Comprehensive Guide to Single-Cell RNA Sequencing
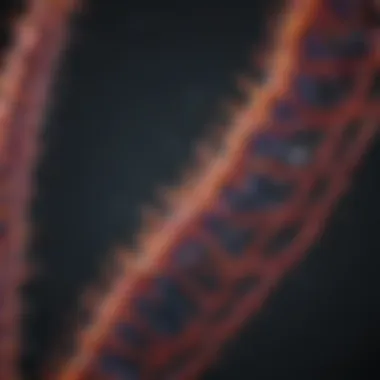
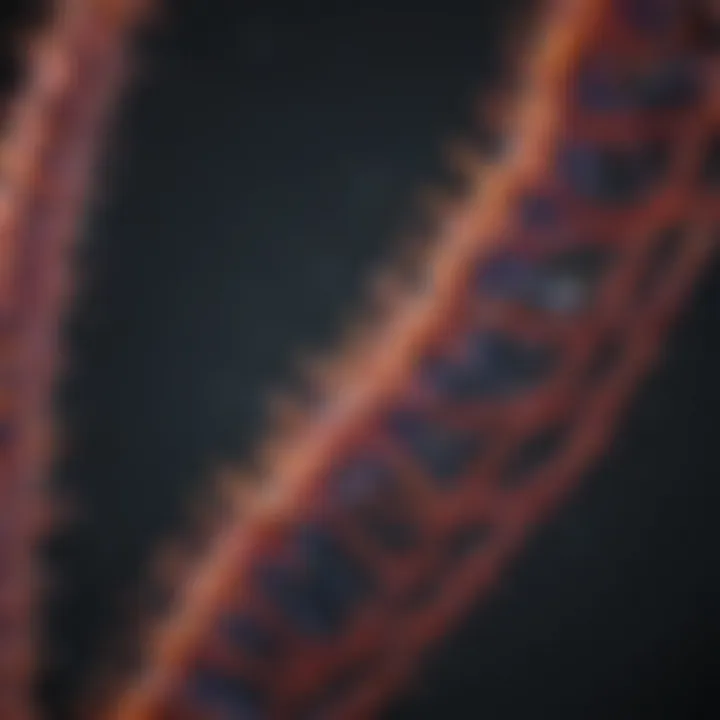
Intro
Single-cell RNA sequencing (scRNA-seq) has emerged as a game-changer in the realm of molecular biology, enabling researchers to delve deep into the complexities of cellular behavior at an unprecedented resolution. Gone are the days when scientists had to settle for averaging the gene expression profiles of entire tissue samples, which often masked the unique characteristics of individual cells. In this nuanced landscape, scRNA-seq offers a pivotal tool that not only enhances our understanding of cellular diversity but also sheds light on the functional states of cells in various contexts, whether in development, disease, or response to treatment.
This article serves as a comprehensive guide, meticulously outlining the protocols and methodologies that underpin scRNA-seq. From sample preparation to data analysis, we will navigate through the critical steps that researchers must undertake to ensure high-quality data. Furthermore, we’ll shine a spotlight on the advancements in technologies that are redefining our approach to single-cell analysis and the various factors that can influence data integrity.
In a landscape often flooded with methods and emerging technologies, clarity is paramount. As we venture deeper into the particulars of scRNA-seq, we will highlight not only the crucial steps in the protocols previously laid out but also discuss their implications for future research and practical applications. By the end of this exploration, readers will have a well-rounded understanding of how to effectively implement scRNA-seq in their investigations, underscoring its significance in shaping the future of biological research.
"Single-cell analysis may very well lead us toward personalized medicine, wherein treatment can be tailored based on a patient's unique cellular makeup."
Let’s commence our detailed journey by examining the intricacies of the Research Methodology.
Prelims to Single-Cell RNA Sequencing
Single-cell RNA sequencing (scRNA-seq) has opened a new frontier in understanding the complexities of biological systems. In contrast to bulk RNA-seq, which averages gene expression across a cluster of cells, scRNA-seq allows researchers to investigate gene expression at the individual cell level. This granularity enables the dissection of cellular heterogeneity, revealing insights into developmental processes, disease mechanisms, and more.
Definition and Importance
So, what exactly is single-cell RNA sequencing? At its core, it refers to techniques that provide a snapshot of the RNA content of individual cells, enabling a detailed pathway to unveil cellular functions and states. This approach is pivotal in modern biology, as traditional methods often mask the diversity present within seemingly homogenous cell populations. With the ability to identify rare cell types and transitional states, scRNA-seq has become an invaluable tool in many fields, including immunology, cancer research, and neuroscience.
The importance of this technology can not be overstated. By capturing the idiosyncrasies of individual cells, scientists can discern how these differences contribute to various biological responses and conditions. It lays the groundwork for personalized medicine, where treatments can be tailored based on the unique cellular landscape of a patient’s disease.
Historical Context
The roots of single-cell RNA sequencing can be traced back to the early 2000s, a time when genomics was quickly evolving. The advent of microarray technologies was the first step towards understanding gene expression at a higher resolution. However, the data produced were still a compromise, failing to portray the dynamic nature of individual cells.
The real turning point came with the introduction of next-generation sequencing (NGS) technologies around 2006. This shift paved the way for single-cell technologies to flourish, culminating in the first scRNA-seq publications in 2009. Researchers began to comprehensively profile the transcriptomes of individual cells, dwarfing previous capabilities.
Since then, the field has ballooned, driven by advancements in microfluidics, computational biology, and our understanding of cell biology itself. Today, scRNA-seq is no longer a novelty but a staple for many laboratories, allowing scientists to scrutinize cellular behavior in both healthy and diseased states. It represents a leap into a more nuanced understanding of life at a cellular level, illuminating the biological pathways that were previously shadowed in ambiguity.
"Life’s intricacies often lie in the details—scRNA-seq gives us the lens to see those details like never before."
In summary, the journey of single-cell RNA sequencing underscores not just a technological revolution but a paradigm shift in biological research. By exploring this vast landscape, we uncover the narratives of cells that compose the fabric of life itself.
Overview of Approaches to scRNA-seq
Single-cell RNA sequencing (scRNA-seq) has completely reshaped our understanding of cellular biology by allowing researchers to examine the transcriptomic landscape at the level of individual cells. The importance of recognizing the diverse approaches to scRNA-seq lies in optimizing experimental design based on specific research goals, thus ensuring reliable data generation and interpretation. Different methodologies, each with its own set of advantages and challenges, facilitate varying aspects of single-cell analysis, making it essential for researchers to choose wisely among them.
Microfluidics-Based Techniques
Microfluidics technology involves manipulating small volumes of fluids, often in the range of microliters or nanoliters, to capture and analyze single cells. This method has gained traction due to its capability to conduct multiple experiments simultaneously, allowing for high throughput while minimizing reagent costs. One notable system is the Fluidigm C1 platform. It captures single cells via a series of channels etched into a microchip, achieving high spatial resolution while keeping the environment controlled. The precision with which these techniques operate is a game changer; it enables researchers to perform intricate single-cell analyses that would be hard to accomplish with traditional methods.
Moreover, microfluidics allows for real-time monitoring of cellular responses to various stimuli, presenting opportunities for time-sensitive studies in areas like pharmacology or developmental biology. Yet, challenges remain, such as potential biases introduced during the cell capture process, which can skew results if not appropriately addressed.
Droplet-Based Protocols
Droplet-based protocols, particularly the widely recognized 10x Genomics Chromium system, rely on generating tiny droplets that encapsulate individual cells with a bead containing barcoded oligonucleotides. This method allows simultaneous processing of thousands of cells, which enormously amplifies data collection capacity. One of the remarkable aspects of this approach is its efficiency in using reagents, as multiple samples can be processed concurrently.
There are significant advantages to these protocols, including the ability to ascertain the cellular heterogeneity within a sample, providing insights into how different cells respond to treatments or lifestyle changes. However, this approach requires careful optimization to ensure that droplet formation is consistent, as inconsistencies may lead to data variability. Managing the delicate balance between cell capture efficiency and microenvironment stability can often prove to be a thorny issue for researchers.
Plate-Based Methods
In contrast, plate-based methods, such as Smart-seq2, offer more control over individual cell handling and processing. This approach usually involves manually isolating single cells into a multi-well plate before conducting the sequencing procedures. A notable strength of plate-based methods is the robust quality of data generated; they often allow for full-length transcript sequencing, giving a detailed view of gene expression levels. This is particularly useful for applications where understanding the full transcriptome is critical, such as in novel gene discovery or detailed developmental studies.
However, these methods are typically lower throughput than droplet or microfluidics techniques, and they can be quite labor-intensive, creating trade-offs in terms of scalability. That's often a consideration when planning large-scale studies where numerous samples must be processed swiftly. Nonetheless, the ability to gather comprehensive data from a well-defined group of cells makes these methods invaluable in specific contexts.
In summary, the different approaches to scRNA-seq — microfluidics-based techniques, droplet-based protocols, and plate-based methods — collectively enhance our understanding of cellular diversity and functionality. Each technique has its unique benefits and limitations, making it crucial for researchers to assess their objectives and choose the method that aligns best with their scientific inquiries.
Exploring the nuances of these methodologies can unlock new perspectives in single-cell biology, propelling forward both basic and applied research fields.
Step-by-Step Protocol for scRNA-seq
The step-by-step protocol for single-cell RNA sequencing (scRNA-seq) holds critical importance in the successful execution of this cutting-edge technology. By outlining clear procedures, researchers can produce high-quality data while ensuring consistency and reproducibility. The nuances in sample handling and processing can markedly influence the outcome, hence a methodical approach proves beneficial. With a detailed understanding of each phase—from sample preparation to data analysis—scientists can leverage the full potential of scRNA-seq in their research.
Sample Preparation
Effective sample preparation serves as the cornerstone of any scRNA-seq study. Preparing the sample correctly not only preserves the integrity of the RNA but also maximizes the quantity of usable material. The variability in cellular conditions necessitates a robust method of isolating cells followed by thorough viability assessments to ensure accurate representation of the cellular transcriptome.
Cell Isolation Techniques
Cell isolation techniques play a pivotal role in determining the fate of scRNA-seq experiments. Techniques like fluorescence-activated cell sorting (FACS) and microdissection are commonly employed, contributing to high-resolution analysis at the single-cell level. FACS stands out due to its ability to sort viable cells based on specific marker expression, allowing researchers to target particular cell types effectively. Moreover, microdissection permits the extraction of individual cells from tissues with precision, presenting a reliable option for histologically rich samples.
However, these techniques are not without their trade-offs. For instance, while FACS provides versatility, it can introduce substantial shear stress, potentially compromising cell viability. Conversely, microdissection is meticulous but can be labor-intensive and time-consuming, making it less favorable for large-scale studies.
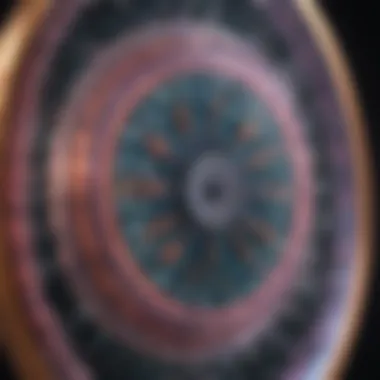
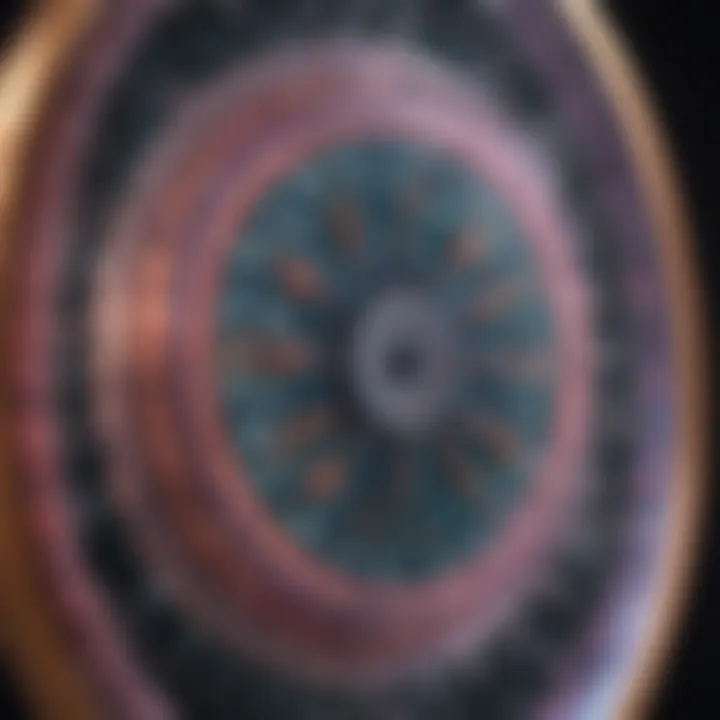
Cell Viability Assessment
Before proceeding to library preparation, it is crucial to assess cell viability. This assessment ensures that only high-quality cells, which can yield interpretable RNA data, enter the subsequent stages of analysis. Common methods include trypan blue exclusion and flow cytometry, which allow for a quick estimation of live versus dead cells.
A key advantage of cell viability testing is its immediate feedback about sample quality, guiding decisions on whether to adjust isolation procedures or repeat the experiment. However, simplified viability measures may overlook subtle cellular stresses that could affect RNA integrity, so caution is needed when interpreting results based solely on viability.
Library Preparation
Library preparation is a crucial phase where isolated RNA is converted into a form suitable for sequencing. This phase encapsulates RNA extraction and cDNA synthesis, laying the foundation for high-quality sequencing data.
RNA Extraction Methods
The method employed for RNA extraction substantially influences the yield and quality of RNA. Techniques such as TRIzol extraction and silica-column-based methods are particularly favored. TRIzol, for instance, utilizes phenol and guanidine in a single-step extraction and is often noted for its efficiency and compatibility with various downstream applications. This method captures RNA along with preserved mRNA while often achieving high purity levels.
On the other hand, silica-column methods offer quick processing times and reduced hands-on effort. However, they may sometimes lead to lower yields, necessitating careful volume adjustments during the experiment to ensure a successful library preparation. The choice of method should align with the overall goals of the research while also satisfying quality control measures.
cDNA Synthesis
cDNA synthesis represents the transformative step where the extracted RNA gets converted into complementary DNA, which is more stable and better suited for sequencing. Enzymatic reverse transcription is the standard approach, employing reverse transcriptases to synthesize cDNA from mRNA templates.
A notable characteristic of this process is the choice of primers, which can be of different types, such as oligo(dT), random hexamers, or gene-specific primers. Oligo(dT) primers allow for the capture of full-length transcripts but are biased toward polyadenylated RNA, which means some important non-coding RNA might be overlooked. In contrast, random hexamers provide a broader representation of the RNA pool, capturing a diversity of transcripts.
Nevertheless, cDNA synthesis can introduce variability, a consideration that needs careful management. Balancing between primer types and ensuring optimal conditions during synthesis can mitigate some of these concerns and ultimately improve the quality of the resultant libraries.
Sequencing Strategies
The actual sequencing of cDNA libraries is the thrilling phase of scRNA-seq, where biological questions start to receive answers. However, the choice of sequencing strategies and platforms profoundly impacts the feasibility and data quality.
Choice of Sequencing Platforms
Selecting the appropriate sequencing platform is paramount and can significantly dictate the depth and breadth of the data obtained. Platforms such as Illumina HiSeq and 10X Genomics each present unique benefits. Illumina’s systems offer high throughput at lower costs, making them popular choices for large-scale transcriptomic studies. Meanwhile, 10X Genomics provides sophisticated single-cell capture methods that yield comprehensive, cellular resolution data at relative speed.
Both platforms, while powerful, emphasize the need for careful design of experiments based on research goals, as well as budget constraints. Each researcher should weigh the pros and cons to align their sequencing technology with their specific needs.
Read Depth Considerations
Read depth plays a central role in determining the granularity of findings derived from scRNA-seq experiments. High read depths generally ensure that rare transcripts are detected, providing a detailed snapshot of the transcriptomic landscape. However, deeper sequencing is not simply about capturing more data; it introduces complexities regarding data management and analysis.
While deeper read depths can enhance sensitivity, the increased cost associated with such methodologies may limit feasibility. In many practical contexts, a sweet spot exists where sufficient data quality is achieved without excessive resource expenditure. Careful consideration of these trade-offs helps ensure that experiments yield useful, actionable insights into cellular behavior and characteristics.
Data Quality and Considerations
In the realm of single-cell RNA sequencing (scRNA-seq), ensuring data quality is paramount. The reliability of any conclusions drawn from scRNA-seq studies hinges on meticulous attention to the quality of the generated data. Poor data integrity can obscure biological insights and lead to misguided interpretations. Various factors can influence data quality, making it critical to understand and address each element in the sequencing process.
Factors Affecting Data Integrity
Technical Variability
Technical variability refers to inconsistencies that arise during the experimental procedures. These can stem from myriad sources, like differences in reagent batches, instrument calibration, or even operator handling. The contributions of this variability to the overall coherence of data can be quite pronounced.
A key characteristic of technical variability is its ability to introduce disparity within sample processing. It is a significant consideration as it showcases the potential for bias in results. In this article, it’s important to explore this aspect because it can lead to false positives or negatives, which significantly skews the interpretation of biological phenomena.
The unique feature of technical variability lies in its measurability; it can often be statistically observed and documented. However, this comes with its own set of challenges. On one hand, understanding technical variability allows researchers to refine their protocols. On the other, it presents a drawback as managing such variability requires additional resources and careful control measures.
Biological Variability
Biological variability encompasses the natural differences observed within biological systems. This can be genetic diversity between cells, cell cycle stages, or even the microenvironment affecting cellular behavior. It profoundly contributes to the nuances of single-cell sequencing, allowing for a richer understanding of cellular heterogeneity.
One key characteristic of biological variability is that it reflects the complexity of living systems. Its relevance to this article stems from the fact that it represents real biological phenomena as opposed to artifacts. This allows scientists to draw genuine insights into how cells function under different conditions.
Biological variability also holds a unique feature in that it cannot be eliminated; it's inherent to the life sciences. Its recognition is two-fold: while it provides essential data points for understanding biological processes, it also leads to challenges in data interpretation. The existence of biological variability demands sophisticated analytical methods to discern genuine biological signals from noise.
Metrics for Quality Control
To ensure robust data output from scRNA-seq experiments, quantifying metrics for quality control becomes paramount. These metrics serve as benchmarks for understanding the reliability and validity of the generated data.
Mapping Quality
Mapping quality pertains to the accuracy with which sequenced reads align to a reference genome. It acts as an essential measure of how well the sequencing process is capturing the true transcriptional landscape of the sampled cells. A high mapping quality indicates that a significant proportion of reads can be reliably traced back to the genome, which is crucial for downstream analyses.
This metric is popular in the context of scRNA-seq for its quantitative insight into sequencing performance. The unique feature of mapping quality is its ability to highlight potential biases early in the analytical workflow, guiding researchers on whether adjustments in sequencing strategy might be required. However, it also poses its challenges; mapping algorithms may introduce discrepancies based on their complexity and parameters, leading to some inconsistencies if not calibrated properly.
Gene Detection Rates
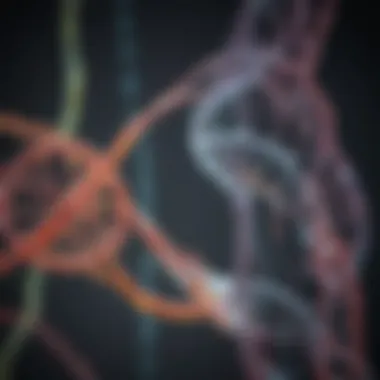
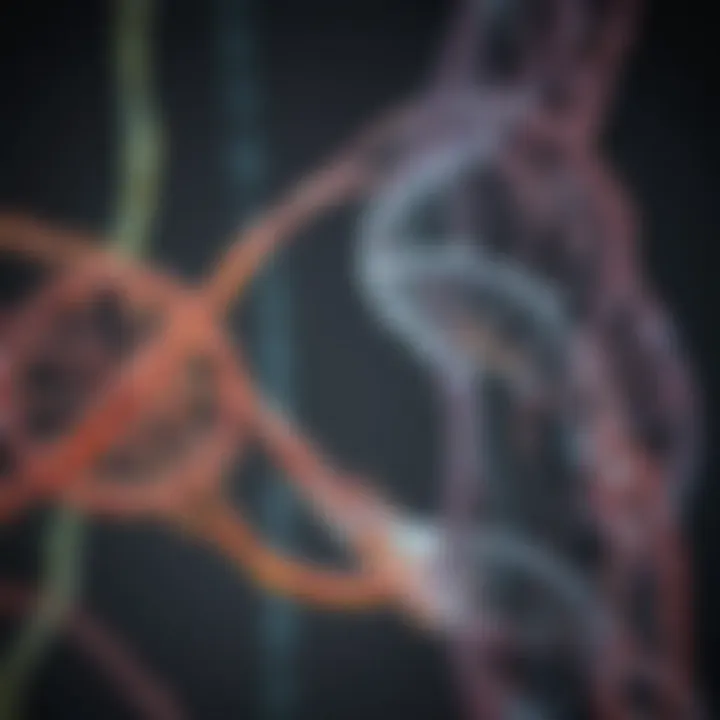
Gene detection rates represent the ability of a sequencing protocol to identify and quantify the expression levels of genes. This metric is fundamental, as it underpins our understanding of cellular function by indicating how many genes are actively being transcribed in a given sample. Higher gene detection rates correlate with richer datasets that offer greater insight into the cellular landscape.
In terms of significance, gene detection rates provide invaluable knowledge critical for interpreting cell states and transitions. The unique aspect of gene detection rates lies in their direct correlation to the biological quality of the sample; if rates are low, it raises red flags regarding the integrity of the data collected. Conversely, while high detection rates indicate thorough sampling, they may sometimes mask rare cellular events if the analysis isn’t approached with care.
"Understanding both technical and biological variability, along with implementing rigorous quality control metrics - such as mapping quality and gene detection rates - is essential for the integrity of single-cell RNA sequencing data."
Data Analysis in scRNA-seq
Data analysis in single-cell RNA sequencing (scRNA-seq) forms the backbone of understanding the complexities of cellular traits and behaviors. This crucial step translates raw sequencing data into meaningful biological insights. The effectiveness of scRNA-seq in shedding light on cellular heterogeneity heavily relies on the robustness of its data analysis techniques. Researchers have to navigate through numerous methods and tools that can either make or break their quest for understanding cellular functions and identities.
Preprocessing of Data
Preprocessing hints at the initial stage in data analysis, laying the groundwork for subsequent analyses. It involves cleaning and preparing data for a deeper dive. This stage is paramount, as the accuracy and reliability of findings directly hinge on the quality of the preprocessed data, making it a step of significance.
Normalization Techniques
Normalization techniques come into play by adjusting the data to account for variations not related to biological differences. These methods help to level the playing field, ensuring that true biological signals are discernible amid unwanted noise. One of the key characteristics of normalization techniques is their ability to standardize gene expression levels across different cells. This uniformity aids researchers in making valid comparisons between cells. Popular normalization methods like CPM, TPM, and RPKM reign supreme for their intuitive approach, making them beneficial choices for many scRNA-seq studies.
However, normalization isn't without its challenges. The unique feature of these techniques often lies in their assumptions about the data distribution, which can sometimes lead to misinterpretations if the underlying assumptions don't hold true. Hence, researchers need to carefully assess their choice of normalization method to avoid unwarranted biases in subsequent analysis.
Batch Effect Correction
In the scRNA-seq landscape, the presence of batch effects can introduce variability that is more attributed to experimental conditions rather than biological differences. Batch effect correction methods work to rectify this by diminishing the non-biological variation, allowing for a clearer focus on genuine biological signals. A key characteristic of these methods is their proactive approach in identifying and adjusting for these unwanted variations. Popular techniques such as Combat or MNN (Mutual Nearest Neighbors) become essential tools, making them favored for many studies.
Yet, these techniques come with their own set of trade-offs. The unique feature of batch effect correction is that while it strives to enhance data quality, it can also sometimes mask genuine biological variability. Hence, while these methods are invaluable, a balanced approach is critical to maintain biological relevance in the findings.
Clustering and Dimensionality Reduction
Clustering and dimensionality reduction dive deeper into the processed data, enabling researchers to categorize similar cells and uncover underlying relationships. This step is crucial as it directs attention to cell types or states that may have previously gone unnoticed.
Methods of Clustering
The methods of clustering enable the identification of distinct cell populations within heterogeneous single-cell data. Algorithms like Seurat, K-means, and Hierarchical clustering serve diverse purposes, from unmasking hidden subpopulations to elucidating developmental trajectories. One key characteristic that makes clustering methods appealing is their capacity to reduce complexity, simplifying vast datasets into more manageable and interpretable forms.
For instance, hierarchical clustering provides a visual representation through dendrograms, which aids in understanding relationships between cells. However, the unique aspect of clustering lies in its subjective nature – the choice of method can significantly influence interpretation. Thus, researchers should tread carefully, ensuring to validate their findings through complementary methods.
Visualization Techniques
Moving towards visualization techniques, this segment serves to enhance interpretability by translating complex data into understandable graphical representations. Visualization methods like UMAP (Uniform Manifold Approximation and Projection) and t-SNE (t-distributed Stochastic Neighbor Embedding) are popular choices due to their ability to effectively demonstrate cellular relationships in lower dimensions. The key characteristic that makes these tools particularly beneficial is their adeptness at preserving local structures in data while reducing dimensionality.
Their unique feature is that they not only showcase clustering but can also reveal subtle variations across cell types. However, one must remain wary; visualizations can sometimes mislead if not interpreted in context. Therefore, coupling visualization with robust statistical analysis is prime for deriving accurate conclusions from the data.
Differential Expression Analysis
Differential expression analysis stands as a cornerstone in scRNA-seq, permitting the identification of genes whose expression varies between different conditions or cell populations. This step empowers researchers to ascertain which genes may play pivotal roles in development or disease. Through the application of various statistical tests, scientists can distill the intricacies of cellular activity and highlight critical biological pathways involved in certain states or responses. The insights gained from this analytical process serve as a lens, helping to illuminate the underlying mechanisms governing cellular behavior in diverse contexts.
Applications of scRNA-seq
Single-cell RNA sequencing, commonly referred to as scRNA-seq, has ushered in a new era in the field of molecular biology, which can't be overstated. Its capability to dissect the intricate tapestry of gene expression at the single-cell level offers potent insights that were previously unattainable through bulk RNA sequencing methods. This precision is not merely beneficial; it is pivotal for advancing our understanding of complex biological systems.
The relevance of scRNA-seq spans various domains, each illuminated by its unique applications. Researchers harness this technology to:
- Understand cellular heterogeneity within tissues.
- Explore developmental processes at a granular level.
- Investigate the cellular responses to diseases such as cancer and neurodegenerative disorders.
Through the lenses of developmental biology and disease research, scRNA-seq demonstrates its potential to unveil the mechanisms underlying both normal and pathological states.
Research in Developmental Biology
In developmental biology, scRNA-seq serves as a telescope into the otherwise obscured world of cellular differentiation and lineage tracing. Researchers track the growth and specialization of cells through various developmental stages. For instance, understanding how embryonic stem cells differentiate into various cell types with unique roles lays the groundwork for regenerative medicine and therapeutic strategies.
A prime example that stands out is the ability to chart the transcriptional landscape as cells transition from pluripotent states to a more differentiated lineage. This helps answer questions such as:
- What genes are crucial at specific stages of development?
- How do environmental factors impact cellular fate?
The precision of scRNA-seq makes it an invaluable tool for developmental biologists aiming to decipher the complex gene regulatory networks guiding development.
Implications in Disease Research
The utility of scRNA-seq extends notably into disease research. Conducting single-cell analyses provides fine-grained insights into the pathogenesis of diseases, allowing for a more profound understanding of cellular responses and interactions in diseased states.
Cancer Studies
Cancer studies benefit significantly from scRNA-seq. One specific aspect is the ability to profile tumor microenvironments. By assessing the transcriptomic profiles of individual cancer cells, researchers can identify heterogeneity within tumors, highlighting differences in gene expression that correlate with treatment outcomes.
A key characteristic of cancer studies utilizing scRNA-seq is the focus on the tumor microenvironment, which includes immune cells, stroma, and malignant cells. This insight offers a comprehensive view of how these components interact, leading to treatment resistance or tumor progression. The versatile nature of scRNA-seq allows researchers to appreciate not just the cancer cells themselves, but the intricate network sustaining them, making it a popular choice for cutting-edge cancer research.
- Advantages:
- Disadvantages:
- Reveals heterogeneity within tumors.
- Identifies potential therapeutic targets based on gene expression.
- Requires meticulous interpretation from high-dimensional data.
- Increased complexity in data analysis may pose challenges for researchers.
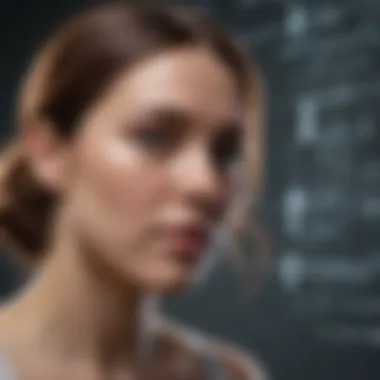
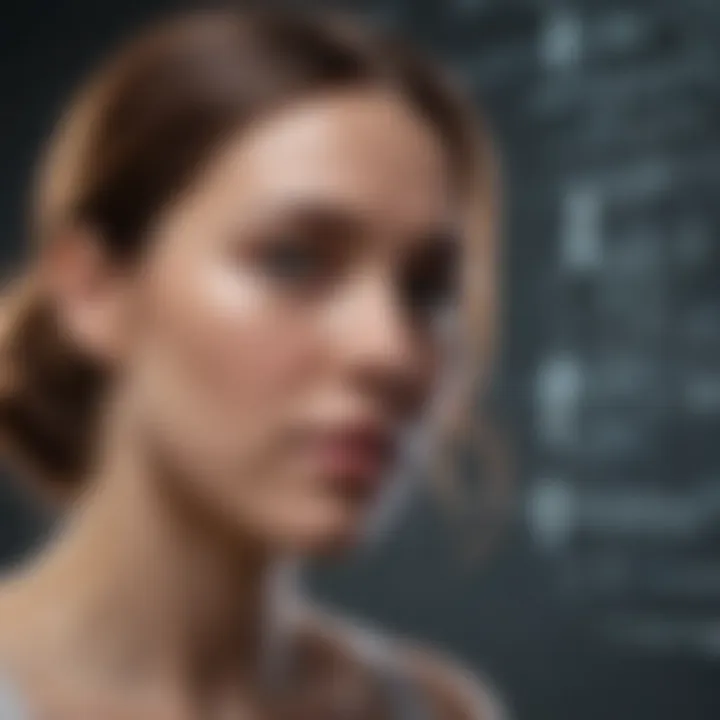
Neurodegenerative Diseases
When it comes to neurodegenerative diseases, scRNA-seq provides a lens through which the cellular components of the brain can be scrutinized. By examining neurons, glial cells, and other brain cell types, scientists can unravel the pathways deteriorating in diseases like Alzheimer’s and Parkinson’s.
A notable aspect of neurodegenerative studies using scRNA-seq is its capacity to uncover cell-type-specific vulnerabilities to stressors or toxic compounds. By profiling the gene expression of single cells, researchers gain insights into the molecular signatures associated with disease progression. These insights can contribute to identifying novel biomarker candidates for early diagnostics or therapeutic interventions.
- Advantages:
- Disadvantages:
- Offers insights into cell-type specific responses to neurodegeneration.
- Potential to identify therapeutic targets for intervention.
- The progressive nature of these diseases can complicate data interpretation.
- Requires longitudinal studies for a complete understanding of disease progression.
Both cancer and neurodegenerative disease research demonstrate the transformative potential of scRNA-seq in modern biology.
As scRNA-seq continues to bridge the gap between cellular dynamics and clinical implications, its applications across developmental biology and disease research carve pathways toward novel insights and therapeutic strategies. Its importance can not be understated, as it paves the way for a comprehensive understanding of health, disease, and development.
Challenges and Limitations
The field of single-cell RNA sequencing (scRNA-seq) is as dynamic as it is complex, leading to various challenges that can affect outcomes. Understanding these challenges is crucial for researchers who wish to apply scRNA-seq technology effectively in their studies. The importance of recognizing technical and interpretative limitations cannot be overstated, as these factors can significantly influence the quality and insights from collected data.
Technical Challenges
Technical challenges often present themselves early on in the scRNA-seq workflow. One of the most pressing issues is cell heterogeneity. When dissecting tissues, researchers might isolate cells that belong to different cell types, introducing variability in the RNA expression profiles. This diversity makes it tricky to interpret the resulting data meaningfully, as the profiles might reflect a mixture of several cell populations rather than a singular type.
Moreover, sample preparation plays a pivotal role in the reliability of results. Poorly isolated cells can lead to low RNA yields and subsequently biases in the dataset. Techniques like the Smart-Seq or 10X Genomics can help, but the choice of method will impact the quality of sequencing, leading to trade-offs between sensitivity and throughput.
Another aspect to consider is dropout events, which occur when a non-negligible proportion of RNA transcripts are not captured during the sequencing process. Dropouts can skew the perceived expression levels of genes, giving a false impression of the biological narrative being traced back to the cells.
Interpretation of Results
Once data is acquired, interpreting the results can be an uphill task. Researchers face the hurdle of establishing biologically relevant connections from large datasets, which often include noise that leads to misleading conclusions. One of the toughest nut to crack is defining differential expression. The various statistical methods employed may yield different conclusions regarding what constitutes significant expression differences, which can often compound confusion.
A reliable interpretation hinges on context: understanding the biological relevance of the detected changes requires both computational savvy and a solid grounding in biology. Without this, results may lead to hypotheses that lack a strong basis in reality.
Additionally, the integration of data from different experiments or platforms presents hurdles in standardization. Researchers must navigate the interplay of varying protocols, treatment conditions, or sequencing depths, complicating any overarching conclusions drawn from disparate datasets.
In summary, both technical challenges and the complexities of interpretation can act as roadblocks in the pursuit of insights through scRNA-seq. Internally, researchers must adopt rigorous protocols, while also being mindful of how they interpret the voluminous data obtained. Understanding these limitations can position researchers to better harness the technology for future applications, ensuring they tread carefully in drawing biological significance from their findings.
Future Perspectives in scRNA-seq Technology
The landscape of single-cell RNA sequencing (scRNA-seq) is quickly evolving, and understanding its future directions is crucial for researchers looking to stay ahead of the curve. As the complexity of biological systems unveils itself, the demand for more refined techniques grows ever louder. With advancements on the horizon, scRNA-seq is set to enhance its role in both fundamental research and clinical applications.
Advancements in Sequencing Technologies
The next generation of sequencing technologies is poised to redefine our capabilities in single-cell analysis. Historically, scRNA-seq was limited by issues like low throughput and high costs. However, innovations such as spatial transcriptomics and long-read sequencing are addressing these hurdles. Emerging platforms are now able to generate high-quality data at a fraction of the time and expense previously required. Here’s a closer look at some notable advancements:
- Higher Sensitivity and Specificity: Newer methods are designed to improve the capture of low-abundance transcripts, making it easier to detect subtle biological variations that might have been missed.
- Parallel Processing: Innovations in technology now allow for high-throughput processing of numerous samples, enabling researchers to conduct large-scale studies efficiently.
- Reduced Sample Input Requirements: Improvements in protocols mean that even limited amounts of biological material can yield reliable results, broadening the scope of what can be studied, particularly in clinical settings.
These advancements not only enhance data quality but can also enable real-time analysis in dynamic environments, giving researchers the tools to adopt a more experimental approach to biological inquiry.
Integration with Other Omics Data
One of the most exciting prospects for the future of scRNA-seq is its integration with other omics technologies, such as proteomics and metabolomics. The merging of these platforms enables a more holistic view of cellular states and functions. Combining transcriptomic data with other layers of biological information offers several advantages:
- Enhanced Biological Insights: By analyzing RNA alongside proteins and metabolites, scientists can develop a more complete picture of metabolic pathways and cellular responses.
- Identification of Biomarkers: Integrative approaches may reveal new biomarkers for diseases, facilitating early detection and therapy customization.
- Systems Biology Applications: Merging various types of data feeds into systems biology models, which can simulate complex biological processes and predict outcomes based on multiple influencing factors.
Integrating scRNA-seq with other omics is not without its challenges, though. Data standardization, sharing, and computational needs are complex issues that researchers must grapple with to make the most of these integrated approaches.
"The future of scRNA-seq will depend significantly on how well disparate fields of omics collaborate and standardize methods for data generation and analysis."
As we look ahead, it is clear that the combination of cutting-edge sequencing technologies and the integration of multiple omic layers is set to propel molecular biology into an exciting new era, enhancing our understanding of biological complexity.”
End
The significance of concluding an article on single-cell RNA sequencing (scRNA-seq) cannot be overstated. This section serves to synthesize the extensive discussions provided throughout the piece, emphasizing how critical this nascent technology is in both research and clinical applications. Given the ever-growing complexity of biological systems, the ability to analyze single cells opens doors to new avenues of understanding that were previously unimaginable.
Summary of Key Points
In recap, several pivotal points emerged throughout our exploration of scRNA-seq:
- Technical Methods: We delved into the various approaches utilized in scRNA-seq, noting how microfluidics, droplet-based protocols, and plate-based methods each have unique benefits and trade-offs.
- Protocol Steps: From cell isolation to library preparation and sequencing strategies, we outlined each step's precise nature, detailing the methods that ensure optimal data quality.
- Data Analysis: Emphasizing the importance of preprocessing, clustering, and differential expression analysis, we highlighted how embracing these analyses can significantly enrich the data interpretation.
- Real-World Applications: The discussion illuminated scRNA-seq's role in developmental biology, cancer research, and its implications for understanding neurodegenerative diseases, showcasing its widespread relevance.
- Future Directions: Lastly, advancements in sequencing technology and integration with other omics data suggest boundless possibilities moving forward.
Final Thoughts on scRNA-seq
As we close this discussion, it’s crucial to appreciate how scRNA-seq transforms our understanding of complex biological landscapes. This technique is not merely a tool; it's a revolutionary approach to genomics, enabling researchers to drill down to the intricacies of cellular behaviors. The practicality of scRNA-seq, paired with its profound implications for unexpected findings, positions it at the forefront of molecular biology.
"The future of biological research will increasingly depend on our ability to dissect the complexity of individual cells."
Moving ahead, the challenge remains to improve accessibility and reduce costs as technologies continue evolving. These considerations, tied with ethical frameworks surrounding data usage, will shape the discourse in future research.
In summary, the depths of single-cell RNA sequencing represent an extraordinary evolution in biological research, offering insights into the cellular makeup that can lead to significant discoveries and therapeutic advances. Embracing this technology might pave the way not just for significant academic discoveries, but also for tangible improvements in human health and understanding of life itself.