Exploring Stress Testing Models: A Comprehensive Overview
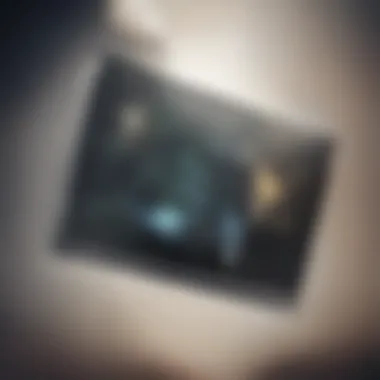
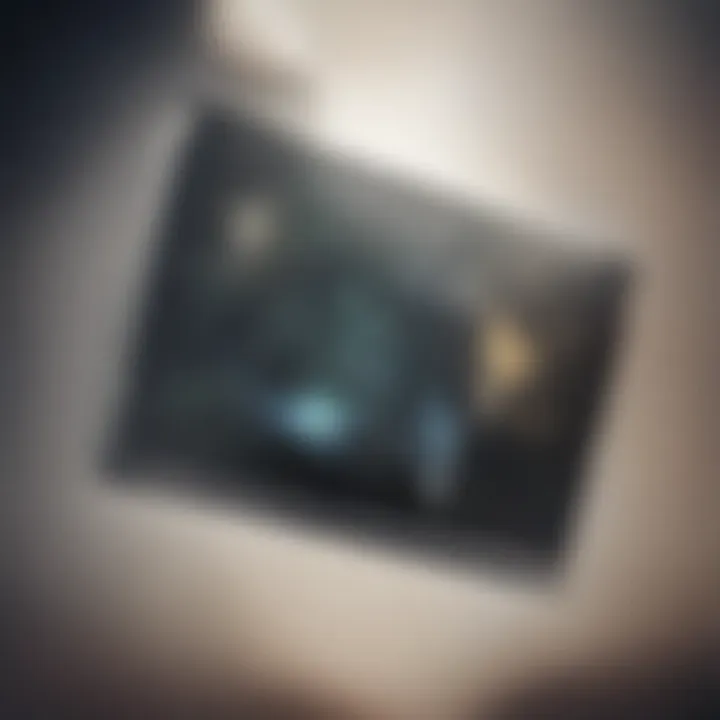
Intro
Stress testing has gained prominence across numerous scientific disciplines, serving as a crucial tool for assessing the resilience of systems under various adverse conditions. It is vital to understand how these models work and their practical implications for real-world applications. This article aims to breakdown different stress testing models, emphasizing their methodologies, significance, and challenges faced during implementation. By analyzing current trends, this comprehensive overview provides insights beneficial for researchers, educators, and professionals.
Research Methodology
Description of Research Design and Approach
The research design utilized in stress testing models primarily revolves around a systematic examination of existing literature and case studies across various fields. For instance, finance, engineering, and environmental science each utilize unique methodologies tailored to their contexts. The approach taken integrates qualitative and quantitative analysis to derive meaningful conclusions from the data.
Materials and Methods Used in the Study
For this study, a variety of materials were utilized:
- Peer-reviewed journals to ensure reliability and current understanding of models.
- Statistical software such as R or Python for data analysis and visualization, enabling effective representation of findings.
- Case studies from industry environments to provide practical insights while illustrating the models in action.
A comprehensive review was conducted focusing on recent publications and seminal texts that laid the groundwork for current practices in stress testing.
Discussion and Interpretation
Interpretation of Results in the Context of Existing Literature
The analysis reveals a growing consensus on the importance of incorporating stress testing into decision-making processes. The literature provides a wide range of perspectives regarding methodologies and their outcomes. For example, the application of stress testing in financial markets highlighted by authors like Benston and Hunter demonstrates how sensitivity analysis can predict market reactions amidst economic turbulence.
"Stress testing is not just about economic indicators; it is about understanding interdependencies and vulnerabilities within systems."
Implications for Future Research or Practical Applications
Future research should focus on improving the models’ adaptability to emerging variables such as climate change impacts or technological advancement. Additionally, practitioners are encouraged to collaborate across disciplines to establish best practices that can streamline processes and enhance predictive accuracy.
As this field evolves, it becomes increasingly essential to develop standardized methods that both researchers and practitioners can utilize, ensuring that findings are widely applicable and actionable.
Prologue to Stress Testing Models
Stress testing models have become an essential component in various fields, particularly in finance, healthcare, and engineering. They serve as a means to evaluate how systems respond under adverse conditions. This section unfolds the critical elements of stress testing, emphasizing its significance in decision-making processes.
Definition of Stress Testing
Stress testing is a simulation technique used to evaluate the resilience of a system under extreme conditions. It allows organizations to determine how factors such as economic downturns, operational failures, or unexpected events can influence performance metrics. The primary goal is to identify vulnerabilities and strengthen the system. By understanding the limits of what a system can endure, stakeholders can make better-informed decisions.
Historical Context and Evolution
Historically, the concept of stress testing originated in the financial sector where institutions needed to analyze potential risks following economic crises. The 2008 financial crisis acted as a catalyst, highlighting the necessity for rigorous stress testing practices. Over time, this practice evolved not only in finance but also in sectors like public health and environmental science. Today, advancements in technology and data analytics have further refined stress testing methodologies, making them more robust and applicable across diverse fields. The continuous evolution reflects the growing recognition of potential risks associated with dynamic environments.
Stress testing is not only about identifying weaknesses but also about developing actionable strategies for resilience.
In summary, understanding stress testing models is crucial. It aids in risk management and enhances preparedness against unpredictable events. As systems become increasingly interconnected, the relevance of these models will only grow.
The Importance of Stress Testing
Stress testing is crucial in various fields, offering insights into potential vulnerabilities. Understanding its importance can positively impact decision-making processes.
Risk Assessment and Management
In the context of financial services, stress testing plays a pivotal role in identifying and assessing risks. Financial institutions use stress tests to evaluate their ability to withstand economic disruptions. By simulating adverse conditions, such as a significant market downturn or an economic shock, organizations can quantify the potential impact on their operations and financial health. This proactive approach allows for effective risk management strategies to be developed and implemented.
Stress testing models facilitate enhanced risk awareness among stakeholders. They provide detailed analyses that highlight areas subject to significant risk exposure. For example, how will a sudden increase in interest rates affect a bank's capital ratios? Evaluating different scenarios aids in understanding vulnerabilities that might not be apparent under normal operating conditions.
Moreover, these models promote a culture of preparedness. Institutions that regularly conduct stress tests typically have better crisis management plans in place. This leads to improved resilience, allowing organizations to navigate uncertainties with more confidence.
Regulatory Compliance Implications
Compliance with regulatory requirements is another vital aspect of stress testing. Financial regulators often mandate that banks conduct regular stress tests to ensure stability within the financial system. These tests help regulators assess whether a firm has sufficient capital to face adverse economic situations. Failure to comply with such requirements can lead to severe penalties, including fines or sanctions.
For instance, the Dodd-Frank Act requires stress testing for large banking organizations in the United States. These regulations aim to mitigate systemic risks and promote transparency in financial reporting. Firms must not only prepare for stress testing but also be able to demonstrate their findings to regulators.
Furthermore, regular stress testing reinforces trust within the market. Investors and stakeholders are more likely to engage with firms that exhibit solid risk management practices. When organizations proactively demonstrate their preparedness for potential shocks, they foster an environment of confidence and security, which ultimately benefits the broader financial ecosystem.
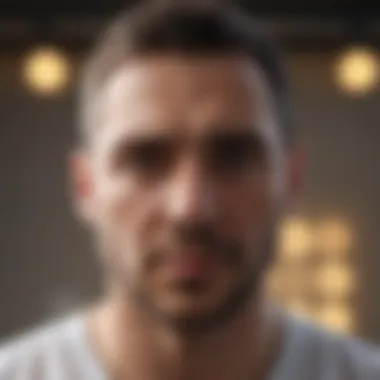
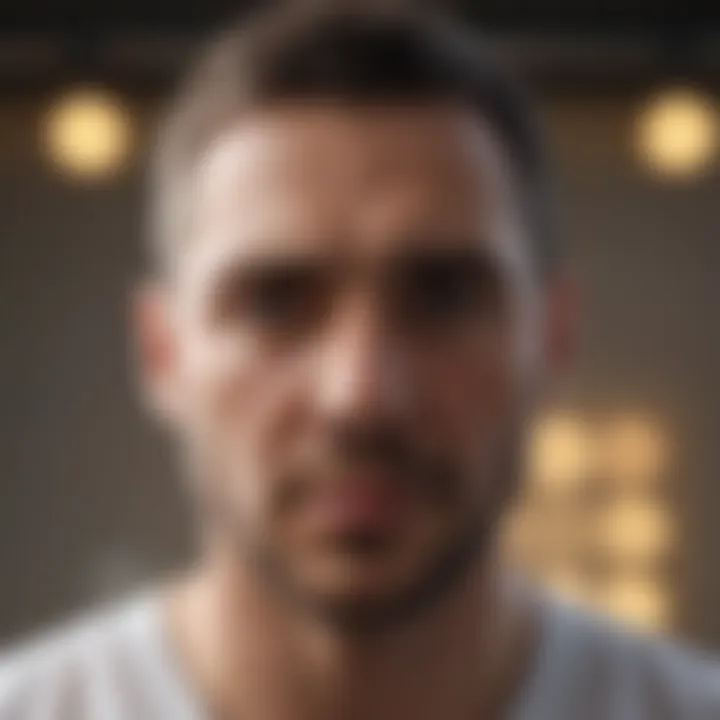
In summary, understanding the importance of stress testing models is essential for effective risk management and regulatory compliance, ensuring that organizations are prepared for economic challenges.
The incorporation of stress testing in risk assessment and compliance frameworks ensures that organizations can navigate complex market conditions effectively. Investing effort in these processes leads to better resilience and trust among stakeholders.
Types of Stress Testing Models
Understanding the different types of stress testing models is crucial for comprehensive risk management and assessment. Each model offers distinct advantages and nuances in application, making them suited for various contexts across disciplines. By carefully selecting the right type of stress testing model, researchers and professionals can identify vulnerabilities and ensure that organizations are resilient against adverse conditions. This section dives into three primary models: Scenario-Based Stress Testing, Sensitivity Analysis, and Reverse Stress Testing.
Scenario-Based Stress Testing
Scenario-Based Stress Testing is a proactive approach that simulates extreme yet plausible events. This type focuses on exploring what-if scenarios to assess how systems respond to hypothetical stresses. The selection of scenarios is critical, as they must reflect possible future states, including adverse economic shifts or environmental disasters. These simulations reveal the impact on key metrics, ensuring preparedness in uncertain times.
Benefits:
- Provides insights into potential consequences of extreme events.
- Helps in fine-tuning risk management strategies.
- Can inform regulatory compliance requirements by demonstrating due diligence.
Considerations include the need for accurate models that reflect real-world dynamics. Scenario selection must be grounded in robust data and expert opinion. Overall, Scenario-Based Stress Testing cultivates a deeper understanding of risk exposure in various scenarios.
Sensitivity Analysis
Sensitivity Analysis evaluates how sensitive a particular outcome is to changes in input variables. This method addresses the question of which variables carry the most weight in influencing results. By varying input parameters systematically, it is possible to assess their impact on outcomes and identify critical thresholds for risk.
Benefits:
- Aids in determining which factors drive variability in results.
- Supports decision-making by highlighting areas requiring closer monitoring.
- Enables optimization of resources by focusing on the most influential variables.
This type of analysis is particularly valuable in financial modeling and risk assessment where numerous variables impact the overall picture. However, it relies heavily on the integrity of the data utilized, making proper data collection techniques paramount.
Reverse Stress Testing
Reverse Stress Testing flips the traditional approach. Instead of starting with an event and assessing impacts, this method begins with a desired outcome and works backward to identify scenarios that could lead to failure. This perspective helps organizations understand potential tipping points and vulnerabilities they might not be aware of under normal stress testing scenarios.
Benefits:
- Encourages a deeper exploration of low-probability, high-impact events.
- Helps organizations to proactively design strategies to avoid identified risks.
- Shifts focus from compliance to resilience and strategic planning.
While Reverse Stress Testing can be highly insightful, careful consideration is required in scenario formulation to avoid catastrophic assumptions. It often requires collaboration across departments to integrate diverse knowledge bases and perspectives.
“Stress testing models should be dynamic, evolving alongside the changing landscape of risks.”
In summary, the different types of stress testing models each bring unique benefits and considerations. Organizations and researchers must choose appropriate models that align with their specific needs and context. This tailored approach enhances risk awareness and informs more effective management strategies.
Methodological Framework
A methodological framework is crucial in the study of stress testing models. It provides a structured approach to understanding how these models function, the various techniques employed, and the metrics used to evaluate their effectiveness. A well-defined framework ensures clarity and consistency in the application of stress testing across different sectors. This becomes particularly relevant when considering the complexity of financial, health, and environmental systems.
Data Collection Techniques
Data collection is the first step toward effective stress testing. The quality and relevance of data directly influence the outcome of any stress test. There exist several methods for collecting data, including surveys, existing data sets, and observational research. Each method carries its own advantages and disadvantages.
- Surveys can yield specific information directly from stakeholders, but they may also introduce biases if not carefully designed.
- Existing data sets can provide extensive historical data, reducing the time needed for data gathering. However, the context of old data may not align with current situations or conditions.
- Observational research offers real-time insights but requires extensive resources and time.
Selecting the right data collection technique is pivotal for ensuring that the subsequent modeling and evaluation processes are based on the most relevant and up-to-date information available.
Modeling Approaches
Modeling approaches are the heart of the stress testing process. Various techniques can be employed to simulate stress scenarios and evaluate their impact on a system.
- Deterministic models offer specific outputs for given inputs. They are straightforward but may oversimplify complex systems.
- Stochastic models, on the other hand, incorporate randomness, allowing for a distribution of possible outcomes. This is particularly useful for capturing uncertainty in financial markets.
- Agent-based modeling simulates the behavior of individual agents within a system, providing a deeper understanding of interactions and emergent properties.
Choosing the appropriate modeling approach depends on the context of the stress test and the specific aspects being analyzed. It is essential that modelers recognize the limitations of their chosen methods and remain transparent in their model interpretations.
Metrics for Evaluation
The evaluation of stress testing models hinges on the metrics used. These metrics provide the necessary parameters to gauge the effectiveness of the stress test and to validate its findings. Common metrics include:
- Value at Risk (VaR), which estimates the potential loss in value of an asset.
- Expected Shortfall (ES), providing a risk measure that accounts for extreme loss scenarios beyond the VaR threshold.
- Liquidity ratios help to assess a firm’s ability to meet its short-term obligations under stress conditions.
"Choosing the right metrics is as important as the modeling approach itself. It defines how well one understands the impacts of stress tests on the system being studied."
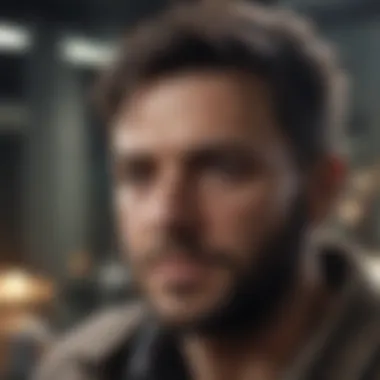
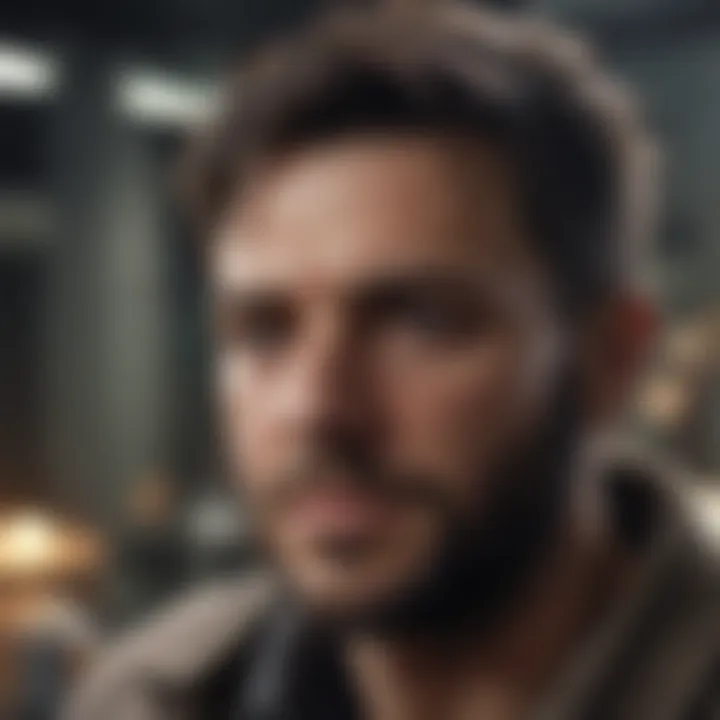
Applications of Stress Testing Models
Stress testing models are essential tools used across various sectors to evaluate the resilience and stability of systems under pressure. The broad applicability of these models underscores their importance. Understanding the specific applications helps to grasp how stress testing can provide valuable insights and inform decision-making.
Banking and Financial Services
In the banking and financial services industry, stress testing is crucial for assessing risks related to economic downturns and other financial shocks. Banks use stress tests to evaluate their financial health in adverse conditions. This practice not only aids in risk management but also ensures compliance with regulatory requirements.
One key example is the Comprehensive Capital Analysis and Review (CCAR) conducted by U.S. banks. This program mandates financial institutions to assess their capital adequacy under stressful economic scenarios. The results inform regulators about the institution's ability to withstand financial crises.
Benefits for the banking sector include:
- Enhanced Risk Awareness: Through stress tests, banks identify vulnerabilities in their portfolios.
- Improved Capital Planning: Test results inform more strategic capital allocation.
- Regulatory Compliance: Stress testing demonstrates adherence to regulatory standards.
Insurance Sector
In the insurance industry, stress testing models are potent tools for evaluating underwriting and investment risks. Insurers face varied risks from natural disasters, economic fluctuations, and changing market conditions. Thus, stress testing provides a lens to view how these risks affect their balance sheets.
"Stress testing enables insurers to anticipate claims during catastrophic events and maintain adequate reserves."
Applying stress tests allows insurers to measure the impact of extreme events on their solvency ratios. This capability is particularly vital for life insurance, where long-term obligations must be matched against current economic indicators.
Considerations for the insurance sector:
- Risk Mitigation: Identifying potential liabilities enhances risk avoidance strategies.
- Operational Resilience: Enables firms to adapt operationally to crisis conditions.
- Consumer Confidence: Provides assurance that companies can meet policyholder claims.
Public Health and Safety
Stress testing models in public health focus on evaluating the capacity of health systems to respond to crises such as pandemics or bioterrorism. By analyzing various stress scenarios—such as an outbreak or natural disaster—health officials can gauge resource availability.
This application highlights the importance of modeling potential capacity gaps in hospitals and healthcare systems. Stress tests can reveal weaknesses in supply chains critical for medicine and equipment. Furthermore, it encourages proactive planning and resource allocation.
Main aspects of public health applications include:
- Preparedness Planning: Helps in devising strategies for potential health emergencies.
- Resource Allocation: Optimizes distribution of medical supplies and personnel.
- Performance Assessment: Benchmarks current readiness against stressed conditions.
Environmental Studies
Finally, stress testing models play a significant role in environmental studies, helping researchers assess ecosystem resilience against climate change. Models can simulate the effects of increased temperatures, rising sea levels, or extreme weather events on various ecosystems.
This approach is integral for environmental policy-making. It aids in predicting the impacts of environmental stressors and shapes conservation strategies. By identifying vulnerabilities within ecosystems, stress tests inform stakeholders about necessary interventions.
Key benefits in environmental applications:
- Ecosystem Management: Provides data for sustainable resource management decisions.
- Policy Development: Informs evidence-based policies to mitigate climate impacts.
Challenges in Stress Testing Models
The topic of challenges in stress testing models is critical for comprehending the efficacy and reliability of these frameworks. Stress testing serves as a fundamental component in various sectors such as banking, insurance, and environmental science. Despite its relevance, practitioners consistently encounter a plethora of challenges that can undermine the effectiveness of stress tests. Understanding these challenges offers insights into better practices and more robust models.
Data Limitations
Data quality stands as a main pillar in the effectiveness of any stress testing model. Insufficient or poor-quality data can lead to skewed results and, ultimately, flawed conclusions. Often, historical data may not reflect future conditions due to substantial changes in market dynamics, regulatory frameworks, or underlying economic assumptions. Here are several factors associated with data limitations that practitioners must consider:
- Data Inaccessibility: Many organizations struggle to obtain complete datasets that capture all relevant variables needed for accurate stress testing. This is prevalent in sectors like public health, where dataset completeness is crucial.
- Outdated Information: Relying on historical data raised during a vastly different economic environment can lead to misleading predictions.
- Granularity Issues: Some stress tests require high-resolution data; however, organizations may only have aggregated or averaged data that does not provide the necessary detail required for precise modeling.
Model Complexity and Interpretation
Stress testing models often exhibit considerable complexity. This complexity can arise from the intricate interactions between numerous variables and assumptions made within the models. Successful interpretation of these models is not always straightforward, which raises several challenges:
- Model Overfitting: This occurs when the model is too tailored to historical data, failing to generalize well to future scenarios. It compromises the predictive accuracy of stress tests.
- Interpretational Challenges: Stakeholders may struggle to comprehend model outputs, especially if they lack the technical background. This can lead to a disconnect between model results and strategic decision-making.
- Continuous Calibration Requirement: Models need frequent updating to remain relevant in fast-changing environments. Without constant calibration, their reliability may diminish quickly.
Dynamic Market Conditions
The ever-evolving nature of markets poses significant challenges for stress testing models. External factors such as geopolitical risks, natural disasters, and technological advancements can dramatically alter the landscape:
- Rapid Market Changes: Market conditions may shift abruptly. Models designed under stable conditions may fail to predict outcomes accurately during turbulent times.
- Regulatory Changes: New regulations can impose different requirements on models, compelling organizations to redesign or modify existing frameworks.
- Unpredictable External Shocks: Factors like financial crises or pandemics can introduce hysteresis in market behavior, making previous data less applicable for future stress tests.
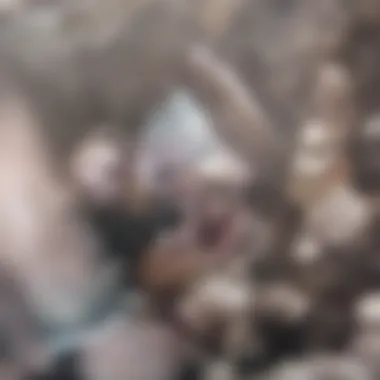
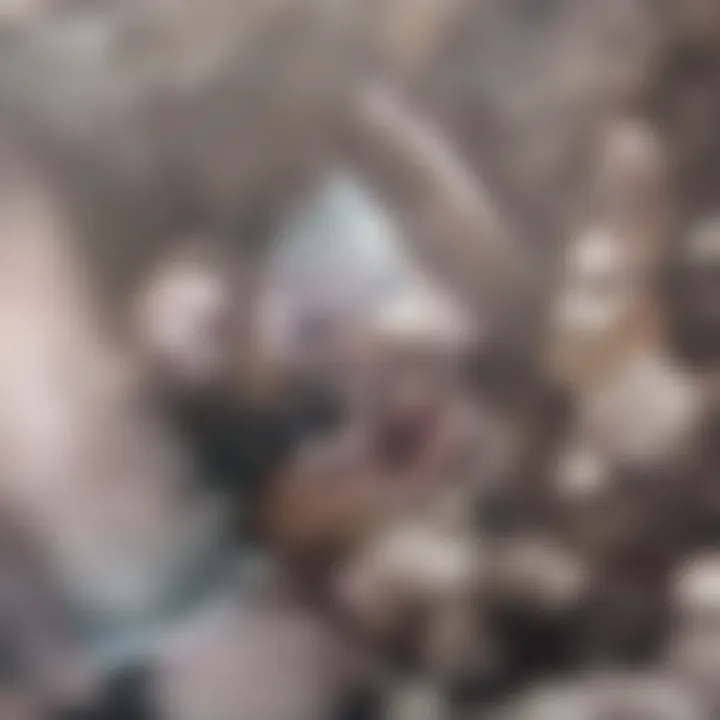
In summary, the challenges posed by data limitations, model complexity, and dynamic market conditions underscore the necessity for ongoing diligence in the development and implementation of stress testing models. Practitioners must remain vigilant to adapt their methods accordingly to ensure reliable and relevant assessments.
Comparative Analysis of Stress Testing Frameworks
The comparative analysis of stress testing frameworks plays a crucial role in enhancing the understanding of various approaches adopted across different sectors. By dissecting the different methodologies and standards, this section aims to provide insights into the effectiveness and limitations of these models. The importance of such an analysis lies in its ability to identify commonalities and differences, ultimately leading to the development of best practices that can be universally applied. This contributes significantly to the reliability and robustness of stress testing processes.
Global Standards and Best Practices
Understanding the global standards and best practices is essential for organizations looking to implement effective stress testing frameworks. These standards, often set by regulatory bodies, provide a foundation that helps ensure consistency and credibility. For example, frameworks established by institutions like the Basel Committee on Banking Supervision guide financial organizations in maintaining adequate capital reserves.
In addition to these regulatory frameworks, best practices evolve from real-world applications. These practices focus on:
- Clear documentation of methodologies used.
- Ongoing validation of models to ensure their effectiveness against changing conditions.
- Regular updates to incorporate new data and insights.
*
"Establishing benchmarks for stress testing enhances transparency and comparability across industries."
*
Global standards also drive innovation in stress testing. As organizations adopt these practices, they often tailor them to meet their unique requirements, leading to advancements that can benefit the wider industry.
Case Studies of Different Industries
When reviewing case studies across various industries, it becomes evident that stress testing frameworks can differ significantly based on context. In finance, for example, stress tests may manipulate economic scenarios to evaluate a bank's resilience to systemic shocks. Meanwhile, in public health, frameworks might focus on assessing the healthcare system's capacity to manage sudden increases in patient load during a crisis.
Some illustrative examples include:
- Banking Sector: After the 2008 financial crisis, banks enhanced their stress testing practices, focusing on liquidity and capital reserves under hypothetical stress scenarios.
- Insurance Industry: Insurers apply stress testing to assess the impact of catastrophic events on their portfolios, determining potential payouts and reserve adequacy.
- Public Health: Stress testing during pandemics evaluates healthcare systems' readiness to handle surges in patients, informing resource allocation and planning.
Each of these examples underscores the necessity for tailored approaches in stress testing, with an emphasis on lessons learned documented through detailed case studies. By examining diverse applications, stakeholders can gain a broader understanding of the operational significance of stress testing and refine their approaches according to industry-specific needs.
Future Directions in Stress Testing
Future directions in stress testing are critical as they pave the way for enhanced risk assessment methods across various sectors. As complexities in financial systems, environmental challenges, and healthcare risks grow, adapting stress testing models to meet new demands becomes essential. This section explores the integration of technology, machine learning, and advanced simulation techniques, all of which can redefine stress testing methodologies.
Impact of Technology and Automation
The role of technology and automation cannot be understated in modern stress testing. Enhanced computational power and sophisticated software allow for quicker and more detailed analysis of potential risks. The adoption of automation tools enables institutions to conduct stress tests more frequently and efficiently. This not only saves time but also improves the accuracy of the results. Automated systems can incorporate real-time data, allowing organizations to adjust their models dynamically in response to changing market conditions.
Moreover, integrating technology within testing frameworks can help to uncover scenarios that were previously hard to model or unnoticed altogether. This increased capacity to explore extreme scenarios will ensure that organizations are better prepared for unforeseen events.
Integration of Machine Learning
Machine learning presents a transformative opportunity in stress testing. By utilizing algorithms that can learn from vast datasets, financial and risk managers can develop more sophisticated stress testing models. These models can identify patterns and correlations that traditional statistical analyses may overlook.
Furthermore, machine learning models can adapt and improve over time, leading to ongoing refinement of stress testing capabilities. For instance, by applying supervised learning techniques, institutions can refine their estimates based on historical outcomes after stress events. This evolution will support more robust risk management strategies moving forward.
Advancements in Simulation Techniques
Advancements in simulation techniques also signal the future direction of stress testing. Tools that leverage Monte Carlo simulations, for instance, enhance the variety and range of scenarios tested. These advanced simulations can model complex interactions between risk factors and financial instruments, leading to a deeper understanding of potential vulnerabilities.
In addition to Monte Carlo methods, agent-based modeling provides an innovative approach to visualize the behavior of individual entities within a system. By manipulating parameters and observing outcomes, organizations can evaluate resilience under various stresses.
The implementation of such cutting-edge techniques ensures that stress testing remains relevant and effective in addressing contemporary challenges. As risks evolve, so too must the methods used to assess them, making ongoing research in this area crucial.
"The future of stress testing lies in its ability to adapt and evolve with technology, providing organizations with resilience against ever-changing risks."
Ending
The conclusion serves as a vital endpoint of this article, encapsulating the overarching themes and insights discussed. It is in this section where the reader can synthesize the wealth of information presented, reinforcing the importance of stress testing models in various fields. By summarizing the key findings, the conclusion solidifies the reader's understanding and appreciation of the complexities surrounding these models.
Summary of Key Findings
Stress testing models have emerged as indispensable tools across multiple sectors. They offer a structured approach to evaluate the resilience of systems under adverse conditions. Key findings include:
- Risk Management: The ability to foresee potential failures enhances strategic planning.
- Regulatory Compliance: Meeting the demands set by entities such as the Basel Committee is essential for financial institutions.
- Diverse Applications: From banking to public health, the relevance of stress testing spans various critical domains.
By integrating these insights, organizations can better navigate uncertainties and bolster their resilience.
Final Thoughts on Stress Testing Models
Stress testing models are not static; they evolve continually with advancements in technology and methodology. As organizations search for robust solutions to mitigate risks, embracing these models is increasingly significant. The trajectory suggests a shift towards greater automation and integration of machine learning, enhancing predictive capabilities.
It is crucial for stakeholders to remain proactive in refining their stress testing approaches. Collaboration between industries and academia can foster innovation in model development, ultimately leading to improved systemic stability.