Exploring the Foundations and Implications of Networks
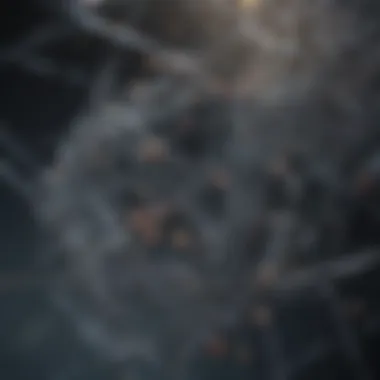
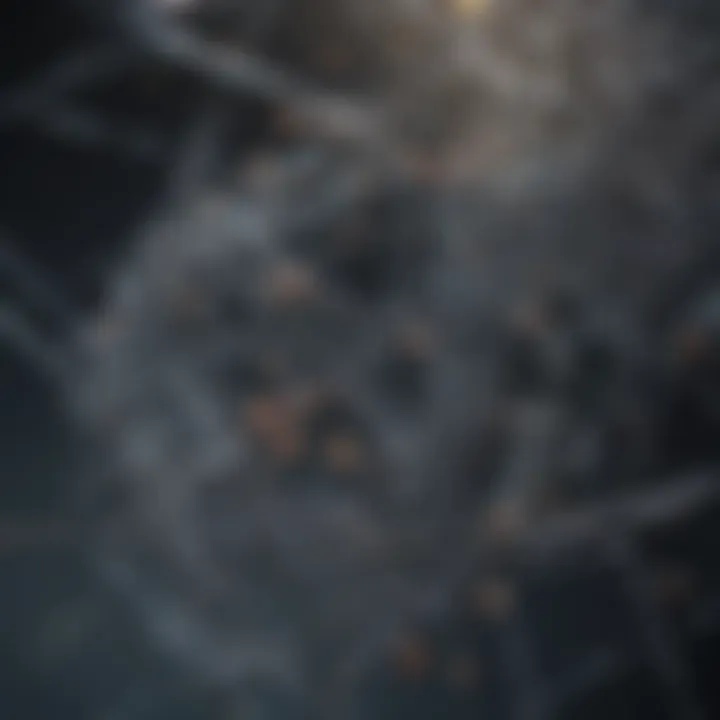
Intro
The study of networks presents a rich tapestry of structures and functions across different fields. Networks, consisting of nodes tied together by connections, exist in varied forms, from the biological networks of neurons in the brain to technological networks like the Internet, and social networks that shape human interactions. Understanding these networks is crucial, as they not only outline the framework of interactions but also pave the way for insight into complex systems. In this article, we aim to dissect the intricate dynamics of networks, exploring their types, significance, and implications.
Research Methodology
Description of research design and approach
The research strategy employed in this study incorporates a multi-disciplinary approach, aiming to integrate perspectives from biology, technology, and sociology. This allows for a comprehensive understanding of networks and their interdependencies. Observational data is gathered through both qualitative and quantitative methods, ensuring a broader scope of interpretation.
Materials and methods used in the study
The materials involved in this research include existing literature, case studies, and empirical data from various fields. Methods such as network analysis, statistical tools, and simulation algorithms are utilized to examine the interactions within networks. The aim is to enhance our understanding of not just how connections form, but also how they evolve over time.
Discussion and Interpretation
Interpretation of results in the context of existing literature
Our findings reflect a significant alignment with current literature, particularly in biological and social domains. For instance, previous studies highlight the importance of strong ties in social networks for information diffusion. The analysis suggests that similar principles apply across different network types, establishing a commonality in node behavior regardless of context.
Implications for future research or practical applications
The implications of this study extend to various fields. Enhanced understanding of networks can benefit technological advancements and promote better social systems and health outcomes. Future research could explore the robustness of networks and their reaction to changes, offering practical applications in crisis management and resource allocation.
"Understanding networks is pivotal in navigating today's interconnected world. The focus should not only be on their structures but also on the underlying dynamics that drive interaction and change."
Foreword to Networks
Networks are a fundamental aspect of the world around us. Their structures and interactions influence various fields, from biology to technology and social sciences. Understanding networks is essential for comprehending complex systems. This section provides an overview of what networks are, their historical development, and their importance in contemporary research.
Definition of Networks
A network is a collection of interconnected elements, known as nodes, which are linked by relationships called edges. These connections can represent various forms of interactions, such as communication, transportation, or biological processes. Networks vary in complexity and can be simple or highly intricate. Examples include social networks like Facebook, transportation networks like railways, and biological networks, including metabolic pathways in cells. This definition serves as a foundational concept, allowing for deeper exploration of the interplay between different network types.
Historical Context
The study of networks dates back several centuries, evolving through various disciplines. Initially, graph theory, developed by mathematicians like Leonhard Euler in the 18th century, laid the groundwork for understanding networks. The well-known Seven Bridges of Königsberg problem illustrated early network analysis. Over time, the application of networks expanded, particularly in the 20th century with the rise of computer networking and telecommunications. Scholars began to see networks as crucial structures, leading to a surge in research across fields, including sociology, biology, and computer science. By tracing the historical development, one can appreciate how the concept of networks became a cross-disciplinary phenomenon today.
Importance in Today’s Science
In modern science, the relevance of networks cannot be understated. They provide a lens to study interactions within complex systems.
- In biology, networks help in understanding disease pathways and gene interactions.
- In technology, they are vital for data transfer and network security, underpinning the internet.
- In sociology, analyzing social networks reveals patterns of behavior and community structures.
The integration of network theory into scientific inquiry enhances our ability to model real-world phenomena. It allows researchers to derive insights about relationships and dynamics that are not apparent when viewing elements in isolation. Therefore, networks serve as a potent tool for advancing knowledge and solving real-world challenges.
Types of Networks
In the examination of networks, categorizing them into specific types is essential. This classification helps in understanding how networks function within different domains, as well as the unique characteristics and implications of each. By exploring various types of networks, one can gain insights that apply across disciplines, from biology to technology to social realms. Recognizing these types allows researchers and practitioners to adopt tailored methodologies that best suit their objectives when conducting analysis or implementation.
Biological Networks
Biological networks are critical in understanding the complex interactions within living organisms. They provide a framework for studying how different biological components collaborate to sustain life processes.
Metabolic Networks
Metabolic networks relate to the biochemical reactions that occur within organisms. They illustrate how substrates convert into products through chemical processes. Metabolic networks are valuable in this article as they reveal the interdependence of various pathways and their influence on overall organismal health. A key characteristic of metabolic networks is their dynamic nature. They provide insight into how organisms adapt to changes in their environment.
However, metabolic networks also have limitations. One of the disadvantages is the complexity involved in accurately modeling these networks due to numerous interactions and feedback mechanisms.
Gene Regulatory Networks
Gene regulatory networks focus on how gene expression is controlled and regulated. These networks help us understand the intricate patterns that determine when and where genes are active. The significance of gene regulatory networks is paramount in this article because they bridge genetic information with cellular function. A key characteristic is their hierarchical nature, involving transcription factors that regulate target genes. This aspect makes them indispensable in studying genetic disorders and developmental biology.
Despite their significance, gene regulatory networks face challenges in interpretation. The variability in gene expression can complicate the analysis, leading to uncertainties in conclusions drawn from studies.
Technological Networks
Technological networks encompass the systems that facilitate communication and data exchange. This category includes computer and telecommunication networks, which are foundational to modern infrastructure.
Computer Networks
Computer networks consist of interconnected computing devices that share resources and information. They are vital for enabling digital communication and collaborative work. The emphasis on computer networks in this article arises from their role in advancing technology and information systems. A critical feature is their scalability. As organizations grow, computer networks can expand to accommodate new devices and users.
One limitation of computer networks, however, includes security vulnerabilities that can lead to data breaches and other cyber threats. Ensuring security is a continuous challenge.
Telecommunication Networks
Telecommunication networks enable the transmission of information over distances. They include a range of devices and technologies that allow voice, video, and data communication. Their relevance in this article stems from their fundamental role in connecting people globally. The key characteristic of these networks is their ability to support various communication formats like voice, text and video services, enabling seamless interactions.
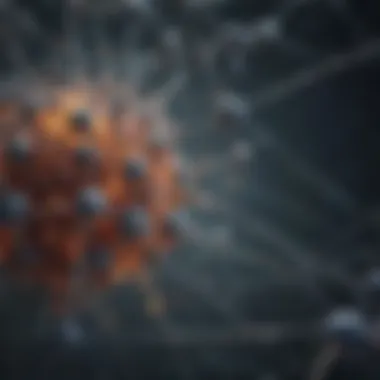
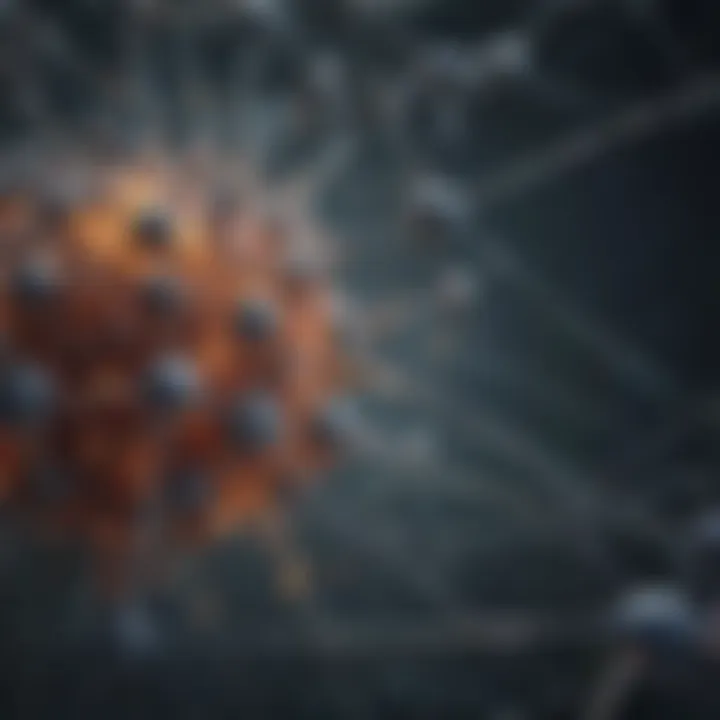
Nevertheless, telecommunication networks can face issues related to service quality and capacity. Increased traffic can degrade performance, highlighting a need for continual investment in infrastructure.
Social Networks
Social networks illustrate the relationships among individuals and groups, through both online and offline means. They encapsulate the dynamics of human interaction and information sharing.
Online Social Media
Online social media networks provide platforms where users interact, share content, and connect. They play a significant role in shaping public opinion and social behaviors. Their popularity is reflected in the article through their profound impact on modern communication. A unique feature is that they allow rapid dissemination of information, enabling users to reach wide audiences quickly.
On the downside, online social media also faces criticism for issues like misinformation and privacy concerns. The challenge of moderating content is an ongoing concern.
Professional Networks
Professional networks refer to connections that individuals foster within workforce and industry contexts. They provide an avenue for career development, knowledge sharing, and collaboration. Their inclusion in this article reinforces the idea that networks are not merely social tools but also critical for professional growth. A key characteristic is the strong ties created through shared goals and interests, promoting collaboration.
However, professional networks may also result in exclusivity, where opportunities might be limited to those within certain circles. This can hinder diversity and innovation.
Fundamental Components of Networks
Understanding the fundamental components of networks is crucial for analyzing their structures and functions. Networks consist of nodes, edges, and specific properties that define their behavior and characteristics. Each component plays a significant role in determining how a network operates, interacts and evolves.
These foundational elements help researchers identify patterns, influences, and trends within various fields, from biology to social sciences.
Nodes
A node represents an individual element in a network, whether it is a person in a social network or a protein in a biological system. The importance of nodes lies in their role as the primary points of interaction within the network. Each node can have unique attributes, such as its strength, connections, and roles.
In biological networks, nodes can represent genes or metabolites, playing critical roles in underlying processes like metabolism or gene expression. In social networks, nodes often represent users or groups, showcasing their influence and connections to others.
Edges
Edges are the connections that link nodes together, highlighting the nature of relationships between them. They can be classified into two main types: directed and undirected, and weighted and unweighted.
Directed vs. Undirected
Directed edges imply a one-way relationship, meaning that the connection flows from one node to another. This is common in scenarios like follower relationships on platforms such as Facebook, where one user follows another but not necessarily vice versa. The unique feature of directed edges allows for a clear understanding of influence and interaction.
On the other hand, undirected edges indicate a mutual relationship, where the connection flows both ways. This is typical in collaborations or friendships that exist equally between two parties. The choice between directed and undirected edges is often based on the specific requirements of the analysis being conducted. Each has its own advantages depending on the context and the questions being asked.
Weighted vs. Unweighted
When talking about weighted edges, they assign a value to the connection based on strength, frequency, or significance. This type of edge offers insights into the strength or importance of a relationship. In contrast, unweighted edges treat relationships equally, indicating a simple presence of connection without any value.
Weighted edges are particularly beneficial when analyzing nuanced relationships, such as the amount of interaction on a particular social media post, while unweighted edges can simplify models where relational strength is not as important.
Network Properties
The properties of a network provide vital insights into its structure and behavior. They are key to understanding how networks function and influence their respective environments. Two significant properties are degree distribution and clustering coefficient, which both exhibit important details about the network.
Degree Distribution
Degree distribution measures the frequency of different connectivity levels across nodes in a network. It is crucial for identifying whether a network follows certain models, such as scale-free networks, where a few nodes have many connections, and most have few. This characteristic is advantageous as it reveals underlying structures and can predict behaviors in systems, such as vulnerability to attacks.
Clustering Coefficient
The clustering coefficient quantifies the degree to which nodes in a network tend to cluster together. A higher clustering coefficient suggests that nodes are likely to form tightly knit groups. This property is significant for understanding community structures and can highlight how information spreads within networks. Analyzing clustering can provide insights into how connectedness influences certain outcomes in social systems, like group behaviors, and also in biological systems, like the co-expression of genes.
Understanding the fundamental components of networks not only helps in analyzing their structure but also provides a substantial framework for more complex studies in diverse fields, from epidemiology to technological innovation.
Theoretical Frameworks in Network Analysis
The study of networks is fundamentally grounded in specific theoretical frameworks that provide the necessary tools for understanding complex interactions. In this section, we will explore the main frameworks that guide the analysis of networks: Graph Theory, Complex Network Theory, and the study of Network Dynamics. Each of these frameworks contributes uniquely to the understanding of networks, making clear their importance in both academic research and practical applications. The frameworks help in organizing various components of networks, providing insights into structures, behaviors, and potential applications across different domains.
Graph Theory
Graph Theory serves as the backbone for many network concepts. At its core, it deals with graphs, which represent networks through nodes and edges. Nodes symbolize entities, while edges depict the relationships between those entities. One key benefit of Graph Theory is its ability to yield powerful mathematical models which simplify the representation of complex systems.
Key aspects of Graph Theory include:
- Types of graphs: This includes directed and undirected graphs, weighted and unweighted graphs. Each type has distinct properties and implications for how information flows within a network.
- Pathfinding: Algorithms based on Graph Theory, like Dijkstra’s and A* algorithms, enable the efficient navigation of networks. This is crucial in fields such as computer networking and transportation.
- Connectivity: Understanding how nodes are connected within a graph informs the robustness and resilience of networks against failures or attacks.
Graph Theory forms the foundation for more advanced network analyses and is crucial for students and researchers aiming to comprehend network structures rigorously.
Complex Network Theory
Complex Network Theory expands on the concepts introduced by Graph Theory by addressing real-world networks that exhibit non-trivial topological features. Unlike simple or regular graphs, complex networks often display patterns such as small-world phenomena, scale-free properties, and clustering.
Important considerations within Complex Network Theory include:
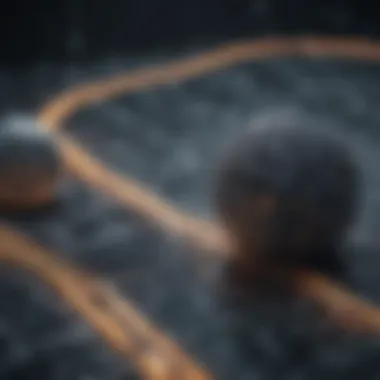
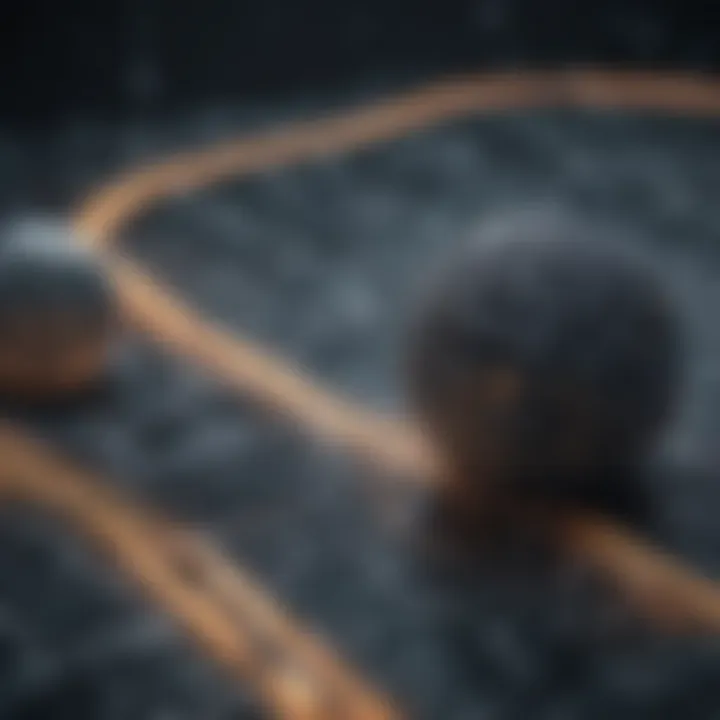
- Scale-free networks: These are networks whose degree distribution follows a power law. This means a small number of nodes have a very high degree (connections), while most nodes have relatively few connections.
- Small-world properties: This refers to a network characteristic where most nodes can be reached from every other node through a small number of steps, promoting efficient communication or flow in large networks.
- Robustness and fragility: Understanding the behavior of complex networks can highlight vulnerabilities and strengths, essential for applications in epidemiology, infrastructure, and social sciences.
Complex Network Theory is instrumental in elucidating the structural and functional complexities of networks, making it invaluable for interdisciplinary research and analysis.
Network Dynamics
Network Dynamics focuses on studying how networks evolve over time. This area is particularly important as systems often face changes due to various external and internal factors. Understanding these dynamics allows researchers to predict future behaviors or changes in networks.
Considerations surrounding Network Dynamics include:
- Evolving Networks: Many networks are not static; they grow and change in response to actions or events. For instance, social media networks expand as new users join and relationships form.
- Diffusion Processes: Understanding how information, disease, or behaviors spread within networks can provide insights into effective interventions or communication strategies.
- Stability Dynamics: Certain networks may exhibit stable or unstable configurations depending on the feedback mechanisms at play, which is critical for analyzing systems in biology or technology.
Through the lens of Network Dynamics, one gains the ability to understand both the transient and persistent behaviors of networked systems.
In summary, the theoretical frameworks outlined provide a robust foundation for analyzing and interpreting the complexities of networks. As technologies advance and the need for networked systems increases, a thorough understanding of these frameworks is vital.
Methodologies for Analyzing Networks
Analyzing networks involves systematic methodologies that are crucial for understanding the complex interactions and relationships within different types of networks. These methodologies provide insights that can significantly enhance the clarity and efficacy of network research. They help researchers make informed decisions based on data-driven analyses, leading to more reliable conclusions. In this section, we will discuss three primary methodologies used: quantitative methods, qualitative approaches, and visualization techniques. Each methodology offers unique benefits and considerations that contribute to the overall analysis of networks.
Quantitative Methods
Quantitative methods represent a fundamental approach in network analysis, emphasizing the use of numerical data and mathematical frameworks. These methods enable researchers to measure and statistically analyze various properties of networks, such as centrality, connectivity, and robustness. By applying mathematical models, researchers can identify patterns and trends that might not be evident through qualitative observations alone.
Some key benefits of quantitative methods include:
- Objectivity: Quantitative approaches reduce subjectivity by relying on numeric data, ensuring that results are reproducible and verifiable.
- Efficiency: Advanced algorithms and computational tools can process vast datasets quickly, allowing for rapid analysis even in large networks.
- Predictive Power: Quantitative methods often facilitate the development of models that predict future behaviors or changes within a network.
However, researchers must also consider potential limitations, such as:
- Data Quality: Inaccurate or incomplete data can skew results, leading to misleading conclusions.
- Over-Simplification: While numbers provide clarity, they may overlook the nuanced interactions that qualitative methods could capture.
Qualitative Approaches
Qualitative approaches in network analysis focus on understanding the context and meaning behind the relationships and interactions within a network. These methods are particularly valuable for exploring complex social dynamics or when numerical data is scarce or unavailable. By employing techniques such as interviews, case studies, and ethnographic research, researchers can gain deeper insights into how networks function.
The benefits of qualitative approaches include:
- Contextual Understanding: They provide a richer understanding of the social, cultural, and psychological factors that influence network behavior.
- Flexibility: Qualitative research can adapt to changing circumstances, allowing for new questions and insights to emerge during the study.
- Exploratory Nature: This methodology is particularly useful for generating hypotheses for future quantitative testing.
Nonetheless, qualitative methods also come with challenges:
- Subjectivity: Interpretations may vary based on the researcher’s perspective, potentially introducing bias.
- Limited Generalizability: Findings may not be easily transferrable to other contexts or populations.
Visualization Techniques
Visualization techniques are paramount in network analysis, as they convert complex data into graphical representations, making it easier for researchers to identify patterns and interact with data. They allow for an immediate appraisal of network structure and dynamics. Effective visualizations can illustrate relationships, cluster formations, and flow patterns within networks, thereby facilitating a more intuitive understanding of the data.
Some important aspects of visualization techniques include:
- Clarity: Graphical representations can summarize large datasets at a glance, highlighting critical insights that may otherwise stay hidden.
- Engagement: Good visualizations engage the audience, encouraging exploration and fostering a sense of discovery.
- Spatial Representations: They illustrate spatial relationships, which is essential in geographic or social networks.
Researchers should take care to incorporate effective visualization practices:
- Data Integrity: Accurate representations ensure that the visualized data conveys the correct information without misleading viewers.
- Tools and Software: Employing suitable software can enhance the quality and functionality of visualizations, such as Gephi or Cytoscape.
Practical Applications of Networks
The practical applications of networks play a crucial role in understanding how they influence various disciplines. By applying network concepts, researchers and professionals can address complex problems, optimize processes, and enhance decision-making. In this section, we will explore several key applications of networks across fields such as biology, technology, and social science.
Applications in Biology
Disease Spread Modeling
Disease spread modeling utilizes network theory to assess how infections travel through populations. It is fundamental for public health strategies and response planning. Understanding the network structure of a population allows for better prediction of disease propagation. A key characteristic of this approach is its ability to represent contacts among individuals, which can vary widely. Disease spread modeling is beneficial because it can inform vaccination strategies and resource allocation. However, a unique feature is its reliance on accurate data about contact rates, creating a potential disadvantage if data quality is poor.
Drug Discovery
Drug discovery, a critical aspect of molecular biology, employs networks to explore interactions between various compounds and biological targets. This approach is central in identifying potential drug candidates efficiently. The key characteristic of drug discovery is its integration of diverse biological data into a cohesive network, facilitating the identification of pathways that can be leveraged for therapy. It is popular due to its capacity to reduce the time and cost associated with traditional drug development methods. However, a challenging aspect is the complexity of biological systems, which can lead to unpredictable outcomes, necessitating caution in interpretation.
Technological Innovations
Network Security
In the realm of technology, network security is vital for protecting data from unauthorized access and cyber threats. Strong network security measures can safeguard organizational information and maintain user trust. A key characteristic is its multi-layered approach, which includes aspects such as firewalls, intrusion detection systems, and encryption. This makes network security a beneficial topic, as it is critical to the functioning of modern businesses and the privacy of users. However, one unique feature is the continuous evolution of threats and defensive mechanisms, making constant updates and vigilance necessary to ensure security.
Data Transfer Optimization
Data transfer optimization focuses on improving the efficiency of data flow across networks. It is essential for enhancing user experience and maximizing resource utilization. The key aspect of data transfer optimization lies in protocols and techniques that aim to minimize latency and maximize throughput. This makes it a central topic as organizations increasingly rely on timely data access. Unique in its technicality, this optimization often encounters challenges related to bandwidth limits and network congestion, which can complicate implementation.
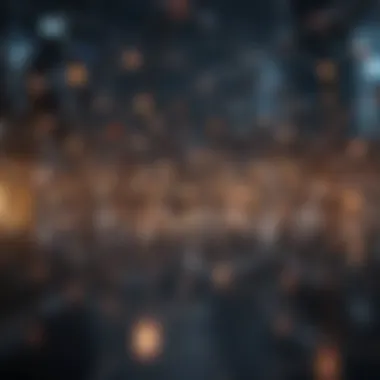
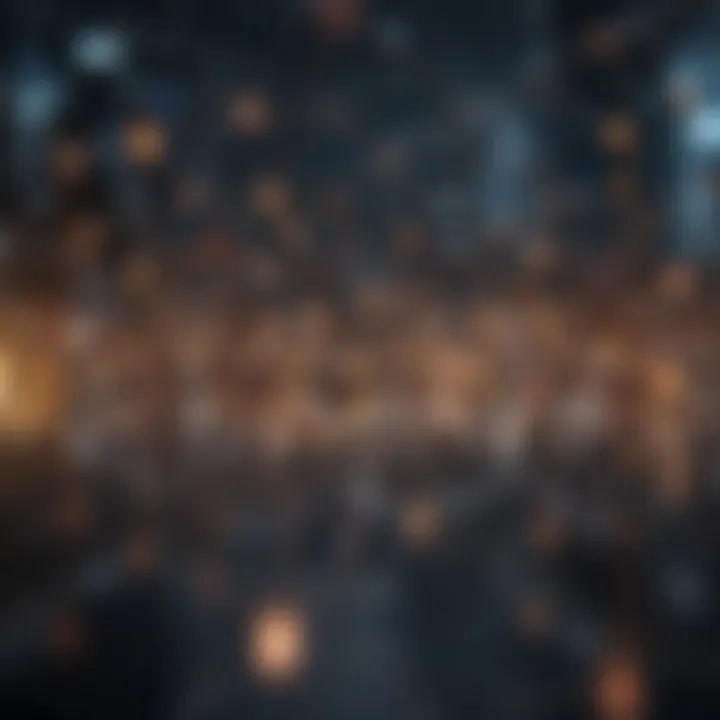
Social Insights
Behavioral Analysis
Behavioral analysis uses network theories to interpret how individuals interact within social groups. It uncovers patterns that reveal deeper insights into societal behaviors. A key characteristic of behavioral analysis is its focus on relationships and influence within groups, making it particularly insightful for understanding trends. It is a relevant choice as it helps businesses and researchers predict responses to changes in social conditions. Nonetheless, the unique feature lies in its potential limitations due to privacy concerns when analyzing personal data, necessitating ethical considerations.
Community Detection
Community detection identifies clusters within social networks, revealing how different groups interact. This approach is significant in both sociological research and marketing strategies. A key aspect of community detection is its capacity to segment the network into meaningful subgroups, facilitating targeted strategies. It is beneficial for its applicability in diverse fields, including sociology and organizational development. However, a challenge remains in defining what constitutes a community, which can affect the accuracy of detection algorithms.
Challenges in Network Analysis
The exploration of networks introduces a multitude of complexities that are crucial to understand. Network analysis, while offering powerful insights, faces a range of challenges that can hinder its effectiveness. Addressing these challenges is essential for accurate data interpretation, informed decision-making, and successful implementation of network-based solutions. This section highlights three primary challenges: data quality issues, computational complexity, and the interpretation of results.
Data Quality Issues
Data quality is foundational for any analysis. In network studies, the accuracy and reliability of the data directly impact outcomes. There are a few prominent issues that often arise:
- Incomplete Data: Missing data can lead to significant biases. Nodes or connections that aren't included in the dataset can result in an inaccurate portrayal of network dynamics.
- Inconsistent Data: Variability in how data is recorded can cause confusion. For example, different criteria for defining a connection can lead to inconsistencies.
- Outdated Information: Networks can change over time. Using outdated data may yield results that are no longer relevant.
To navigate these challenges, it is essential to establish robust data collection protocols, validate data sources, and regularly update datasets. Reliable data enables more effective network analysis.
Computational Complexity
The complexity of networks increases with the size and interconnectivity of the data. As networks grow, the computational requirements for analysis also escalate. Several factors contribute to this complexity:
- Large Datasets: Handling extensive networks with millions of nodes and edges demands significant computational resources.
- Algorithm Performance: Not all algorithms are developed to handle large and complex networks efficiently. Some algorithms may offer inaccurate results when applied to larger datasets.
- Dynamic Changes: Networks are not static; their topology can change, leading to challenges in real-time analysis.
To cope with these issues, researchers often utilize advanced computational techniques, such as distributed computing or parallel algorithms. By optimizing performance, the analysis can remain feasible even with large and complex networks.
Interpretation of Results
Interpreting results in network analysis can be challenging. The nuances in understanding the social, biological, or technological implications of network data can vary significantly. Key considerations include:
- Context Sensitivity: Network data may convey different meanings based on the context. The same structure can represent various phenomena in different fields.
- Complex Relationships: Relationships within a network can change direction and intensity over time, complicating the analysis of its results.
- Potential for Misinterpretation: Results can be misleading without proper context. Analysts must be cautious in drawing conclusions based solely on algorithmic outputs.
Establishing clear methodologies for interpretation can help mitigate these challenges. A well-defined framework will help to clarify the implications of network structures while considering their limitations.
"Recognizing and addressing the challenges in network analysis is instrumental in deriving meaningful insights that can drive innovation across multiple disciplines."
By understanding these challenges, researchers and practitioners can take proactive measures to enhance their analysis, leading to more accurate insights and applications.
Future Directions in Network Research
The landscape of network research is constantly evolving. It reflects the complexity of the systems being studied and the rapid advancements in technology. As such, identifying future directions in this field is critical for both theoretical development and practical applications. Several specific elements emerge as focal points in driving the next wave of insights in network studies.
Emerging Trends in Theory
Theory in network research must adapt to the increasing complexity of networks. One prominent trend is the focus on multilayer networks, which allow the analysis of interdependent systems across different domains. For instance, social media affects personal relationships while simultaneously influencing societal trends. Researchers are exploring how these layers interact, leading to smarter models that can predict various outcomes across complex dynamics.
Another direction is the development of dynamic network models. These models aim to capture temporal changes, providing insights into how networks evolve over time. Here, the distinction between static and dynamic views becomes crucial. The integration of real-time data into models can offer a more nuanced understanding of phenomena, from disease outbreaks to viral marketing campaigns. Engaging in these emerging theoretical frameworks can drastically improve our comprehension of networks at large.
Interdisciplinary Approaches
The future of network research lies heavily in the embrace of interdisciplinary frameworks. Collaboration across scientific domains enhances methodologies and can generate innovative perspectives. For instance, combining principles from biology, computer science, and sociology can enrich understanding and lead to advances in various fields.
Fostering interdisciplinary connections promotes the sharing of knowledge and tools. An example is the adoption of machine learning from computer science in analyzing large-scale biological networks. This cross-discipline synergy can lead to breakthroughs in areas such as public health and climate science, revealing insights that single-discipline approaches might miss.
Technological Innovations on the Horizon
Technological advancements will undeniably shape the future of network research. The growing prevalence of big data is a game-changer. Researchers can now analyze vast datasets that include diverse network types, including social networks, transportation networks, and more. This capability allows for better modeling and exploration of very complex interactions.
Moreover, artificial intelligence and machine learning will play increasingly vital roles in network analysis. AI can efficiently handle data complexity and generate predictive models, opening avenues for research that have not been possible before. For instance, AI-driven algorithms can optimize network structures for better performance in fields such as logistics and telecommunications.
In summary, approaching the future of networks through the lenses of theoretical evolution, interdisciplinary collaboration, and technological innovation promises significant advancements in understanding complex systems. Embracing these directions can facilitate more impactful exploration and application of networks across various fields.
Epilogue
In concluding this article, it is vital to emphasize the significant impact that the study of networks has on multiple disciplines. The complexities of networks stretch from the microscopic interactions seen in biological systems to the vast structures of technological and social systems. This conclusion serves as a reflection on the collective insights gathered from previous sections, consolidating the understanding and importance of networks in contemporary research.
Summary of Key Points
The article systematically uncovered several key aspects related to networks:
- Definition and Historical Context: Networks are defined and contextualized within their historical development, illustrating their evolution over time.
- Types of Networks: Various types of networks such as biological, technological, and social were explored, each revealing unique characteristics and functions.
- Components and Properties: A detailed discussion on nodes and edges outlined how these components interact to form complex systems.
- Frameworks and Methodologies: Theoretical frameworks such as graph theory were presented, alongside methodologies that facilitate network analysis.
- Applications and Challenges: The practical applications in different fields highlight the relevance of networks, while also addressing challenges that need ongoing attention.
- Future Directions: Emerging trends and interdisciplinary approaches pointed to areas ripe for further exploration.
This summary helps to crystallize the articles focus and demonstrates just how expansive the field of network analysis is, making it relevant across disciplines.
Implications for Future Research
Looking ahead, the implications for future research in network analysis are profound. The landscape continues to evolve, and several areas warrant deeper examination:
- Interdisciplinary Fusion: The blending of insights from various disciplines can lead to innovative solutions to complex problems. Collaborations between biology, computer science, and social science can yield new frameworks for understanding networks.
- Technological Advances: Continued development in computational tools will enable more sophisticated analyses of networks and their dynamics. Machine learning, for example, is poised to enhance predictive capabilities in network behavior.
- Real-World Applications: The urgency to apply network insights to real-world issues such as health, security, and social dynamics remains a pressing concern. Studies that focus on application can bridge gaps between theory and practice, advancing the societal relevance of network research.
- Ethical Considerations: As networks in social contexts expand, ethical implications around data privacy and surveillance must continue to be addressed. Understanding the ramifications of network actions on individual behavior will be crucial.
In all, future research should build on existing knowledge while embracing the potential of innovative methodologies and interdisciplinary collaboration. The implications are vast and underscore the need for ongoing inquiry within the field of network studies.