Diabetes Datasets: Insights for Research and Practice
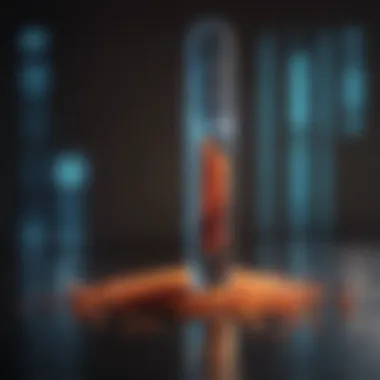
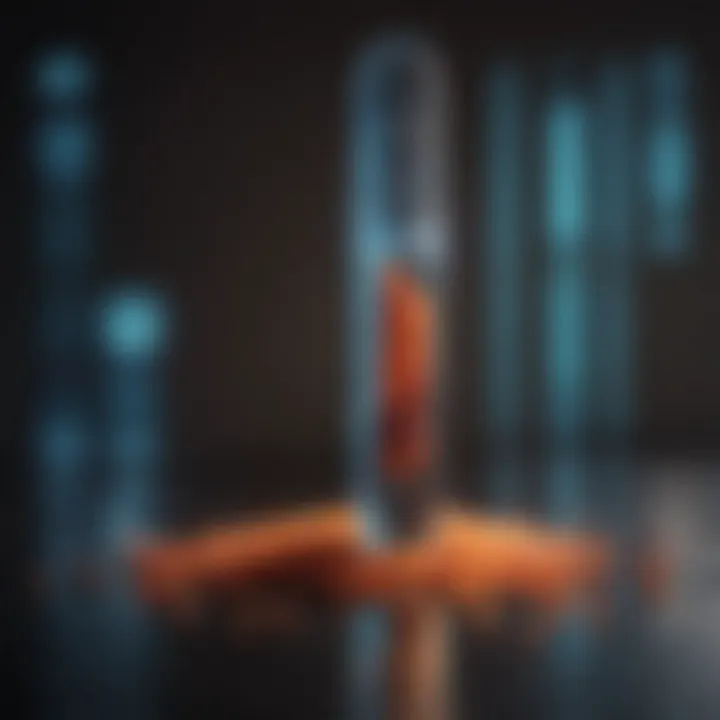
Intro
Diabetes is a chronic health condition affecting millions worldwide. Its complexities extend beyond biological processes, delving into how these aspects are analyzed and understood through research. Datasets play a pivotal role in deciphering the patterns associated with diabetes, evaluating treatment efficacy, and improving patient outcomes. By dissecting different diabetes datasets, researchers can uncover insights that inform practices and policies. This article intends to provide an expansive perspective on the significance of diabetes datasets in the medical landscape, highlighting both their technical merits and the ethical considerations attached to them.
Research Methodology
Description of Research Design and Approach
The study on diabetes datasets employs a mixed-method research design, utilizing both quantitative and qualitative approaches. The quantitative element involves analyzing numerical data derived from various sources, including clinical trials, health registries, and electronic health records. These datasets often contain demographic, clinical, and treatment-related variables that are crucial for understanding diabetes trends.
In parallel, the qualitative approach focuses on interviews and focus groups involving healthcare professionals. This method provides insights into how these datasets are interpreted in clinical settings.
Materials and Methods Used in the Study
- Data Sources
- Data Analysis Techniques
- Ethical Considerations
- The National Health and Nutrition Examination Survey (NHANES)
- Diabetes Control and Complications Trial (DCCT)
- U.S. Centers for Disease Control and Prevention (CDC) diabetes statistics
- Statistical analysis using software like R or Python
- Regression analysis to establish relationships between variables
- Machine learning algorithms for predictive modeling
- Patient confidentiality maintained throughout data handling
- Institutional Review Board (IRB) approval for data use
Discussion and Interpretation
Interpretation of Results in the Context of Existing Literature
Findings derived from the datasets emphasize trends such as the rising prevalence of type 2 diabetes among younger populations. This trend correlates with findings stated in previous studies, suggesting an urgent need for targeted prevention strategies. By comparing data across various demographic groups, researchers can pinpoint disparities in treatment access and health outcomes.
Implications for Future Research or Practical Applications
The insights gained from diabetes datasets underscore several future research avenues. One significant implication includes enhancing personalized medicine approaches, where treatments are tailored based on demographic and clinical data. Furthermore, an ongoing evaluation of new technologies such as continuous glucose monitoring can be integrated into these datasets for improved patient management.
"Effective utilization of diabetes datasets can lead to groundbreaking advancements in how we understand and manage this chronic condition, impacting millions of lives."
Preamble to Diabetes Datasets
Diabetes is a global health issue affecting millions of individuals. To understand and address its complexities, diabetes datasets play a pivotal role in gathering and analyzing critical information. These datasets encompass a variety of data types, including patient demographics, clinical measurements, and lifestyle factors. Their relevance cannot be overstated, as they provide valuable insights that inform clinical practices and public health strategies.
The introduction of diabetes datasets sets the stage for a more in-depth discussion of their importance, types, and challenges in research and practice. This section will define what diabetes datasets are and highlight their significance in advancing medical research.
Definition of Diabetes Datasets
Diabetes datasets refer to structured collections of data specifically about diabetes. They may include information on blood glucose levels, insulin usage, patient demographics, medical history, and treatment outcomes among others. These datasets can be gathered from various sources such as clinical trials, hospitals, and epidemiological studies. The comprehensive nature is essential for researchers aiming to analyze trends, treatment effects, and disease progression.
Importance in Medical Research
In the field of medical research, diabetes datasets are invaluable. They enable researchers to identify patterns and correlations in diabetes prevalence and management. Understanding these aspects is critical for several reasons:
- Treatment Efficacy: By analyzing data, healthcare providers can evaluate the effectiveness of different treatments. This leads to more personalized care for patients.
- Public Health Strategies: Aggregated data helps public health officials develop programs aimed at diabetes prevention and management.
- Policy Development: Analyzing comprehensive datasets can inform policymakers about necessary health interventions to tackle diabetes-related issues.
"Understanding diabetes datasets is fundamental to improving health outcomes for individuals affected by diabetes. They are instrumental in shaping evidence-based practices and guidelines."
In short, the importance of diabetes datasets in medical research cannot be underestimated. They not only contribute to our understanding of diabetes but also have a direct impact on patient care and public health initiatives. As we progress through this article, we will explore various types of diabetes datasets and their critical variables, highlighting their multifaceted contributions to medical science.
Types of Diabetes Datasets
Understanding the different types of diabetes datasets is crucial for several reasons. First, the diversity of data types allows researchers to explore diabetes from various angles. This multifaceted approach can lead to more comprehensive insights into the disease. Second, each dataset type often serves a specific purpose in research or clinical practice, making it important for investigators to choose the right type for their particular study. Lastly, a nuanced understanding of these datasets can enhance the accuracy of data interpretation and the effectiveness of treatment strategies.
Epidemiological Data
Epidemiological data plays a vital role in understanding the overall prevalence and incidence of diabetes across different populations. This data includes information about the demographic characteristics of individuals affected by diabetes, including age, sex, ethnicity, and geographic location.
These datasets can help identify trends over time and highlight at-risk populations. They can also inform public health policies aimed at prevention and management. For instance, access to robust epidemiological data can enable health organizations to allocate resources effectively in areas with higher diabetes rates.
Clinical Trial Data
Clinical trial data is essential in evaluating new treatments or interventions for diabetes. This type of data usually arises from controlled studies that compare effects of different medications, lifestyle changes, or procedures. It typically includes the outcomes of interest, ranging from blood glucose levels to diabetes complications.
Analyzing clinical trial data helps researchers draw conclusions about the efficacy and safety of different treatments. Moreover, such data can guide healthcare professionals in making evidence-based decisions for their patients.
Patient Registries
Patient registries are databases designed to collect and analyze data about individuals diagnosed with diabetes over a significant period. This data often includes clinical assessments, patient-reported outcomes, and long-term management strategies.
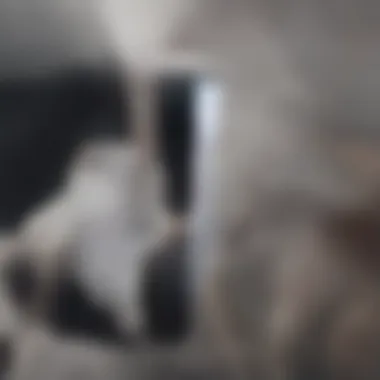
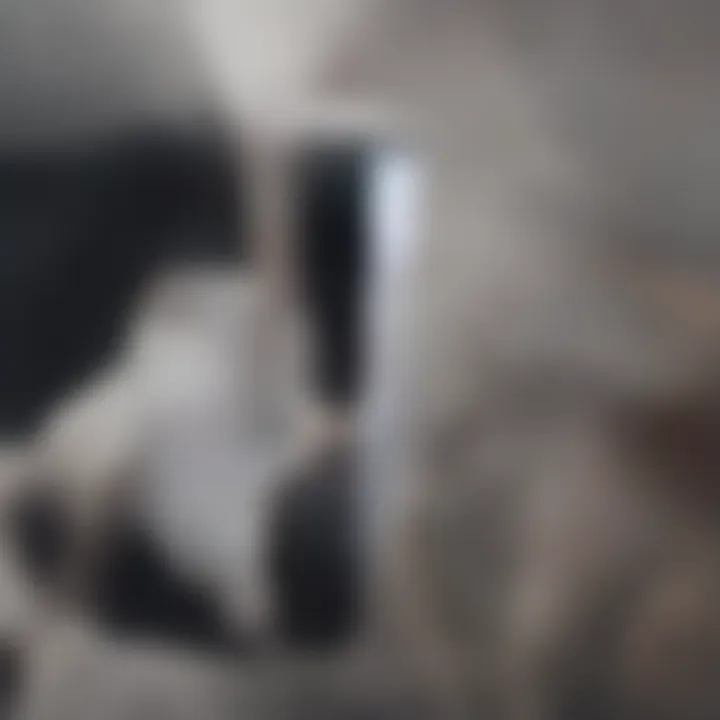
These registries can provide a comprehensive view of disease progression and management in real-world settings. They allow researchers to track treatment effectiveness over time and gather insights about patient behaviors and outcomes. This information can be pivotal in refining treatment guidelines and enhancing patient care.
Electronic Health Records
Electronic Health Records (EHRs) offer a rich source of data as they collect comprehensive patient information in one system. EHRs store a plethora of information, such as medical history, medications, lab results, and physician notes.
The integration of EHRs in diabetes research allows for real-time data analysis and monitoring. Researchers can study patterns in treatment adherence, medication effectiveness, and complication rates. Furthermore, EHRs facilitate the tracking of large populations, which can enhance the understanding of diabetes management at a broader scale.
Critical Variables in Diabetes Datasets
Understanding critical variables in diabetes datasets is essential for effective analysis and interpretation of the data. These variables underpin how healthcare professionals draw insights into diabetes management, patient care, and research trends. They help in identifying patterns, and potential risk factors, and evaluating treatment outcomes. Each variable contributes to the holistic understanding of diabetes and assists in tailoring personalized treatment plans.
Demographic Factors
Demographic factors are foundational in diabetes research. They encompass age, gender, ethnicity, income level, and education. These elements can influence the prevalence and management of diabetes. For instance, older individuals often experience different symptoms and responses to treatment compared to younger populations. Furthermore, socio-economic status can play a significant role in access to care and education about diabetes management strategies. Thus, analyzing demographic variables enables researchers to identify trends and tailor interventions to specific population needs.
- Age: Different age groups may exhibit distinct patterns in diabetes onset and progression.
- Gender: Hormonal differences can affect the development and management of diabetes.
- Ethnicity: Certain ethnic groups may have higher rates of diabetes, influencing targeted health initiatives.
Clinical Measurements
Clinical measurements are critical in determining the health status of individuals with diabetes. They include metrics such as blood glucose levels, HbA1c (glycated hemoglobin), blood pressure, and body mass index (BMI). These variables provide quantitative data that can be tracked over time, illuminating the effectiveness of treatment plans and guiding adjustments as necessary.
- Blood Glucose Levels: Directly indicate the body's ability to manage sugar intake.
- HbA1c: Offers insight into long-term glucose control, essential for assessing diabetes management effectiveness.
- Blood Pressure: Monitoring blood pressure is crucial due to the increased cardiovascular risk in diabetic patients.
Lifestyle Factors
Lifestyle factors also play a significant role in understanding diabetes datasets. These include physical activity, dietary habits, smoking status, and alcohol consumption. These variables often dictate patient behavior and disease outcomes. For instance, regular exercise and a balanced diet can lead to better blood sugar control, while poor lifestyle choices may exacerbate symptoms or lead to further complications.
"Lifestyle interventions are equally important as pharmacological treatments for diabetes management."
- Physical Activity: Regular exercise can improve insulin sensitivity and promote weight loss, crucial for diabetes control.
- Dietary Habits: Understanding what patients eat enables healthcare providers to make personalized dietary recommendations.
- Smoking and Alcohol: Both can have direct impacts on overall health and exacerbate diabetes complications.
By comprehensively examining these critical variables, researchers can achieve a nuanced understanding of diabetes. This understanding not only aids in effective treatment strategies but also informs public health policies aimed at preventing diabetes.
Data Collection Methodologies
Data collection methodologies play a vital role in understanding and using diabetes datasets; they ensure the integrity, reliability, and relevance of information gathered. The methods chosen significantly affect the data quality and the insights that can be drawn from the analysis. A rigorous data collection strategy makes it possible to design effective interventions and informs public health policy, thereby addressing diabetes more effectively.
Survey Designs
Survey designs are a cornerstone in collecting data on diabetes. They allow researchers to gather information directly from individuals affected by diabetes, capturing their experiences, behaviors, and health outcomes. Surveys can be administered in various formats, including online questionnaires, telephone interviews, or in-person assessments.
One of the key benefits of survey designs is their ability to reach a wide audience and collect data from diverse populations. This broad reach enhances the generalizability of findings. In addition, surveys can be tailored to specific research questions, allowing for targeted data collection. However, careful consideration is required when crafting survey questions to avoid biases and ensure clarity.
Key Considerations:
- Question Format: Open-ended vs closed-ended
- Sampling Methods: Random vs stratified sampling
- Response Rate: Strategies to improve participation
Retrospective Studies
Retrospective studies analyze existing data to investigate outcomes or associations. In diabetes research, these studies are often utilized to examine patient records in healthcare databases or registries. By looking backward in time, researchers can identify trends and patterns in diabetes prevalence, risk factors, and treatment outcomes.
These studies have strengths and weaknesses. A significant strength is the ability to study large patient populations without needing long follow-up periods. However, researchers must be mindful of potential biases due to missing data or inaccuracies in historical records. Furthermore, while retrospective studies can provide insights into correlations, they cannot establish causal relationships definitively.
Prospective Cohorts
Prospective cohort studies involve following a group of individuals over time, allowing researchers to observe how certain factors impact diabetes development and management. This methodology helps identify risk factors and the effects of interventions before any outcomes occur. Prospective studies can provide more reliable data about causation than retrospective studies.
However, they also demand significant resources, including time, funding, and participant commitment. Selecting an appropriate cohort is essential for the generalizability of results. Moreover, continuous data collection is needed to maintain the study's integrity, which can pose logistical challenges.
In summary, understanding data collection methodologies is integral to diabetes research. Each method—surveys, retrospective studies, or prospective cohorts—has its place in building a comprehensive diabetes dataset. The appropriate choice can enhance data quality and the applicability of research findings, ultimately informing better healthcare practices and policies.
Data Analysis Techniques
Data analysis techniques are crucial in the realm of diabetes datasets. They allow researchers to distill meaningful insights from large volumes of data, enabling better understanding of disease patterns and treatment outcomes. Employing the most appropriate techniques ensures that findings are reliable and relevant, ultimately guiding clinical practice and public health initiatives.
Statistical Methods
Statistical methods form the backbone of data analysis in diabetes research. They provide a framework for testing hypotheses, evaluating relationships, and drawing conclusions from datasets. Common techniques include descriptive statistics, which summarize data characteristics, and inferential statistics, which allow generalizations about populations based on sample data. Applying these methods helps researchers identify factors associated with diabetes progression, treatment effects, and patient characteristics.
Researchers utilize tools such as regression analysis to explore the relationship between variables. For instance, logistic regression often assesses the odds of developing diabetes based on predictive factors like age, gender, and lifestyle. These statistical evaluations inform public health strategies, guiding effective interventions tailored to specific populations.
Machine Learning Applications
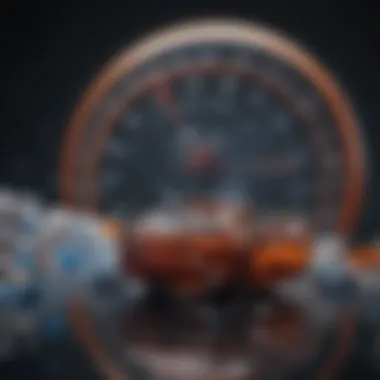
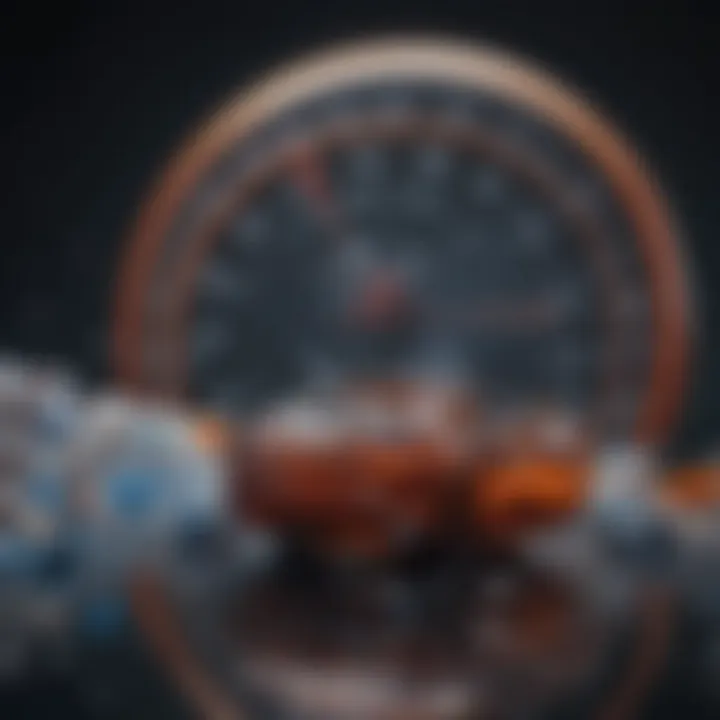
Machine learning applications have gained traction in diabetes research, offering advanced techniques to analyze complex datasets. Unlike traditional methods, machine learning can handle vast amounts of data, identifying patterns that may not be immediately apparent. Various algorithms, including decision trees, support vector machines, and neural networks, enable researchers to make predictions about diabetes risk and outcomes.
These applications are particularly valuable in personalized medicine, where individual patient data can guide tailored treatment plans. For example, a machine learning model can predict a patient’s response to insulin therapy based on historical data, thereby optimizing medication strategies. This dynamic approach is reshaping how diabetes is managed, as it emphasizes data-driven decision-making.
Predictive Modeling
Predictive modeling is another significant facet of data analysis techniques employed in diabetes research. It involves using historical data to forecast future outcomes, enabling proactive healthcare measures. For instance, models can predict the likelihood of diabetes onset based on a combination of lifestyle and genetic factors.
This approach not only aids in early detection but also influences policy development geared towards preventative care. Stakeholders can identify high-risk groups and allocate resources more effectively. Moreover, predictive models can evaluate the impact of interventions, informing changes in healthcare practices.
"Effective data analysis techniques are a key component in developing targeted interventions that can improve diabetes management across diverse populations."
In summary, data analysis techniques are fundamental to the exploration of diabetes datasets. Statistical methods offer a basis for understanding data, while machine learning applications and predictive modeling provide innovative solutions to complex healthcare challenges. Collectively, these techniques enhance research quality and applicability in the clinical setting.
Privacy and Ethical Considerations
In the realm of diabetes research, ensuring privacy and ethical handling of sensitive data stands as a cornerstone for fostering trust and compliance. These considerations are essential not only for fulfilling legal obligations but also for maintaining the integrity of research efforts. As datasets often contain personal health information, the adherence to privacy standards influences participant willingness and the validity of research outcomes.
Data Protection Regulations
Data protection regulations provide a framework to safeguard personal information within diabetes datasets. Authorities like the General Data Protection Regulation (GDPR) and the Health Insurance Portability and Accountability Act (HIPAA) lay down strict guidelines. They aim to prevent misuse of health data, ensuring that only authorized individuals have access to sensitive information.
Researchers must remain informed about these regulations when analyzing or sharing data. Failure to comply can result in severe penalties, which may include fines and damage to an institution's reputation. Moreover, establishing transparent data collection policies can enhance participant confidence, encouraging broader participation in diabetes studies.
Informed Consent Protocols
Informed consent protocols form the bedrock of ethical data utilization. Participants must be fully informed about how their data will be collected, stored, and used before agreeing to partake in studies. This process not only empowers individuals but also establishes a partnership between researchers and participants.
Effective informed consent should cover key aspects:
- The purpose of data collection
- Types of data being collected
- Any potential risks involved
- Procedures for maintaining confidentiality
- Options for participants to withdraw consent at any time
By prioritizing informed consent, researchers uphold ethical standards and foster trust. This is particularly crucial in fields like diabetes research, where personal health decisions significantly impact study outcomes.
Anonymization Techniques
Anonymization techniques are critical for protecting participant identity while allowing for the analysis of diabetes datasets. These methods involve removing or modifying personal identifiers from the data, rendering it impossible to trace the information back to individual participants.
Common anonymization techniques include:
- Data Masking: Altering data in a way that retains its analytical value without revealing personal details.
- Aggregation: Presenting data in a summarized format to conceal individual records.
- Pseudonymization: Replacing private identifiers with fictitious names or other non-identifying labels.
Implementing these techniques not only complies with ethical standards but also significantly mitigates risks associated with data breaches. As diabetes datasets contribute to critical research and public health policies, maintaining participant anonymity becomes an ethical requisite.
Applications of Diabetes Datasets
The utilization of diabetes datasets plays a crucial role in enhancing our understanding of diabetes and improving management strategies. These datasets provide insights that can inform public health decisions, contribute to personalized medical approaches, and help craft effective policy changes. Therefore, comprehensively reviewing the applications of diabetes datasets is essential for professionals and researchers in the field.
Public Health Insights
Diabetes datasets are vital in shaping public health initiatives. They help identify population-wide trends in diabetes prevalence and compounding factors such as obesity. By analyzing demographic data, researchers can understand which populations are most at risk and design targeted interventions.
For instance, using data from electronic health records, statisticians might notice an increase in diabetes cases among a specific age group in a certain location. This information enables health authorities to allocate resources efficiently and implement community programs.
Moreover, these datasets facilitate epidemiological studies that track the effectiveness of public health interventions over time. A continual analysis can reveal whether initiatives like screening programs or educational campaigns are successful in reducing new diabetes diagnoses. The ultimate goal is to leverage these insights to improve overall public health outcomes.
Personalized Medicine Approaches
The rise of personalized medicine is another significant application of diabetes datasets. With advanced data analytics, clinicians can examine an individual’s health data to tailor treatment plans based on specific needs and conditions.
This approach considers a range of factors, including genetic predispositions, lifestyle choices, and comorbid health issues. For example, analyzing patient registries can help determine how different subpopulations respond to medications such as metformin. By understanding these variations, healthcare providers can personalize treatment more effectively.
Additionally, machine learning applications can identify patterns within large diabetes datasets, allowing for predictions about which treatments are likely to be most effective for individual patients. This level of precision leads to better management of diabetes, improving patient outcomes and reducing healthcare costs.
Policy Development
Diabetes datasets are crucial in informing health policy development. By understanding the complexities of diabetes through comprehensive data analysis, policymakers can create more effective health regulations and resource allocation strategies.
For example, evidence gathered from clinical trial data regarding the economic impact of diabetes treatments can support funding decisions for public health programs. Moreover, insights from patient registries can highlight gaps in current care practices, prompting reforms.
Moreover, integrating various data sources assists in developing a holistic view of the diabetes landscape. This can lead to more informed decisions around prevention strategies, screening guidelines, and healthcare inequality should be addressed.
By leveraging diabetes datasets, health policymakers can make well-grounded decisions that directly affect public health outcomes.
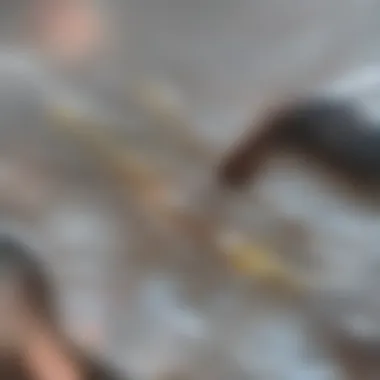
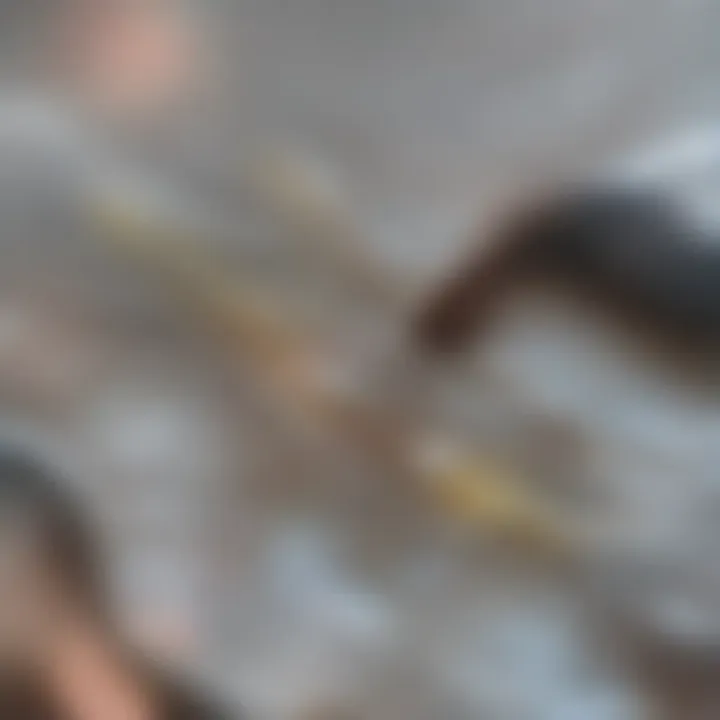
Challenges in Using Diabetes Datasets
The use of diabetes datasets is essential for advancing research and improving treatment outcomes. However, various challenges arise when working with these datasets. Addressing these challenges is crucial for researchers and healthcare professionals who rely on accurate data to make informed decisions. This section examines the critical difficulties faced in using diabetes datasets, emphasizing their importance in ensuring the reliability and validity of conclusions drawn from the data.
Data Quality Issues
One of the foremost challenges is data quality. Issues such as missing, incorrect, or outdated information can significantly skew results. Diabetes datasets often originate from disparate sources, leading to inconsistencies. Researchers must therefore prioritise data cleaning and validation processes.
- Missing Data: It is common in health-related datasets. If a key variable is missing, it may impair the analysis.
- Inconsistent Measurement: Different studies may measure the same variable differently, resulting in complications when merging datasets.
- Outdated Information: Health guidelines and diagnostic standards evolve, making older data less relevant or accurate.
Focusing on these concerns is vital. All stakeholders in diabetes research need to emphasize rigorous data management practices.
Integration of Diverse Data Sources
Another significant challenge involves the integration of diverse data sources. As noted, diabetes datasets can come from various origins, including electronic health records, clinical trials, and patient registries. This diversity complicates data unification. Unique identifiers, formats, and coding schemes can make it difficult to synthesize information effectively. For example:
- Different Coding Systems: Various health systems might use different codes for the same diagnosis, complicating data harmonization.
- Variability in Definitions: Terms like 'type 2 diabetes' may be defined inconsistently across studies.
- Merging Formats: Different datasets may have differing structures, requiring additional time and resources for integration.
Resolving these integration challenges is essential for producing reliable insights into diabetes management.
Analytical Limitations
Lastly, analytical limitations pose a notable obstacle when working with diabetes datasets. The complexity of diabetes itself, along with the multifaceted nature of the datasets, means that simplistic analyses may not capture the nuances necessary for drawing accurate conclusions.
- Bias in Analysis: Certain analytical methods may introduce bias if the underlying assumptions do not align with the data.
- Overfitting Models: When dealing with high-dimensional data, there is a risk that models become overly tailored to the training data and fail to generalize.
- Inadequate Statistical Methods: Researchers must be equipped with an understanding of advanced statistical techniques to draw meaningful insights.
Such analytical challenges can be mitigated through proper training and awareness of the tools used in data analysis. Researchers and practitioners must maintain a critical eye towards the limitations inherent in their datasets and methodologies.
In summary, addressing the challenges related to data quality, integration of diverse sources, and analytical limitations enhances the credibility of findings in diabetes research. This is vital for informing future healthcare practices and policies.
Future Directions in Diabetes Research
Diabetes research is continuously evolving. Understanding the future of this field is essential for healthcare professionals and researchers alike. It allows them to anticipate changes in treatment, management, and prevention strategies. This section focuses on key areas where advancements are expected to have a significant impact on diabetes outcomes.
Emerging Technologies
The integration of novel technologies into diabetes research holds great promise. Wearable devices, such as continuous glucose monitors, provide real-time data on glucose levels. This immediate feedback is invaluable for patients and clinicians. Mobile health applications also allow patients to track their diet, exercise, and medication adherence. These tools create a more holistic approach to diabetes management, enabling personalized interventions based on real-time data.
Data analytics plays a crucial role too. Machine learning algorithms can analyze vast amounts of data from various sources, predicting patient outcomes more accurately. As technologies develop, the potential to individualize treatment plans becomes more attainable, ultimately leading to improved glycemic control and better patient outcomes.
Interdisciplinary Approaches
Collaboration among different fields is becoming a key aspect of effective diabetes research. Combining expertise from medicine, technology, psychology, and sociology can lead to more comprehensive understanding and treatment strategies. For instance, incorporating behavioral science insights can help develop interventions that encourage healthier lifestyle choices among individuals with diabetes.
Healthcare organizations are beginning to recognize the benefits of interdisciplinary collaborations. This often involves creating teams that include clinicians, data scientists, and public health experts. The diverse perspectives can enhance the research process. They enable researchers to design studies that address multiple dimensions of diabetes care, making results more applicable to real-world settings.
Global Health Perspectives
Diabetes is a global issue. The incidence is rising across all continents, and addressing this requires a worldwide effort. Future research must consider the health disparities that exist in diabetes care. Understanding cultural, economic, and geographical factors can direct better resource allocation to underserved populations.
Additionally, partnerships between countries can enhance the global health response to diabetes. Sharing data and best practices can lead to better health policies and more effective intervention strategies. Global health organizations should continue emphasizing the importance of diabetes as a priority area, especially as it intersects with other health issues such as obesity and cardiovascular diseases.
"Collaborative international efforts can lead to groundbreaking advancements in understanding diabetes and formulating effective interventions."
In summary, the future of diabetes research lies in emerging technologies that personalize treatment, interdisciplinary approaches that enhance the understanding of the disease, and global perspectives that address health disparities. Recognizing these directions can inspire continued innovation and research in the field of diabetes.
Epilogues
In this article, conclusions serve as a critical synthesis of the discussions presented throughout. They encapsulate the main findings and insights regarding diabetes datasets, providing readers with a clear understanding of the implications of this data in both research and clinical practices. The conclusions section highlights specific elements, such as the pivotal role these datasets play in disease management, treatment efficacy, and policy formulation in public health.
Summary of Key Points
The primary takeaways of the article include:
- Diversity of Data Sources: Diabetes datasets originate from varied sources including clinical trials, epidemiological studies, and electronic health records. Each source contributes uniquely to knowledge about diabetes.
- Critical Variables: Important factors such as demographic information, clinical metrics, and lifestyle choices significantly influence the understanding of diabetes and its treatment.
- Data Collection Methodologies: Understanding different methodologies, like survey designs and retrospective studies, aids in effective data utilization.
- Analytical Techniques: Emphasis on statistical methods and machine learning applications shows how modern technology is being harnessed to analyze complex datasets.
- Ethical Considerations: The section stresses the importance of privacy and informed consent, underscoring the responsibility researchers have in protecting sensitive patient information.
Significance for Healthcare Professionals
Healthcare professionals stand to gain significantly from the insights derived from diabetes datasets. By leveraging comprehensive data analysis, they can improve patient outcomes through:
- Informed Clinical Decisions: Access to data enables practitioners to make evidence-based decisions customized to individual patient profiles, enhancing treatment effectiveness.
- Public Health Strategies: Understanding population-level data aids in constructing public health initiatives aimed at diabetes prevention and management.
- Enhanced Patient Engagement: Utilizing data to tailor patient education materials fosters better patient involvement in their own healthcare journeys.
Call for Continued Research Efforts
The field of diabetes research is continuously evolving. There is a clear need for sustained efforts in this area, characterized by:
- Advancements in Data Technologies: Novel methods for data collection and analysis hold promise for even richer datasets, allowing for deeper insights.
- Interdisciplinary Collaboration: Integrating knowledge from various fields such as genetics, nutrition, and psychology can lead to more innovative approaches in tackling diabetes.
- Global Data Sharing: Establishing platforms for sharing data internationally can enrich research and improve outcomes across diverse populations.
"The future of diabetes research depends on our capacity to understand, analyze, and utilize vast amounts of data while upholding the highest ethical standards."