Biomedical Data Analysis: Transforming Healthcare Insights
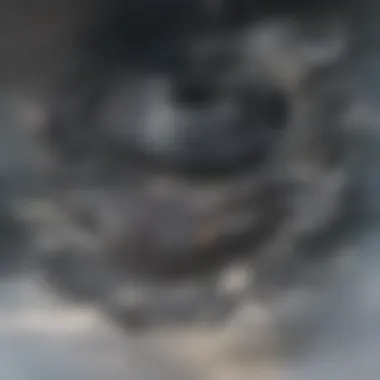
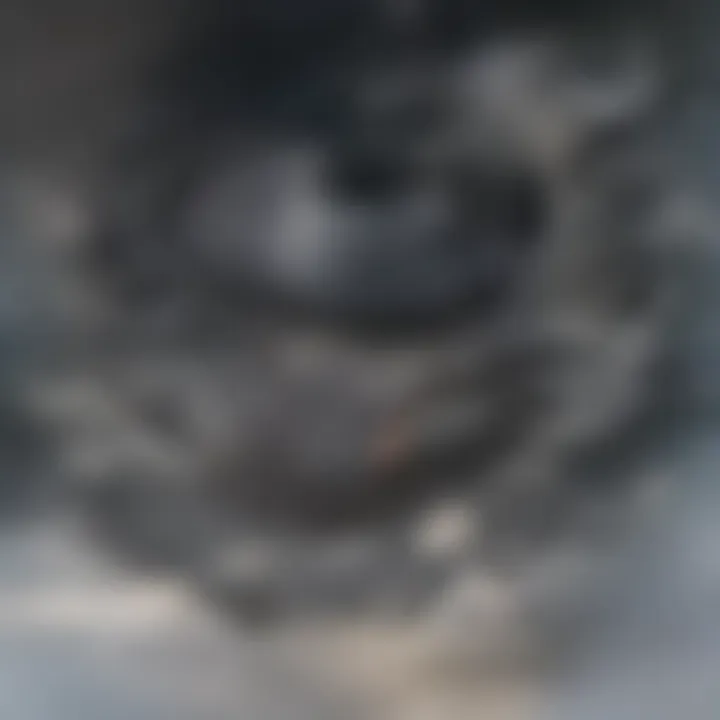
Intro
The world of healthcare is rapidly transforming, largely influenced by the integration of data-driven methodologies. Biomedical data analysis is at the forefront of this change, providing pathways to enhanced patient care, scientific innovation, and better clinical practices. As society moves towards a more empirical approach in medical science, understanding how data is leveraged becomes crucial for various stakeholders, including students, researchers, educators, and professionals.
In this discussion, we will investigate the multifaceted aspects of biomedical data analysis. The discourse will cover essential methodologies, technologies, and real-world applications. Furthermore, it will dive into the ethical considerations and challenges that accompany the analysis of medical data. The ultimate objective is to instill a nuanced comprehension of the significant roles that data analytics play in shaping patient outcomes, aiding clinical decisions, and propelling scientific research forward.
Research Methodology
Description of Research Design and Approach
In biomedical data analysis, a systematic research design is crucial. This typically involves both qualitative and quantitative research approaches. Qualitative research might entail interviews or focus groups with healthcare professionals, while quantitative methods often include large-scale data analytics from electronic health records. Researchers adopt a mixed-methods approach to fully capture insights from multiple angles, enhancing the richness of the data.
Materials and Methods Used in the Study
The choice of materials and methods in biomedical data analysis can vary widely based on the specific question or hypothesis being tested. Common materials include:
- Electronic health records
- Laboratory results
- Genomic sequences
- Patient surveys
Methods utilized often encompass:
- Machine learning algorithms for predictive analytics
- Statistical software for data interpretation
- Data visualization tools for better understanding of findings
It is essential to follow ethical guidelines, particularly regarding patient consent and data privacy, when conducting these analyses.
Discussion and Interpretation
Interpretation of Results in the Context of Existing Literature
When the results of biomedical data analysis are published, they must be interpreted within the framework of existing literature. This involves comparing new findings to previous studies, identifying trends, and recognizing discrepancies. Such comparisons can validate new research or highlight areas needing further exploration. For instance, recent studies could show advancements in predictive analytics, thus influencing treatment protocols.
Implications for Future Research or Practical Applications
The findings from biomedical data analysis have profound implications for future research. Not only do they inform decisions on treatment strategies, but they also open avenues for new investigations into disease mechanisms, prevention practices, and healthcare policies. The potential for practical applications in clinical settings enhances the relevance of ongoing and future studies. As healthcare continues to embrace data-driven approaches, the implications for patient care and outcomes are increasingly significant.
"Data-driven healthcare is not just a trend; it is a fundamental shift that enhances decision-making at every healthcare level."
By understanding the core principles of biomedical data analysis, stakeholders can better navigate this complex but essential domain. As we move forward, it is vital to remain aware of both the opportunities and challenges presented by this evolving field.
Preamble to Biomedical Data Analysis
Biomedical data analysis represents a cornerstone of modern healthcare, facilitating a deeper understanding of biological phenomena through rigorous data examination. This analysis encompasses various types of data generated in healthcare and research, including but not limited to genomic sequences, clinical records, and imaging data. The process of gathering, organizing, and interpreting this data is crucial in deriving actionable insights that can shape patient care and clinical practices.
The importance of biomedical data analysis lies primarily in its ability to transform raw data into meaningful information. It allows healthcare professionals to identify patterns and correlations that may not be immediately evident, thus guiding decision-making processes. For instance, through statistical analysis and machine learning techniques, healthcare systems can optimize treatment plans based on patient-specific data, enhancing the effectiveness of interventions.
Moreover, biomedical data analysis contributes to predictive analytics, enabling clinicians to forecast disease outbreaks and patient outcomes more accurately. This is particularly relevant in an era where personalized medicine is gaining traction, emphasizing tailored approaches to individual patient needs.
The ethical dimensions of biomedical data analysis also merit consideration. Handling sensitive patient information requires strict adherence to privacy standards and ethical principles. Understanding these facets can help professionals navigate the complexities associated with data collection and usage, ensuring that patient trust is maintained.
In summary, biomedical data analysis serves as a bridge between complex data sets and practical healthcare applications. Its significance goes beyond data processing; it actively influences healthcare policies, enhances scientific research, and ultimately contributes to improved patient outcomes.
The Importance of Biomedical Data Analysis
Biomedical data analysis is essential for advancing healthcare practices and improving patient outcomes. This discipline not only supports evidence-based medicine but also enhances the precision of diagnoses and treatment protocols. By analyzing vast amounts of data generated from genetic testing, clinical trials, and electronic health records, professionals can derive meaningful insights that inform clinical decisions and foster preventative care strategies. The integration of data analysis into biomedical research has become a pivotal component in understanding diseases and developing treatments.
Impact on Healthcare Outcomes
The influence of biomedical data analysis on healthcare outcomes cannot be overstated. The application of data analytics leads to better patient care through informed decision-making. By utilizing data, healthcare providers can identify at-risk populations and tailor interventions accordingly. For instance, analyzing trends in patient response to treatments allows for adjustments in therapy that can significantly improve efficacy. Moreover, healthcare systems can optimize resource allocation by understanding patterns in disease prevalence. This leads to a more efficient use of available healthcare resources, ultimately improving care quality.
One study found that hospitals employing advanced analytics saw a reduction in readmission rates by over 20%, demonstrating a direct correlation between data-driven insights and enhanced patient care.
"Data analytics transforms raw numbers into actionable insights, a key factor in modern healthcare."
Role in Precision Medicine
Precision medicine is a tailored approach that considers individual variability in genes, environment, and lifestyle for each person. Biomedical data analysis is the cornerstone of precision medicine, enabling healthcare providers to design customized treatment plans that correspond to unique patient profiles. Through genomic data analysis, clinicians can identify specific mutations that may influence a patient's response to medication.
The utilization of machine learning algorithms in mining large datasets allows for the prediction of how specific patients will respond to different treatments. This capability not only enhances treatment effectiveness but also minimizes adverse drug reactions. As a result, patients receive optimal therapies that align closely with their biological makeup rather than relying on a one-size-fits-all approach.
Types of Biomedical Data
Biomedical data serves as the backbone of analysis and interpretation in the healthcare field. The different types of biomedical data contribute significantly to understanding disease mechanisms, patient behaviors, and treatment outcomes. Each type of data offers unique insights and perspectives, making it crucial for researchers and professionals to grasp these various categories. This section aims to highlight the different types of biomedical data, plus their respective implications for analysis.
Genomic Data
Genomic data refers to information derived from an organism's genetic makeup. It includes sequences of DNA, gene expression profiles, and information from epigenetic modifications. The significance of genomic data is profound; it not only aids in understanding genetic predispositions to diseases but also assists in disease prevention and treatment personalization.
By analyzing genomic data, researchers can identify biomarkers for various conditions, paving the way for targeted therapies. Furthermore, advancements in sequencing technology have dramatically decreased costs, making genomic studies more accessible.
However, the analysis of genomic data poses challenges too. Issues related to data quality, harmonization, and integration remain prevalent. Ethics also plays a crucial role, especially regarding privacy concerns surrounding genetic information.
Clinical Data
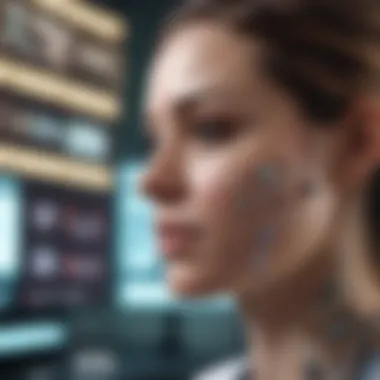
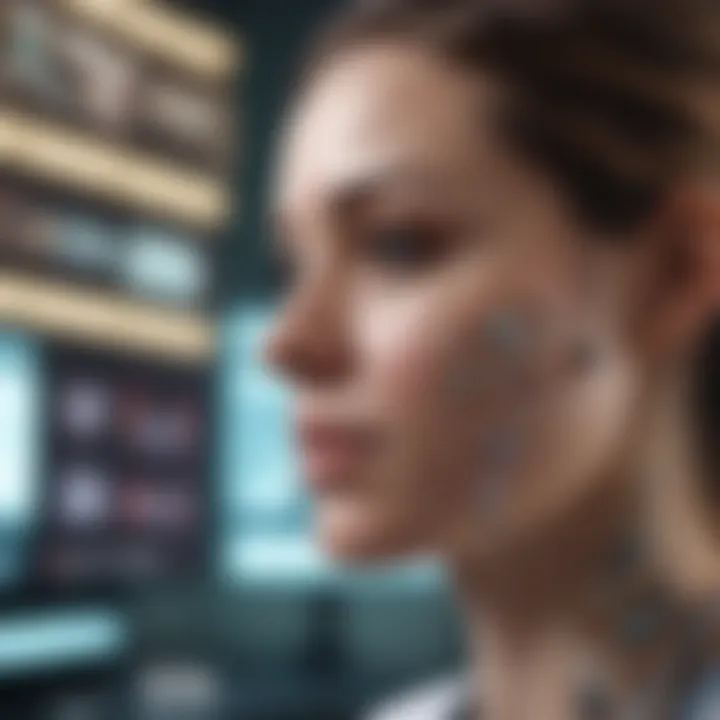
Clinical data encompasses information collected from patient interactions within healthcare settings. This includes patient histories, laboratory test results, medication usage, and clinical outcomes. From a research perspective, clinical data is invaluable for understanding patient populations and outcomes.
Using clinical data, healthcare providers can assess treatment effectiveness, establish clinical guidelines, and improve patient care. Moreover, the insights gained may lead to significant breakthroughs in disease management and healthcare policy.
That said, the reliance on clinical data presents hurdles. Variabilities due to differing standards among institutions can cloud analyses. Furthermore, missing, incomplete, or erroneous data can undermine the reliability of results.
Imaging Data
Imaging data includes a variety of visual representations of biological structures and processes. Common techniques involve X-rays, MRI, CT scans, and ultrasound, which generate critical information for diagnosis and treatment planning. The analysis of imaging data has transformed diagnostics, offering non-invasive methods to monitor disease progression and treatment effects.
Advanced imaging techniques can provide real-time insights into physiological changes in patients. However, interpreting imaging data requires significant expertise. There is also the challenge of harmonizing data from different imaging modalities and ensuring that the classification of images reflects true clinical relevance.
Omics Data
Omics data represents a collection of high-throughput data generated from various biological sources. This includes proteomics, metabolomics, transcriptomics, and lipidomics. Omics approaches allow for a comprehensive understanding of biological systems at multiple levels, providing insights that single-entity analyses cannot.
The integration of omics data is crucial for systems biology; it reveals interactions among various biological molecules and pathways. The challenge here, however, lies in the complexity of datasets and the computational power needed to analyze them effectively. Additionally, data interpretation is a nuanced process, requiring a deep understanding of biological interactions.
The End
Understanding the types of biomedical data is essential for professionals in the field. Each category provides distinct benefits and analytical routes. However, challenges remain in ensuring data quality, privacy, and applicability in healthcare settings. A comprehensive approach to biomedical data analysis relies on effectively utilizing these data types to enhance patient care and advance scientific knowledge.
Data Collection Techniques
Data collection techniques form the backbone of biomedical data analysis. They enable researchers and healthcare professionals to gather the necessary information to inform clinical decisions and advance scientific understanding. The increasing complexity and volume of data necessitate sophisticated methods for obtaining and handling this data. Effective collection techniques directly impact the quality, relevance, and reliability of data, making it essential to choose wisely.
Surveys and Questionnaires
Surveys and questionnaires are critical tools for collecting subjective data in biomedical studies. They allow for the assessment of patient experiences, perceptions, and health-related behaviors. The effectiveness of these tools depends largely on their design. A well-constructed survey can yield rich insights, yet poorly worded questions may lead to biased or unreliable results.
When designing surveys, it is important to consider the target population and their specific needs. Engaging participants and obtaining honest responses are key factors. To enhance response rates, researchers often use online tools or mobile applications. These platforms can streamline the process, making it convenient for participants to contribute their information.
Additionally, surveys must adhere to ethical standards, ensuring that informed consent is obtained. Maintaining participants' anonymity can also increase the likelihood of honest responses, accentuating the need for careful data handling.
Wearable Technologies
Wearable technologies represent a significant advancement in bio-data collection. Devices like smartwatches, fitness trackers, and medical monitors facilitate continuous, real-time data capture from individuals. These tools enable the collection of diverse data, such as physical activity levels, heart rates, and sleep patterns, providing a comprehensive view of a patient's health over time.
The main benefit of wearable technologies is their ability to gather objective data without much intervention. This continuous monitoring contributes to more accurate assessments and personalized treatment plans. However, it is vital to consider data privacy, as sensitive health information is often collected. Researchers must navigate the balance between technological benefits and ethical considerations surrounding user consent and data protection.
Clinical Trials
Clinical trials are a fundamental aspect of biomedical data collection, aimed at testing the efficacy and safety of new treatments or medical devices. These trials involve detailed protocols to ensure that data is collected systematically. Participants are recruited based on specific criteria, and all interactions are documented to maintain the integrity of the research.
The stages of clinical trials provide a structured approach to understanding how treatments function in real-world scenarios. From Phase I, assessing safety in a small group, to Phase III, which evaluates efficacy on a larger scale, each stage requires rigorous data collection methodologies.
The power of clinical trials is their rigor and ability to produce statistically significant results. However, challenges such as participant recruitment, adherence to protocols, and ensuring data quality can pose threats to the overall success of the study. Addressing these challenges is crucial in ensuring that the data collected contributes positively to the broader field of biomedical research.
Clinical trials generate critical insights that shape treatment paradigms; thus, understanding and optimizing data collection methods is essential.
In summary, the methods employed for data collection in biomedical analysis are vital for the overall accuracy and utility of health data. Surveys, wearable technologies, and clinical trials each have unique strengths and challenges, but together they illustrate the complex yet rewarding landscape of biomedical data analysis.
Key Methodologies in Biomedical Data Analysis
Biomedical data analysis has become a cornerstone of scientific progress within the healthcare realm. The methodologies applied in this field are vital in processing vast amounts of complex data, enabling researchers and practitioners to draw meaningful insights. Key methodologies encompass various techniques that, while distinct, often intersect in their application, contributing to a comprehensive understanding of biomedical data. This section highlights three critical methodologies: statistical analysis, machine learning techniques, and bioinformatics approaches.
Statistical Analysis
Statistical analysis serves as a foundational methodology in biomedical data analysis. It involves the use of mathematical techniques to evaluate and interpret quantitative data. Researchers apply statistical methods to draw inferences from sample data and make predictions about larger populations. The importance of statistical analysis lies primarily in its ability to identify trends, correlations, and potential causations in data sets.
For instance, when evaluating clinical trial results, statistical tests help determine whether observed effects are significant or due to random chance. Common statistical methods include regression analysis, t-tests, and ANOVA. Each method has its application depending on the data structure and research question.
Moreover, statistical analysis provides critical value in hypothesis testing, where researchers can verify or refute assumptions based on data. When accurate, the results can support clinical decision-making and ultimately improve patient outcomes.
Machine Learning Techniques
Machine learning techniques represent a cutting-edge approach to analyzing biomedical data. This domain utilizes algorithms to recognize patterns and learn from data without explicit programming. The relevance of machine learning is particularly evident in the era of big data, where traditional analytical methods may fall short due to the data's sheer volume and complexity.
Machine learning can be categorized into supervised, unsupervised, and reinforcement learning. Supervised learning, for instance, is applicable when predicting patient outcomes based on input features such as demographics or treatment histories. Algorithms like decision trees and support vector machines play significant roles in these predictions.
Unsupervised learning, on the other hand, is valuable for discovering hidden structures in data. Clustering techniques, like k-means, can categorize patients into groups based on similarities, guiding personalized treatment strategies. Ultimately, machine learning enhances predictive analytics, enabling healthcare providers to forecast disease progression or treatment responses more accurately.
"Machine learning is not just a method; it is a pivotal contributor to the future of data-driven medicine."
Bioinformatics Approaches
Bioinformatics approaches integrate biology, computer science, and information technology. This methodology is essential in managing and analyzing biological data, particularly genomic data, which is rapidly expanding. Bioinformatics focuses on the development of algorithms and software tools to understand biological data more meaningfully.
One of the areaโs pioneering applications is analyzing DNA sequences. Bioinformatics tools can align DNA sequences, annotate genes, and predict protein structure and function. These capabilities are crucial in genomic research, where understanding genetic variations can lead to breakthroughs in personalized medicine.
Additionally, bioinformatics plays a significant role in drug discovery. By predicting which drug compounds might be effective against specific diseases, researchers can streamline the development process, reducing time and resources needed for testing. This integration of data is increasingly relevant in a world that demands swift and efficient medical advancements.
In summary, each methodology in biomedical data analysis contributes uniquely to the overall field. Statistical analysis provides the groundwork, machine learning leverages complexity, and bioinformatics facilitates innovative breakthroughs. Together, they form a comprehensive toolkit for tackling the challenges presented by modern biomedical data.
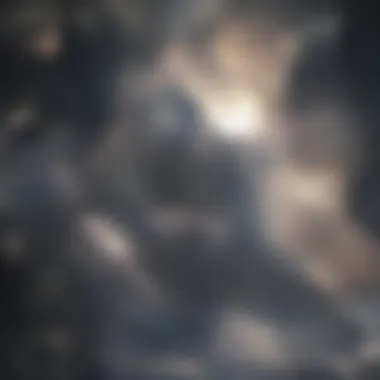
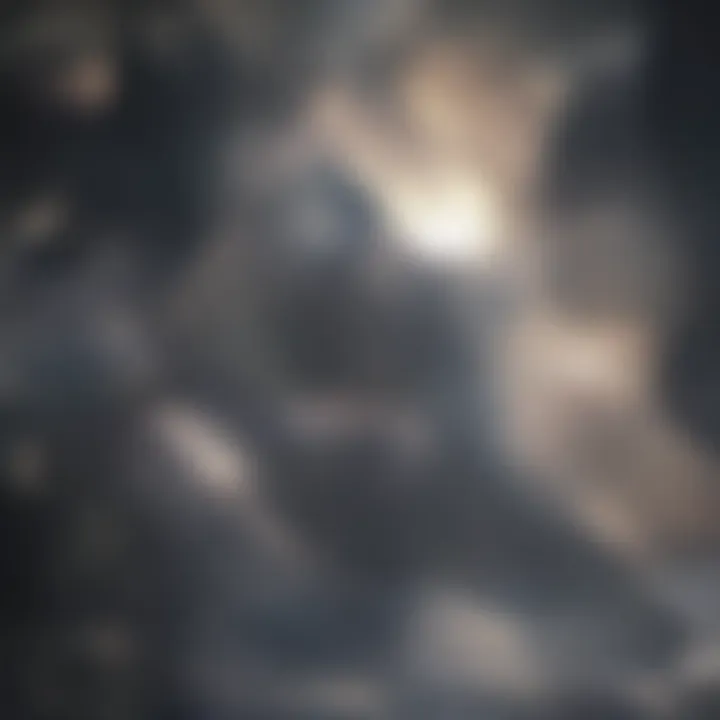
Data Visualization Strategies
Data visualization strategies play a crucial role in biomedical data analysis. In a field characterized by large volumes of complex data, effective visualization techniques allow researchers and practitioners to interpret the information quickly and accurately. By transforming data into graphical formats, these strategies facilitate better understanding, revealing insights that textual data alone might obscure. Visualization minimizes the cognitive load on the viewer and enables more informed decision-making.
Before diving into techniques, it is important to note that effective data visualization also requires an understanding of the audience and the context in which the data will be presented. Key considerations include clarity, accuracy, and aesthetic appeal. Poorly executed visualizations can lead to misinterpretation, potentially misguiding healthcare decisions.
The benefits of robust data visualization strategies are manifold. They include:
- Immediate insight into trends and anomalies that may require further investigation.
- Easier communication of complex data to diverse audiences, including stakeholders who may lack a technical background.
- Enhanced collaboration, as visualizations can serve as common ground for discussion among interdisciplinary teams.
Effective visualization strategies not only enhance understanding but also support data-driven decisions in clinical settings, maximizing the potential benefits of biomedical data analysis.
Graphical Representation
Graphical representation is a foundational element of data visualization strategies. This technique involves presenting data in various formats such as charts, graphs, and plots. The choice of graphical format depends on the type of data and the message that needs to be conveyed. Common formats include:
- Bar charts for comparing categorical data.
- Line graphs for showing trends over time.
- Scatter plots for illustrating relationships between two quantitative variables.
- Heat maps for visualizing complex data matrices.
Each format has advantages in highlighting specific data aspects. For example, bar charts quickly show comparisons between groups, while scatter plots can reveal correlations between variables. Additionally, incorporating color and dimension into graphical representations can provide depth but should be approached with caution to maintain clarity.
Interactive Dashboards
Interactive dashboards are a powerful tool in biomedical data analysis, merging graphical representation with user engagement. They present multiple visualizations in a single interface, allowing users to explore and interact with the data. Users can filter, zoom, and drill down into details, transforming static data into insightful experiences.
The advantages of using interactive dashboards include:
- Dynamic exploration of data, enabling users to investigate different scenarios.
- Real-time updates, which is crucial for clinical settings where timely information is essential.
- Integration of multiple data sources, consolidating disparate datasets into a coherent overview.
However, designing effective interactive dashboards requires careful consideration of usability and user experience. Key factors to keep in mind include:
- Simplicity: Avoid overwhelming users with excessive options or complex layouts.
- Contextual relevance: Ensure that the interactivity serves a purpose and enhances understanding.
- Accessibility: Account for diverse user skill levels and backgrounds, ensuring that all can benefit from the insights presented.
"Effective data visualization is key to unlocking insights hidden within the data, driving impactful healthcare outcomes."
Applications of Biomedical Data Analysis
Biomedical data analysis has emerged as a cornerstone of modern healthcare, offering insights that directly influence treatment protocols, research methodologies, and overall health outcomes. This section explores three critical applications: drug discovery processes, patient care, and disease prediction and diagnostics. Each subsection highlights the specific advantages of utilizing data analysis within these domains, underscoring the synergy between data-driven decision-making and improved healthcare delivery.
Drug Discovery Processes
In the realm of drug discovery, biomedical data analysis plays a transformative role. Traditional methods of drug discovery are often time-consuming and expensive. However, the integration of data analytics accelerates this process significantly. By leveraging data from various sources, including genomic databases and clinical trial results, researchers can identify potential drug candidates much more effectively.
Data analysis not only streamlines the identification of molecular targets but also aids in predicting how compounds will behave in the body. Tools such as machine learning algorithms analyze massive datasets, delivering meaningful predictions regarding efficacy and safety.
"Biomedical data analysis has effectively reduced the time required for drug discovery, bringing new treatments to patients faster than ever before."
Moreover, unique computational methods enable the simulation of drug interactions on biological pathways, thus enhancing the overall understanding of pharmacodynamics and pharmacokinetics. These insights are crucial for ensuring that new therapies are both innovative and safe for patient use.
Patient Care and Personalized Treatment
The paradigm of patient care has evolved, with a growing emphasis on personalization. Biomedical data analysis supports this shift by providing healthcare professionals with a wealth of information tailored to individual patients. By analyzing genetic markers, lifestyle factors, and clinical history, practitioners can develop customized treatment plans that address the unique needs of each patient.
For example, in oncology, data analysis can uncover the specific mutations present in a tumor, allowing for targeted therapies that are more likely to succeed. This approach leads to improved prognosis and minimizes the trial-and-error nature of conventional treatments.
Additionally, predictive analytics can identify patients at risk for certain conditions, enabling proactive interventions that lead to better outcomes and reduced healthcare costs. Such approaches not only enhance the quality of care but also foster a deeper patient-provider relationship, built on trust and personalized attention.
Disease Prediction and Diagnostics
The capacity for accurate disease prediction through biomedical data analysis is a game changer in the clinical landscape. Analytical methodologies can sift through vast amounts of health data, including electronic health records and imaging results, to identify patterns and trends that may indicate the onset of diseases.
For instance, machine learning models are increasingly applied to predict the likelihood of conditions such as diabetes or cardiovascular diseases based on historical data. These predictions empower healthcare professionals to implement preventive measures before the disease manifests.
Furthermore, diagnostics have also benefited significantly from data analysis. Sophisticated algorithms analyze imaging studies to support early diagnosis of conditions, such as cancers, with higher precision. This not only expedites treatment initiation but also increases the likelihood of favorable outcomes.
Challenges in Biomedical Data Analysis
Biomedical data analysis, while offering transformative potential in healthcare, is fraught with intricate challenges. Addressing these challenges is essential for ensuring the reliability and validity of analysis outcomes. This section delves into three primary hurdles: data privacy concerns, data integration issues, and the quality and standardization of data. Each of these elements plays a pivotal role in shaping the landscape of biomedical data analysis.
Data Privacy Concerns
Data privacy is a pressing issue in biomedical data analysis. With sensitive information about patients often involved, there is an urgent need to protect this data from unauthorized access and breaches. Strict compliance with regulations like the Health Insurance Portability and Accountability Act (HIPAA) in the U.S. is critical. Failure to protect patient information can lead to severe legal ramifications and can erode trust between patients and healthcare providers.
Moreover, the usage of electronic health records (EHRs) has increased the volume of sensitive data available for analysis. This includes personal, medical, and financial information. Protecting patient identity while utilizing this data for research remains a complex balancing act.
"In biomedical data analysis, ensuring patient confidentiality is not just a legal requirement but a fundamental ethical obligation."
Data Integration Issues
Data integration poses significant challenges within biomedical research. Often, data exists in disparate formats and from multiple sources, including genomic databases, clinical trials, and wearable devices. Integrating these varied data types into a cohesive system is essential to derive meaningful insights. Without effective integration, critical connections between datasets may be overlooked, resulting in incomplete analyses.
Moreover, a lack of standardized protocols for data collection and storage further complicates the integration process. Researchers often face difficulties in correlating findings across different studies due to variability in data handling. Tackling these issues requires advanced data integration techniques and collaborative efforts frameworks.
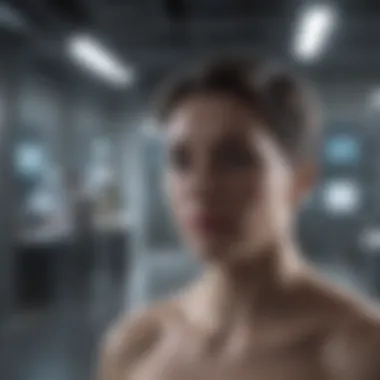
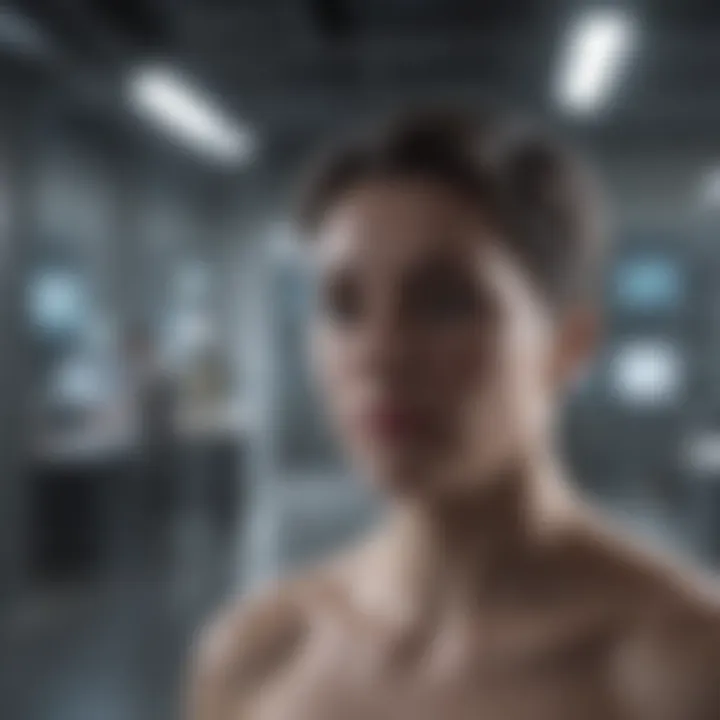
Quality and Standardization of Data
The quality and standardization of data is a crucial consideration in biomedical data analysis. High-quality data is necessary for accurate results. Yet, issues such as missing values, errors, and inconsistencies can undermine the integrity of analytical processes. When data is not standardized, comparing results between studies becomes challenging, hampering the reproducibility of scientific findings.
Implementing robust data validation protocols can help mitigate these quality issues. Standardized data formats and terminologies, such as the use of Common Data Elements (CDEs), can enhance consistency across datasets. Investing in these standardization efforts is critical for ensuring reliable outcomes in clinical research and patient care.
Ethical Considerations in Biomedical Data Analysis
The landscape of biomedical data analysis is marked by complex ethical challenges. As this field grows, the implications of data use in healthcare become critical. Understanding ethical considerations helps ensure that data analysis contributes positively to health outcomes while protecting individuals' rights. Addressing these factors is necessary for building trust and accountability in the use of biomedical data.
Informed Consent
Informed consent is a foundational principle in biomedical research. It involves ensuring that individuals understand what participation entails before agreeing to share their data. This process is not just a formality; it safeguards participants' autonomy. Researchers must communicate the purpose of data collection, how the data will be used, and potential risks involved.
Moreover, informed consent is dynamic. As projects evolve, the circumstances change. Researchers should thus revisit consent agreements, providing updates and obtaining renewed consent if significant changes occur. Failure to do so could lead to ethical violations and erode public trust. A clear and transparent informed consent process can enhance participant engagement in studies and promote ethical standards in data analytics.
Bias and Fairness in Algorithms
Bias in algorithms is a pressing issue in the realm of biomedical data analysis. Algorithms, when trained on biased datasets, can perpetuate existing inequalities. This raises serious ethical concerns about fairness in healthcare outcomes. For instance, if an algorithm primarily trained on data from a specific demographic, it may not perform well across diverse populations.
Addressing bias starts with dataset diversification. Data scientists must ensure that the data sources reflect the population's diversity. This includes gender, ethnic, and socioeconomic factors. Additionally, regular audits of algorithms are necessary to identify and correct biases. Engaging with community stakeholders can also provide insights into how these tools affect various populations. By striving for fairness, biomedical data analysis can improve the efficacy of healthcare solutions provided to all patients.
"Ensuring fairness in algorithms is not just about technical corrections; it's about social responsibility in healthcare."
In summary, ethical considerations in biomedical data analysis warrant careful attention. Informed consent and the management of bias are paramount. By prioritizing these elements, researchers and practitioners can foster a culture of integrity and respect in healthcare data utilization. Such measures ultimately enhance not only the quality of research but also the trust placed in the field by the public.
Future Trends in Biomedical Data Analysis
The field of biomedical data analysis is evolving rapidly. New technologies and methods emerge frequently, enhancing our capabilities to analyze vast amounts of health-related data. Recognizing and addressing future trends is critical for maximizing the benefits of biomedical data analysis in healthcare. Incorporating advanced techniques can lead to significant improvements in patient care, research, and overall healthcare systems. It also encourages a culture of innovation, collaboration, and continuous learning among professionals in this field.
Artificial Intelligence Integration
Artificial intelligence (AI) is playing an essential role in the evolution of biomedical data analysis. By leveraging machine learning algorithms and deep learning techniques, AI can analyze complex datasets more efficiently than traditional methods. This enables researchers to uncover patterns and insights that may have remained hidden in the past.
- Enhanced Predictive Analytics: AI can improve the accuracy of predictive models, enabling healthcare professionals to make informed decisions based on data. This has far-reaching implications for patient outcomes and treatment effectiveness.
- Automation of Data Processing: AI can automate routine tasks such as data cleaning and preprocessing. This helps analysts focus on more complex aspects of data analysis, thereby increasing productivity and accuracy.
- Personalized Medicine: AI's ability to analyze large datasets can lead to more tailored treatment plans, aligning perfectly with the goals of precision medicine. Through AI, healthcare practitioners can create individualized strategies that optimize therapeutics for each patient.
"The integration of AI in biomedical data analysis is transforming how healthcare professionals understand and treat diseases, prompting a shift toward more effective, personalized care."
Decentralized Data Management
As the demand for transparent, secure, and efficient data management rises, decentralized data management systems are gaining traction in biomedical data analysis. Such systems offer significant advantages in terms of data accessibility, privacy, and security.
- Improved Data Accessibility: Decentralized systems allow data to be stored across multiple locations. This makes it easier for various stakeholders, including researchers and healthcare providers, to access essential data while maintaining data integrity.
- Enhanced Security: By utilizing technologies such as blockchain, decentralized data management systems can provide robust security features. This protects sensitive health data from unauthorized access and malicious attacks.
- Facilitating Collaboration: Decentralization can foster collaboration among researchers and institutions. Shared access to data can accelerate discoveries and encourage innovative approaches to tackle complex health issues.
Overall, these future trends in biomedical data analysis present numerous opportunities for improving healthcare outcomes and enhancing research methodologies. By embracing these trends, the healthcare sector can advance towards a more data-driven future.
Case Studies of Biomedical Data Analysis
The examination of case studies in biomedical data analysis serves as a pivotal component in understanding the practical implications and successes of applying data-driven methodologies in real-world contexts. Case studies provide concrete examples that illustrate the benefits, challenges, and innovative solutions that arise when biomedical data is leveraged effectively. By analyzing specific instances, stakeholders in healthcare, research, and policy can glean insights that inform future practices and strategies. The relatability of these cases enhances learning, making complex ideas more accessible and underscoring the relevance of biomedical data analysis in driving improved health outcomes.
Successful Data-Driven Clinical Trials
Clinical trials represent a critical phase in the development of new therapies and medical interventions. The integration of biomedical data analysis into this process has proven invaluable. Successful data-driven clinical trials often utilize large datasets to streamline participant recruitment, optimize treatment protocols, and improve the accuracy of outcomes assessment. With the help of advanced statistical analysis and machine learning algorithms, researchers can identify suitable patient populations, predict responses to treatment, and minimize the risk of adverse effects.
For example, the use of predictive modeling in clinical trials for diabetes medications allowed researchers to select participants who would likely benefit most from the new drug. This targeted approach not only improved the efficiency of the trial but also reduced patient exposure to ineffective treatments.
Moreover, the analysis of real-time data during clinical trials can lead to adaptive trial designs. This flexibility enables modifications to the study as it progresses, ensuring that the research remains relevant and impactful. Key benefits of data-driven clinical trials include:
- Enhanced recruitment strategies through data analytics.
- Improved identification of biomarkers for patient stratification.
- Real-time monitoring of patient outcomes.
- Increased efficiency in trial execution.
Ultimately, successful data-driven clinical trials not only advance medical knowledge but also contribute to safer and more effective treatments for patients.
Innovative Applications in Cancer Research
Cancer research is at the forefront of utilizing biomedical data analysis, with numerous innovative applications enhancing our understanding and treatment of various cancers. The complexity of cancer, involving genetic, environmental, and lifestyle factors, demands sophisticated analytical approaches to uncover meaningful patterns and relationships within vast datasets.
One significant innovation is the use of genomic data analysis to personalize cancer treatment. By sequencing the genomes of tumors, researchers can identify specific mutations that drive cancer growth. This information can be used to tailor therapies that target these mutations, resulting in more effective treatment plans.
Additionally, machine learning algorithms are employed to predict cancer progression and treatment responses. For instance, neural networks can analyze imaging data and clinical records to forecast outcomes, guiding oncologists in their decision-making processes. Some practical applications of biomedical data analysis in cancer research include:
- Genomic profiling to identify treatment targets.
- Predictive analytics for recurrence and survival rates.
- Integration of multi-omics data to understand cancer biology better.
As cancer research continues to evolve, the role of biomedical data analysis will only expand, fostering innovations that have the potential to transform patient care and improve survival rates. > "Real-time data analysis is taking cancer research to new heights."
Culmination
In the context of biomedical data analysis, the conclusion summarizes the crux of the discussion presented in this article. It serves not only as a closure but also highlights the vital role that effective data analysis plays in the modern healthcare framework. This final segment allows the reader to consolidate knowledge around several key considerations that emerge throughout the exploration.
The understanding of what constitutes biomedical data analysis goes beyond merely grasping its methodologies and applications. It encompasses recognizing the implications on healthcare outcomes and the potential future directions this field may take. The discussion about ethical considerations and challenges reflects the complexity of handling sensitive health data responsibly.
Key elements such as those identified in the previous sections underscore that biomedical data analysis is a cornerstone of precision medicine, where treatments can be tailored to individual patients based on comprehensive data insights. Moreover, acknowledging the challenges discussed - like data privacy, integration, and standardization - prepares stakeholders for the hurdles they may encounter in practice.
This article brushes upon awareness of advancements in machine learning and artificial intelligence, spearheading transformational change within clinical settings. The implications of these technologies on drug discovery and patient care cannot be overstated. They are reshaping our approach to diagnostics and treatment, leading to improved patient outcomes.
Thus, the conclusion strings together these various threads of analysis, bringing forth a coherent narrative.
"The advancement of biomedical data analysis signifies where healthcare is headedโnot away from human interaction, but toward a more informed and data-driven approach to patient care."
In essence, the conclusions drawn in this article emphasize the necessity of continued innovation and vigilance in how data is analyzed, shared, and utilized. The journey into biomedical data analysis remains ongoing, with uncharted territories yet to be explored. Continued dialogue and study are crucial as researchers and practitioners strive to refine approaches and ultimately enhance human health across diverse populations.