. Notably, companies like Autodesk are at the forefront of generative design technology, promoting its use in various sectors.
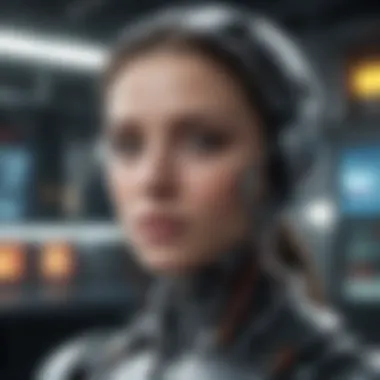
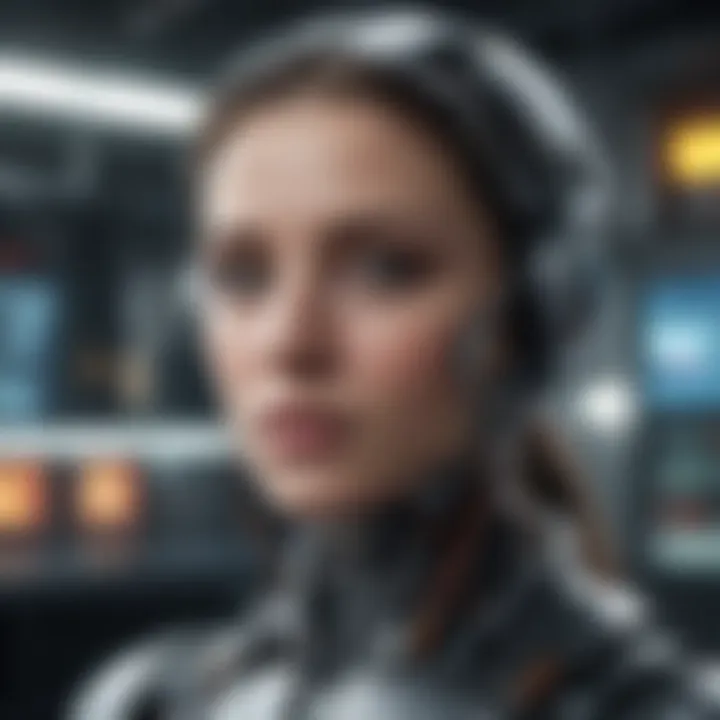
In summary, AI’s role in electronic design and development is transformative. From improving efficiency and accuracy to fostering sustainable practices, AI-driven processes and generative design principles are reshaping the future of electronics. This continuous evolution prompts further research and exploration into the potential that these technologies hold for enhancing engineering practices.
"The mainstream adoption of AI in design fundamentally reshapes how engineers and designers think and work, potentially leading to entirely new approaches in the field."
For more information, you can visit Wikipedia or Britannica.
Data Processing and AI Algorithms in Electronics
The evolution of electronics is increasingly intertwined with the advancements in artificial intelligence (AI). Data processing and AI algorithms have become central to enhancing performance and efficiency across various electronic devices. This section will delve into the significance of data processing and algorithms within electronics, exploring how these components contribute to innovation and improvements in device functionality.
Real-Time Data Processing
Real-time data processing is critical in a landscape where instant information is necessary for effective decision-making. In consumer electronics and industrial applications, the demands for speed and accuracy are paramount. As devices gather large amounts of data, whether from user interactions or environmental sensors, the ability to process this information in real time becomes essential.
Benefits of Real-Time Processing:
- Immediate Response: Devices can react to events as they happen, providing better user experience. Think of smart home devices that adjust settings based on real-time energy consumption data.
- Improved Predictability: In industrial settings, real-time data processing enables predictive maintenance. Equipment can be monitored constantly, predicting failures before they occur.
- Data-Driven Insights: Companies can analyze real-time data for actionable insights, leading to improved strategies and performance optimizations.
This capability relies on robust algorithms that can handle vast data streams efficiently, enabling systems to operate seamlessly while managing complex tasks without latency.
Algorithm Optimization Techniques
The efficiency of AI algorithms significantly impacts the performance of electronic devices. Optimizing these algorithms is essential for ensuring that they run swiftly and accurately without consuming excessive resources.
Key Optimization Techniques:
- Model Simplification: Reducing the complexity of algorithms can lead to faster execution. This includes methods like pruning or quantization, which maintain essential functionalities while minimizing the model size.
- Parallel Processing: Utilizing multicore processors or GPUs enhances processing power, allowing simultaneous execution of multiple algorithms, thus speeding up overall performance.
- Adaptive Learning: Implementing algorithms that adapt based on previous outcomes can improve accuracy and reduce the need for extensive retraining. This technique helps in developing systems that learn continuously as they process more data.
Optimized algorithms provide a better foundation for main AI functionalities, enhancing their practical applications in daily-use devices.
"The advancement in data processing and algorithm optimization is not merely academic; it significantly alters how electronic devices function and interact with users."
In summary, the integration of data processing and AI algorithms is crucial for the advancement of electronics. Real-time processing offers immediacy and relevance, while optimized algorithms ensure that devices run efficiently and effectively. Understanding these elements is vital for anyone keen on exploring the future of electronics driven by artificial intelligence.
AI in Telecommunications
Telecommunications is undergoing a significant transformation with the introduction of artificial intelligence. The integration of AI not only enhances operational efficiency but also improves service delivery in ways previously thought unattainable. This shift affects a wide range of elements within the telecommunications landscape, including network optimization, customer service, and system management. In an era where data traffic is surging, AI is indispensable in managing the complexities involved in handling substantial amounts of data while ensuring optimal performance.
Network Management and Optimization
The concept of network management has evolved with the power of AI technologies. Traditional methods often struggled with scalability and adaptability, which are critical in today’s fast-paced world. AI-driven solutions can analyze vast amounts of data from network operations in real-time. This capability leads to better insights into traffic patterns and usage behaviors, allowing for enhanced network reliability.
Key benefits of AI in network management include:
- Predictive Analysis: By leveraging machine learning algorithms, telecom companies can predict potential outages and performance drops before they happen. This foresight allows for proactive maintenance rather than reactive fixes.
- Automated Monitoring: AI systems can constantly monitor network conditions, detecting anomalies that human operators might miss. This reduces response times and minimizes disruptions.
- Resource Allocation: AI can optimize resource allocation based on real-time demand, leading to reduced operational costs and improved user experiences.
Enhancing Communication Systems
AI also plays a crucial role in enhancing communication systems, making them more responsive to user needs. Systems powered by AI algorithms improve clarity in communications and reduce latency significantly. Here are some elements to consider:
- Chatbots and Virtual Assistants: Many telecommunications firms use AI-powered chatbots for customer service. These tools can efficiently handle a wide array of inquiries, alleviating the workload on human agents and providing prompt responses to customers.
- Improved Call Quality: AI can monitor call quality and apply adjustments instantaneously. This performance refinement translates to clearer conversations and a more satisfying user experience.
- Data-Driven Insights: Communication systems that utilize AI can gather and analyze user feedback to provide tailored services, which helps in maintaining customer satisfaction.
The marriage of AI with telecommunications not only redefines how services are delivered but also creates opportunities for innovation that can lead to new business models and revenue streams.
The intersection of AI and telecommunications is substantial. It catalyzes improvements that enhance efficiency and user experience, marking a critical evolution in this essential industry. As advancements continue, the role of AI in refining communication systems and network management is bound to expand.
Ethical Considerations in AI Technology
Artificial intelligence brings numerous benefits to the electronics industry. Yet, it also fosters serious ethical questions. It is crucial to consider implications that arise as AI integrates more deeply. Ethical considerations in AI technology affect product design, user experiences, and overall trust among stakeholders. Thus, this section aims to illuminate the importance of these ethical elements.
Data Privacy and Security Issues
Data privacy is a foremost concern in AI-driven electronics. Devices continuously collect and transmit user data. This may include personal information, usage habits, and more. As AI systems process this large amount of sensitive data, unauthorized access risks increase. If secured improperly, valuable data can be misused.
Steps to improve data protection are vital. Companies should employ encryption methods and monitor potential vulnerabilities. Additionally, consumers must control their information. Transparency in data usage policies helps users feel secure about their data.
Key Points to Note:
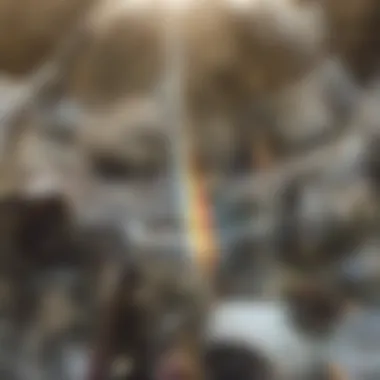
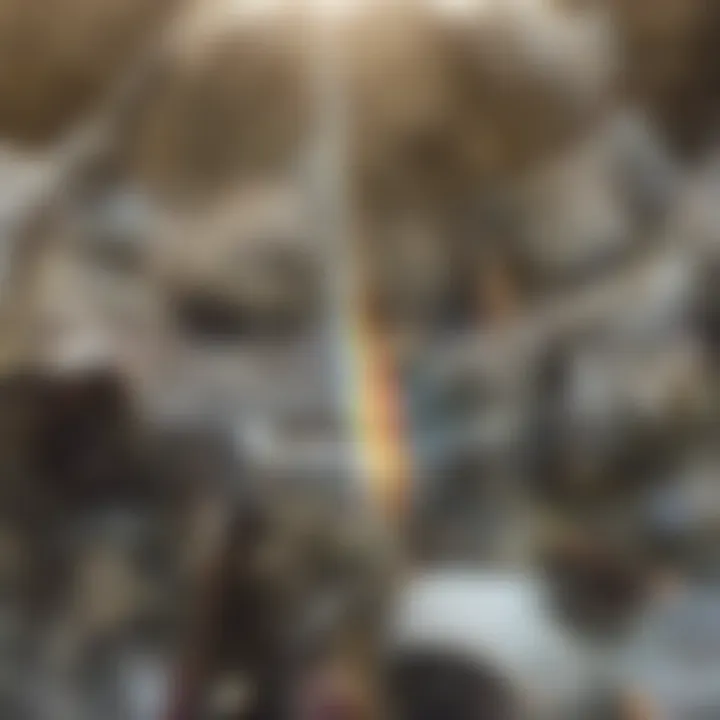
- Regulatory compliance is crucial, as laws evolve.
- Implementing AI systems should include privacy-by-design principles.
- Education for users on privacy rights is necessary.
Proper management of data privacy and security is not just about following laws. It is about earning trust and inspiring confidence among users in AI technology.
Bias in AI Algorithms
Bias in AI algorithms remains a notable challenge. Algorithms learn from historical data. If this data contains biases, the AI system may replicate these prejudices in its decisions. Such biases can affect product recommendations, pricing strategies, and user interactions.
Identifying and mitigating bias requires ongoing efforts. Various methods exist to monitor AI decision-making processes. Techniques like diverse training datasets can reduce these elements of bias. Moreover, researchers must regularly audit algorithms.
Key Strategies to Combat Bias:
- Standardizing data guidelines to ensure fairness.
- Involving diverse teams in the development process.
- Continuously updating systems to reflect changing societal norms.
Addressing bias impacts equity and fairness in technology applications. Companies should also provide accountability. Such measures not only improve the AI systems but also enhance user trust and acceptance.
Future Trends in AI and Electronics
The exploration of future trends in artificial intelligence within the electronics sector is crucial. As AI continues to evolve, its applications and implications become increasingly significant. Understanding these future trends is vital for navigating the coming landscape of electronics. Specific elements, such as emerging technologies, the integration of quantum computing, and sustainable practices, all play a role in shaping this future.
Emerging Technologies
Emerging technologies are at the forefront of the intersection between AI and electronics. Innovations like edge computing, which brings computation and data storage closer to the device, enhance performance and reduce latency. As more devices become smart and interconnected, the need for real-time processing becomes critical.
Examples of emerging technologies include:
- 5G Networks: Enabling faster and more reliable connections for IoT devices.
- Neuromorphic Computing: Mimicking human brain processing capabilities for better efficiency.
- Natural Language Processing: Allowing devices to understand and respond to human language more effectively.
These technologies promise to enhance user experiences and improve operational efficiencies across various electronic platforms. They will not just redefine how devices function, but also influence the design and integration processes within the industry.
The Role of Quantum Computing
Quantum computing stands poised to revolutionize many fields, including electronics. Its ability to solve complex problems at unprecedented speeds can lead to breakthroughs in AI applications. With the capability to process vast amounts of data simultaneously, quantum computers could significantly enhance machine learning algorithms and AI decision-making processes.
Key aspects of quantum computing's impact include:
- Accelerated Data Processing: Solving intricate problems in shorter timeframes.
- Improved AI Algorithms: Enabling more sophisticated models for better predictions.
- Enhanced Security Protocols: Offering stronger data protection through advanced encryption.
As the technology matures, integrating quantum computing into electronics may open new avenues for innovation, transforming industries such as healthcare, finance, and telecommunications.
Sustainable Electronics and AI
Sustainability is a growing concern in the electronics industry. Combining AI with sustainable practices can lead to significant improvements in energy efficiency and resource management. AI technologies can optimize electrical consumption in devices and smart grids, reducing overall carbon footprints.
Considerations for sustainable electronics include:
- Energy Efficiency: AI can help create energy-efficient devices, decreasing consumption.
- Lifecycle Management: AI can monitor and manage the lifecycle of products to minimize waste.
- Resource Optimization: AI techniques can enhance the use of raw materials in manufacturing processes.
Embracing sustainability through AI not only benefits the environment but also aligns with increasing consumer demand for responsible technology. In the long run, it could redefine industry standards and practices.
The intersection of AI and sustainable practices marks a pivotal shift in how the electronics industry approaches environmental challenges, paving the way for a more responsible future.
Ending: The Evolution of AI in Electronics
The evolution of artificial intelligence (AI) in electronics marks a significant milestone in technology's trajectory. As we have explored throughout this article, AI is not merely an enhancement; it is becoming a fundamental element that underpins various innovations in the electronics sector. The convergence of AI technologies with electronic devices creates pathways for unprecedented efficiency, user experience, and development strategies.
Importance of AI Evolution in Electronics
AI's integration has transformed the capabilities of consumer electronics. For examples, smartphones now leverage AI for better photography, predictive text, and customized user experiences. Home assistants powered by AI adapt to individual preferences, creating smarter living environments. Wearable devices monitor health metrics using sophisticated algorithms, making them indispensable for personal wellness.
Key Benefits:
- Efficiency Gains: AI algorithms analyze data swiftly, leading to intelligent automation in industrial settings. Likewise, predictive maintenance minimizes downtime, increasing productivity.
- Innovative Solutions: AI pushes the boundaries of what is possible with electronics. The introduction of generative design in electronic development opens new realms in product design.
- Quality Improvements: Enhanced data processing capabilities ensure higher quality of products and services, impacting sectors from telecommunications to consumer electronics.
"The ability of AI to learn from data and optimize performance is reshaping the landscape of electronics, making it more responsive to user needs and market demands."
Considerations:
However, with these advancements come ethical considerations. Data privacy issues arise when devices collect user information without adequate protections. Moreover, bias in AI algorithms can lead to unintended consequences, impacting the fairness of automated decisions.
As we consider the future, the role of AI in electronics will likely expand. Quantum computing, sustainable technologies, and continuous improvements in machine learning will further influence electronic design and functionality. Staying abreast of these developments is imperative for students, researchers, educators, and professionals who seek to navigate this complex yet exciting domain.
In summary, the evolution of AI in electronics is a comprehensive phenomenon. It embraces innovation while posing challenges that necessitate careful consideration. Understanding these dynamics will empower future practitioners and inform ongoing discussions about the ethical deployment and responsible integration of AI technologies in electronics.