RNA Sequence Analysis: Key Methods and Their Applications
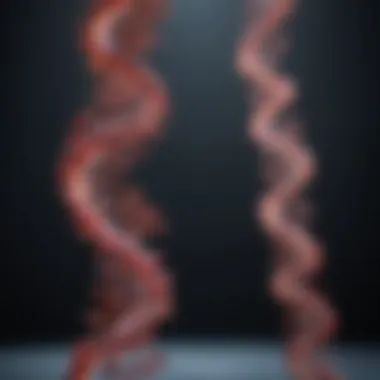
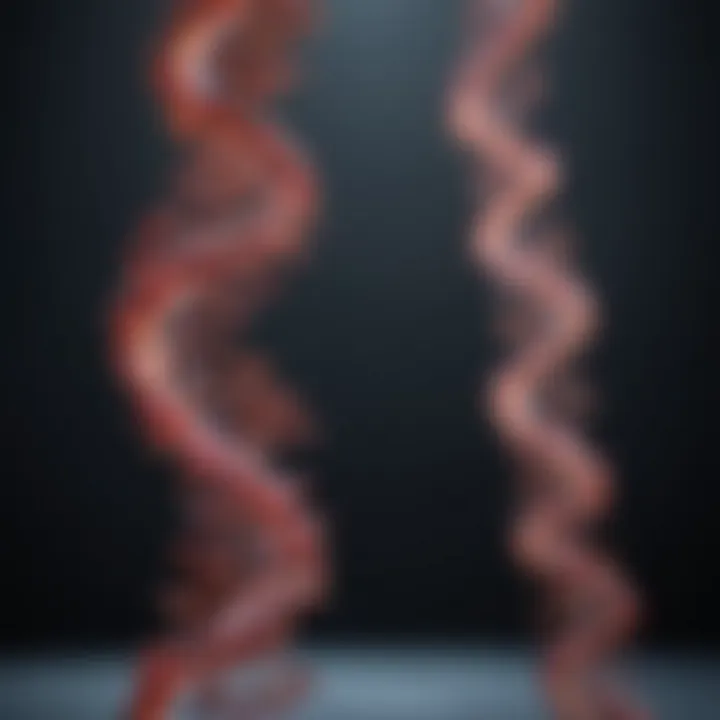
Intro
In recent years, the domain of RNA sequence analysis has gained considerable traction due to its pivotal role in understanding the complexities of gene regulation and expression. This area of research is not just confined to deciphering the genetic code but expands into the realms of genomics and transcriptomics, influencing various scientific and medical fields. With the emergence of advanced sequencing technologies, the methodologies available for RNA analysis have evolved, providing researchers and clinicians with powerful tools to unlock the secrets held within RNA molecules.
Through this article, we aim to bridge the gap between the intricate scientific ideas surrounding RNA analysis and their practical implications in contemporary research. Understanding RNA's significance is paramount as it serves as a key player in processes like transcription, splicing, and translation, making it essential for numerous biological functions.
Thus, it’s crucial to delve into how RNA sequence analysis is conducted, starting from basic techniques to advanced applications that shape modern science. By navigating through these topics, we intend to equip readers with the knowledge necessary to appreciate the depth and breadth of this field, as well as its future potential.
Research Methodology
Description of research design and approach
When approaching RNA sequence analysis, a structured research methodology is of utmost importance. It often begins with the selection of appropriate RNA samples, which could be sourced from various tissues or cell types depending on the research question at hand. Researchers typically utilize a combination of experimental and computational methods to derive meaningful insights.
One common approach is high-throughput sequencing, which allows for the rapid collection of large volumes of RNA data. This technique encompasses several steps, including RNA extraction, cDNA synthesis, library preparation, and sequencing. Each stage is critical, as the quality of the RNA and the protocols used can significantly influence the final results.
Materials and methods used in the study
The materials and methods employed vary based on specific research needs but typically include:
- RNA extraction kits: These are crucial for isolating high-quality RNA. Popular brands include TRIzol and Qiagen.
- Sequencing platforms: Illumina and Oxford Nanopore are examples of platforms that allow for comprehensive RNA sequencing.
- Bioinformatics tools: Software like STAR and HISAT2 is essential for aligning the RNA sequences to reference genomes, while DESeq2 and edgeR are commonly used for differential expression analysis.
Manual and automated methods may be used to process and analyze data, ensuring that the findings are replicable and reliable. The application of varying techniques allows for a comprehensive understanding of RNA behavior under different biological conditions.
Discussion and Interpretation
Interpretation of results in the context of existing literature
After the data collection, the real challenge revolves around interpreting the results. RNA sequencing can yield a wealth of information, but to make the most of it, researchers must contextualize their findings within the broader landscape of existing literature. By comparing the newly acquired data with known gene expression profiles, researchers can validate their results and contribute to the cumulative knowledge in the field.
Implications for future research or practical applications
The implications of RNA sequence analysis extend far beyond theoretical understanding. For instance, in the context of personalized medicine, insights derived from RNA analytics can help tailor treatments to individuals based on their specific gene expression signatures. Furthermore, this technology opens doors to new discoveries in areas like cancer research, where understanding RNA dynamics can lead to novel therapeutic strategies.
In summary, RNA sequence analysis stands at the crossroads of biology and technology, making it a vital area of study. Its methodologies not only enhance our comprehension of genetic expression but also pave the way for future explorations that could transform medical practices and therapeutic approaches.
Intro to RNA Sequence Analysis
RNA sequence analysis has emerged as a cornerstone in our understanding of genetic expression and cellular dynamics. This area of study is not just an academic interest; it’s vital for advances in fields such as medicine, biotechnology, and environmental science.
Importance of RNA Sequence Analysis
The centrality of RNA in various biological processes cannot be overstated. RNA molecules play pivotal roles in translating genetic information into functional entities within a cell. The ability to analyze RNA sequences allows researchers to uncover the mysteries surrounding gene regulation, RNA modifications, and interaction networks.
Here are some key elements that drive the relevance of RNA sequence analysis:
- Gene Expression Profiling: Investigating how genes are expressed under different conditions yields insights into cellular responses and behavior.
- Comparative Analysis: By assessing RNA sequences across species or conditions, scientists can pinpoint evolutionary changes and functional diversifications.
- Disease Mechanisms: Identifying aberrant RNA expression patterns is crucial for understanding diseases, leading the way toward novel diagnostics and therapeutics.
The benefits of deepening our comprehension of RNA sequences extend far and wide. For instance, the knowledge gained contributes significantly to personalized medicine, allowing clinicians to tailor treatments based on individual genetic profiles.
Considerations About RNA Sequence Analysis
However, diving into RNA sequence analysis is not without its challenges. The complexity of RNA molecules, including various types such as mRNA, tRNA, and miRNA, can lead to ambiguous interpretations without robust analytical tools and methodologies. Also, the processing of large RNA datasets necessitates sophisticated bioinformatic approaches, which can be a bottleneck for some researchers.
In summary, RNA sequence analysis bridges the gap between theoretical knowledge and practical application in biological sciences. By providing a rich array of information, it informs various applications, from understanding cellular functions to uncovering the mechanisms of diseases. The exploration of this field promises continued innovations and breakthroughs in both research and clinical settings, marking it as a focal point for scientists and professionals alike.
The significance of RNA sequences lies at the heart of decoding the biological blueprint, unlocking secrets that could lead to new therapies and understanding of life itself.
Fundamental Concepts in RNA Sequencing
In the ever-evolving landscape of molecular biology, understanding the fundamental concepts in RNA sequencing is vital. This section lays the groundwork for grasping how RNA sequencing works and its implications in research. At its core, RNA sequencing provides insights into gene expression and regulation, offering a window into cellular behavior under various conditions. Understanding these concepts is not just pivotal for researchers but anyone interested in the intricate dance of genes and their expressions.
Types of RNA and Their Roles
RNA (Ribonucleic Acid) comes in several varieties, each playing distinct roles in cellular function. Here are the primary types:
- mRNA (Messenger RNA): Serves as the template for protein synthesis, carrying instructions from DNA to ribosomes, where proteins are made.
- tRNA (Transfer RNA): Contributes to the translation of mRNA into amino acids, thus linking the genetic code to the protein structure.
- rRNA (Ribosomal RNA): Forms the core of ribosomes and is crucial for protein synthesis.
- Non-coding RNAs: These consist of various types like miRNA and lncRNA, which participate in regulatory functions and gene expression modulation.
Understanding these types of RNA is fundamental to RNA sequencing, as it aids in the contextual interpretation of sequencing data. Knowing the roles of different RNAs can guide researchers in designing experiments and analyzing outcomes.
Overview of RNA Sequencing Technologies
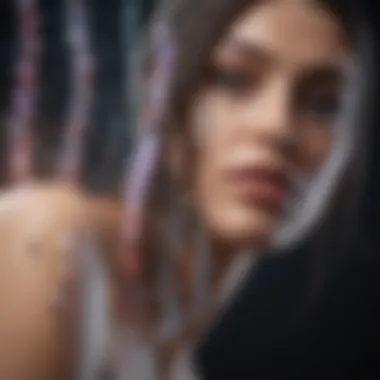
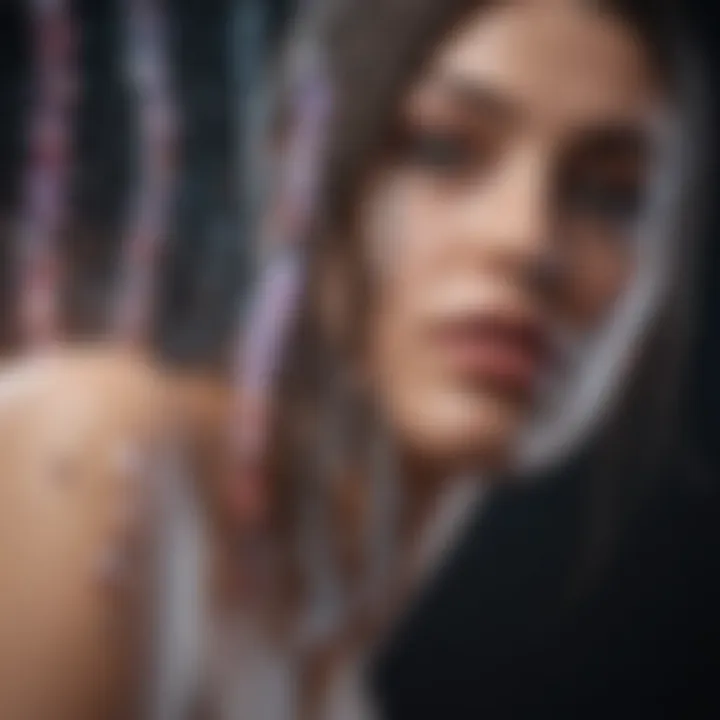
As technology advances, several RNA sequencing methods have emerged, each providing unique advantages and insights. Let's explore three notable types:
Next-Generation Sequencing
Next-generation sequencing (NGS) revolutionized the field by allowing parallel sequencing of multiple DNA fragments. Its primary advantage is the massive throughput it offers, enabling researchers to generate large amounts of data in a single run. This characteristic makes NGS a popular choice for many applications, including whole-transcriptome sequencing.
A unique aspect of NGS is its flexibility; it can be tailored for different applications, whether it’s targeted sequencing or broader genomic studies. However, the complexity of the data can present challenges, requiring robust bioinformatics tools for accurate analysis.
Single-Cell RNA Sequencing
Single-cell RNA sequencing (scRNA-seq) takes precision to the next level by focusing on individual cells rather than bulk populations. This method dives deep into the heterogeneity of cell types, allowing researchers to pinpoint gene expression variations crucial for understanding development and disease progression.
What sets scRNA-seq apart is its ability to reveal the unique transcriptomic landscape of each cell, a feature that opens new avenues in personalized medicine. However, the technology’s sensitivity and complexity necessitate meticulous sample preparation and analysis.
Long-Read Sequencing
Long-read sequencing technologies like PacBio and Oxford Nanopore provide extended reads, making them exceptional for resolving complex genomic regions often missed by shorter read technologies. The major selling point here is their ability to address structural variations and splice variants with greater accuracy.
Nevertheless, this technology can be costly and may involve longer processing times, which is often seen as a trade-off for the deeper insights it provides. Each sequencing approach comes with its unique set of advantages and limitations, making it crucial for researchers to select the most appropriate technology based on their specific research objectives.
Understanding the various RNA sequencing technologies and their nuances equips researchers with the clarity needed to navigate their applications effectively. As the field progresses, these foundational concepts will remain integral to the broader dialogue on genetic research and therapeutic developments.
Preparation of RNA Samples for Sequencing
Preparation of RNA samples serves as the backbone of successful RNA sequencing endeavors. Without proper preparation, researchers may encounter substantial hurdles down the road, consequently undermining the accuracy and reliability of the results. A carefully structured preparation process ensures that the RNA obtained is of high quality and integrity, which is essential for accurate sequencing and downstream analyses. In this section, we will delve into the critical steps involved in the isolation and purification of RNA, as well as the library preparation techniques pivotal for effective sequencing.
Isolation and Purification of RNA
Isolation and purification of RNA is akin to finding a needle in a haystack. Since RNA is often present in varying amounts within biological samples, extracting it cleanly requires finesse and precision. The goal here is to separate RNA from DNA, proteins, and other contaminants that could skew results later on.
The methods typically employed include:
- TRIzol Reagent: A classic in RNA isolation for its capacity to disrupt cells and solubilize their contents, followed by a phenol-chloroform extraction.
- Silica-based Columns: These use a filtration system that binds RNA while allowing impurities to wash away, producing high-purity RNA quickly.
The choice of method can significantly affect the yield and quality of the RNA. In many scenarios, good quality RNA is assessed by its A260/A280 ratio, which indicates the purity of the RNA against protein contamination. High-quality RNA allows for better downstream applications, enhancing overall reliability in data interpretation.
Library Preparation Techniques
When preparing RNA samples, library preparation comes next and is critical for turning raw RNA into a format compatible with sequencing platforms. This involves several steps, including cDNA synthesis and fragmentation methods, each playing a vital role in optimizing the RNA for sequencing.
cDNA Synthesis
cDNA synthesis is a fundamental aspect of preparing RNA libraries. This technique involves the reverse transcription of RNA into complementary DNA (cDNA), which is more stable and easier to manipulate than its RNA counterpart. One of the critical characteristics of cDNA synthesis is its ability to amplify specific RNA regions, offering researchers a deeper insight into gene expression patterns.
Key benefits of cDNA synthesis include:
- Stability: Compared to RNA, cDNA is less prone to degradation.
- Sensitivity: This method allows for detection of low abundance transcripts, which is crucial when studying nuanced biological processes.
However, a unique factor is cDNA's dependence on reverse transcriptase enzymes, which can introduce errors during transcription. These issues can sometimes complicate downstream analyses, though advancements in enzyme technology have mitigated many past concerns.
Fragmentation Methods
Fragmentation methods facilitate the breaking up of long RNA or cDNA sequences into smaller, more manageable pieces. This is important as most sequencing technologies favor shorter fragments, thus enhancing the efficiency of the sequencing process. Common techniques for fragmentation include enzymatic digestion, mechanical shearing, and sonication.
The distinctive characteristic here is the balance required between fragment size and quantity. If the fragments are too short, vital sequence information could be lost; if they are too long, it may lead to poor performance in sequencing workflows. Notably, quality control measures, such as bioanalyzer or gel electrophoresis analysis, are instrumental in confirming that fragments meet the guidelines for sequencer input.
Rocking the boat on traditional approaches, newer methods like
Advantages:
- Flexibility in adaptating to different sequences.
- Potentially higher yields.
have revolutionized how researchers view fragmentation in RNA sequencing.
In summary, meticulously preparing RNA samples through effective isolation, purification, and proper library preparation techniques ensures high-quality data, setting the stage for accurate sequencing results. This careful groundwork is critical, as data quality ultimately drives insights into gene expression and biological understanding.
Analytical Techniques in RNA Sequence Analysis
Understanding analytical techniques in RNA sequence analysis is crucial, not just for professionals in the field but also for students or educators diving into the complexities of RNA research. These techniques serve as the backbone for interpreting sequencing data, ensuring that insights gained are accurate and relevant. Analytical methods can elevate the quality of the research, allowing for discoveries that can influence various domains—from genomics to medical research.
Quality Control of RNA Sequencing Data
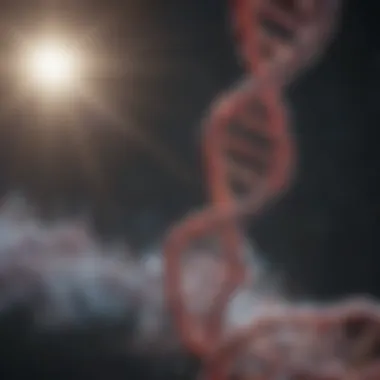
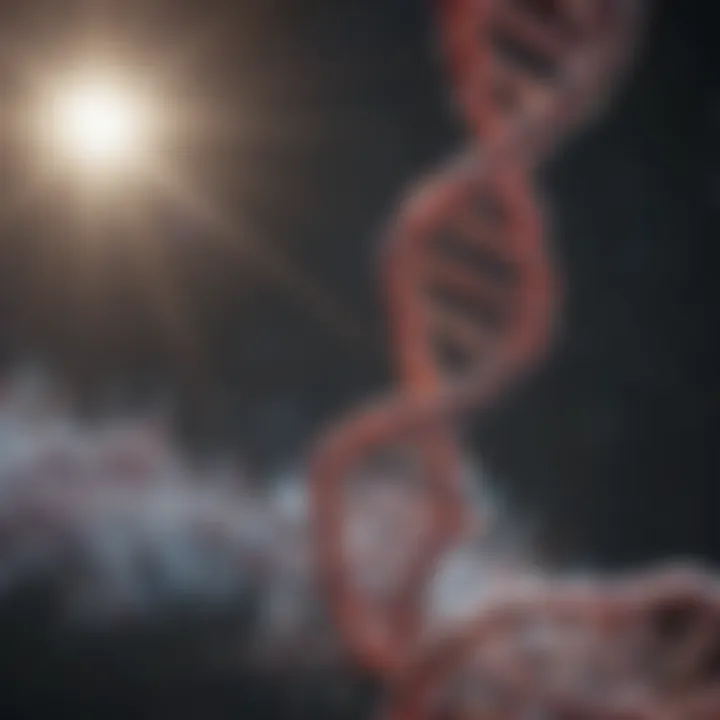
Quality control (QC) is the first line of defense in RNA sequencing. It’s where researchers sift through mountains of raw data to eliminate noise and ensure accuracy. Without a solid QC process, it’s like handing in a report full of typos—you lose credibility immediately.
Several key procedures are part of this process:
- Assessing Read Quality: Using tools like FastQC, researchers can visualize metrics such as read length distributions and GC content. This step helps identify potentially problematic areas in the sequencing data.
- Trimming and Filtering: Tools like Trimmomatic or Cutadapt help remove low-quality reads and adapter sequences, which can skew results if left unchecked. A clean dataset is essential for meaningful analysis.
- Duplication Checks: Identifying duplicate reads is vital, especially in complex samples. Software such as Picard can be employed to analyze the extent of duplication, influencing subsequent mapping and expression quantification.
These QC steps are akin to proofreading a manuscript; they may seem tedious but are necessary to present polished, reliable data.
Data Alignment and Mapping Strategies
Once quality control is done, the next task is aligning reads to a reference genome or transcriptome. This step is essential because precise alignment ensures that researchers can accurately associate the reads with specific genes or genomic regions.
Common mapping strategies include:
- Reference Mapping: Using tools like STAR or HISAT2 allows for rapid alignment. Researchers typically choose reference genomes based on the organism being studied. Given the complexity involved, these tools leverage sophisticated algorithms to accommodate splicing and other genomic intricacies.
- De Novo Assembly: In cases where a reference is absent, de novo assembly can be employed. Programs such as Trinity facilitate this process, piecing together reads to reconstruct transcript sequences. However, this route can come with its own set of challenges regarding computational resources and output reliability.
The choice between these strategies is significant, much like deciding between following a map or navigating by landmarks; each approach has its own merits and potential pitfalls.
Differential Expression Analysis
The terminology might sound complex, but at its core, differential expression analysis allows researchers to identify which genes are turned on or off under different conditions. This could be crucial in understanding diseases or adapting treatments.
Key approaches include:
- Statistical Methods: Packages like DESeq2 and EdgeR are commonly used for data normalization and to perform statistical tests that help ascertain if gene expression changes are significant, providing a robust statistical backing for the findings.
- Gene Set Enrichment Analysis (GSEA): This tool can further illuminate the biological implications behind the differential data. By grouping genes into predefined sets, GSEA reveals whether specific pathways or processes are upregulated or downregulated in a given dataset.
The insights gained from these analyses can be transformative. They might shed light on potential treatment pathways or reveal therapeutic targets for diseases, much like a road map guiding researchers toward new frontiers in biology.
Bioinformatics Tools for RNA Analysis
In the realm of RNA sequence analysis, bioinformatics tools serve as the backbone, facilitating the interpretation of complex biological data. The combination of vast amounts of sequence information and insightful computational techniques allows researchers to uncover biological insights with greater accuracy and speed. This section will delve into the importance of bioinformatics tools, focusing on their specific elements, benefits, and considerations that come into play when leveraging these technologies in RNA analysis.
Software for Sequence Assembly
The assembly of RNA sequences is a critical first step towards rich biological interpretation. Software tools designed for this purpose play a pivotal role in reconstructing the original sequences from short reads generated by sequencing technologies. Programs such as STAR, Trinity, and SPAdes are particularly notable, each offering unique advantages.
- STAR: This tool is tailored for rapid alignments of RNA sequences, handling large datasets efficiently. Its ability to accommodate splice-aware alignments helps in uncovering gene structures more accurately.
- Trinity: Often hailed as a gold standard in de novo assembly, Trinity provides a robust framework for assembling transcripts from RNA-Seq data even when a reference genome is unavailable. It excels in generating high-quality transcriptomes, which can be used for downstream analyses such as differential expression.
- SPAdes: Originally developed for genome assembly, SPAdes has extended its capabilities to RNA assembly as well. It’s designed to piece together fragmented transcripts, thus ensuring even low-abundance transcripts are included in the analysis.
These tools, among others, empower researchers by enhancing the precision of RNA sequence assemblies. However, choosing the right software hinges on the specific needs of the project, including the type of data being analyzed and the desired output.
Statistical Packages for Data Analysis
Once the sequences are assembled, the next step involves analyzing the data effectively. Statistical packages play an instrumental role in this phase. They support researchers in quantifying gene expression, assessing statistical significance, and interpreting results. Popular tools like R/Bioconductor, DESeq2, and EdgeR are widely used for such applications.
- R/Bioconductor: This is an open-source project that provides tools for the analysis and comprehension of high-throughput genomic data. Its comprehensive ecosystem offers numerous packages that cater to different aspects of RNA sequencing data.
- DESeq2: Primarily used for differential gene expression analysis, DESeq2 employs statistical methods to account for variations in sequencing depth and other factors, yielding reliable results even in complex datasets.
- EdgeR: Similar to DESeq2, EdgeR specializes in analyzing count data and is particularly effective for RNA-Seq experiments. Its statistical models are tailored to handle low-count genes, making it a critical tool in RNA analysis.
Inclusion of these packages allows for a rigorous approach to data analysis, ensuring that biological conclusions are well-supported by statistical evidence. However, users must keep in mind the assumptions underlying these models to avoid misleading outcomes.
Visualization Tools for RNA Data
A picture is worth a thousand words, and when it comes to RNA sequence data, visualization tools bridge the gap between complex statistical results and interpretative insights. Tools like ggplot2, Circlize, and Integrative Genomics Viewer (IGV) enable researchers to transform data into intuitive visual formats that can highlight patterns, trends, and anomalies within the dataset.
- ggplot2: This R package is essential for creating a wide range of static and interactive plots. It allows researchers to showcase gene expression distributions, heat maps, and other graphical representations that illuminate their findings.
- Circlize: For exploring relationships in complex datasets, Circlize facilitates the generation of circular visualizations. This is particularly useful when examining interactions between different genes or pathways.
- Integrative Genomics Viewer (IGV): IGV is a powerful tool for visualizing large-scale genomic data. With its user-friendly interface, researchers can navigate through extensive RNA-Seq datasets, viewing alignments and discovering structural variations configurations.
In summary, the selection of appropriate bioinformatics tools is fundamentally necessary for RNA sequence analysis. By harnessing the capabilities of software for sequence assembly, statistical packages for data analysis, and visualization tools, researchers enhance both the analysis process and the overall quality of their biological insights. As they navigate the intricate path of RNA research, a thorough understanding of these tools can significantly elevate the impact of their discoveries.
"Bioinformatics tools have fundamentally changed the landscape of RNA analysis, enabling researchers to derive insights that were previously unimaginable."
Each of these components serves not just a functional role; collectively, they form an ecosystem of analytical approaches that lies at the heart of modern molecular biology. The ongoing evolution of bioinformatics continues to shape the future of RNA analysis, making it an ever-exciting field of study for students, researchers, and educators alike.
Applications of RNA Sequence Analysis
RNA sequence analysis has become a cornerstone in the fields of genomics and transcriptomics, playing a pivotal role in our understanding of biological processes. The myriad applications lie at the intersection of basic research and clinical advancements, making it an area of immense significance. It allows scientists to decipher gene expression patterns, identify novel RNA molecules, and unravel the complexities of cellular functions. The pivotal point of this section is to discuss how RNA sequence analysis not only enhances our knowledge but also influences the approaches to disease diagnosis and treatment strategies.
Role in Genomics and Transcriptomics
In genomics and transcriptomics, RNA analysis provides insights into the dynamic regulation of genes. By studying RNA molecules, researchers can gain a keener understanding of how genes are expressed under various conditions, providing the keys to unlocking gene function and interaction pathways.
- Gene Expression Profiling: One primary application is gene expression profiling, which helps in measuring the expression levels of thousands of genes simultaneously. This data can illustrate how genes respond to different stimuli, such as environmental changes or disease states.
- Alternative Splicing Identification: RNA sequencing enables the identification of alternative splicing events. This means that scientists can discover how a single gene can produce multiple RNA variants, resulting in different proteins. Given their role in development and disease, understanding alternative splicing is crucial.
- Non-coding RNA Research: With the discovery of various non-coding RNAs, RNA sequence analysis helps elucidate their functions and mechanisms. Non-coding RNAs, such as microRNAs and long non-coding RNAs, have significant roles in regulating gene expression, thereby impacting numerous biological processes.
Implications in Disease Research
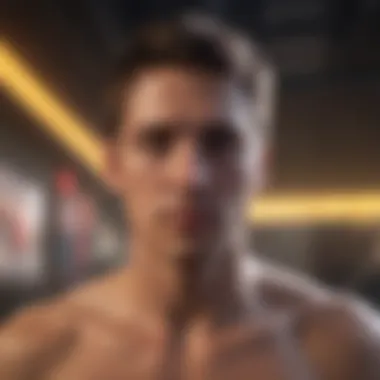
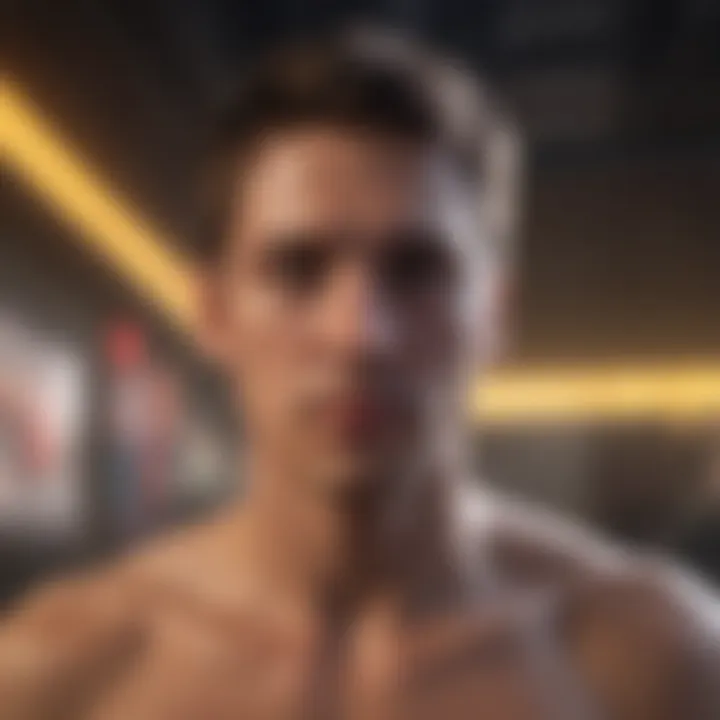
RNA sequence analysis extends its impact significantly into disease research, providing critical insights into the molecular underpinnings of various conditions.
Cancer Genomics
Cancer genomics has seen remarkable advancements through RNA sequence analysis. It focuses on identifying gene expression signatures associated with cancer types, aiding in personalized treatment approaches.
- Key Characteristic: One key characteristic of cancer genomics is its ability to profile tumors based on their RNA expression patterns. This profiling lays the groundwork for targeted therapies, where treatments can be tailored to the molecular characteristics of an individual’s cancer.
- Unique Feature: Through RNA-seq, researchers can detect fusion genes and aberrant splicing events that are often pivotal in cancer development. This feature aids in identifying novel therapeutic targets, enabling a more precise attack on cancerous cells.
- Advantages: The outcomes from cancer genomics offer pathways to better prognostic markers and refined treatment strategies. However, challenges remain, particularly with the interpretation of vast datasets and the integration of findings into clinical practice, which requires a nuanced approach.
Infectious Diseases
The analysis of RNA plays a significant role in investigating infectious diseases, especially in tracking and understanding pathogen behavior.
- Key Characteristic: A crucial aspect of studying infectious diseases is the ability of RNA sequencing to reveal how pathogens express their genes during infection. Understanding these expression patterns is essential for developing effective treatments.
- Unique Feature: One unique feature of RNA analysis in this domain is the capability to monitor viral evolution in real-time. This can lead to actionable insights regarding emerging infectious diseases and their response to antiviral therapies.
- Advantages and Disadvantages: The advantages of using RNA analysis include improved diagnostics and real-time monitoring of outbreaks. However, challenges exist in terms of the variability in RNA expression levels, which can complicate data interpretation and hamper the determination of causative relationships.
"Understanding RNA's roles opens doors to new therapeutic strategies across various fields including cancer and infectious diseases."
Challenges and Considerations in RNA Sequence Analysis
The field of RNA sequence analysis presents a myriad of challenges and intricate considerations that necessitate thorough understanding and careful navigation. Acknowledging these obstacles and ethical implications is indispensable for anyone delving into RNA studies. Understanding the technical limitations and ethical considerations involved can make or break the success of research projects aimed at elucidating the complex roles of RNA in biology.
Technical Limitations of RNA Sequencing
When it comes to RNA sequencing, technology is both a boon and a bane. Several technical limitations can hinder the accuracy and reliability of the results obtained.
- Sequencing Bias: Different sequencing platforms can exhibit bias in how certain RNA molecules are amplified and sequenced. This can lead to inaccuracies in quantifying gene expression levels.
- Fragmentation Issues: During the library preparation stage, RNA can be fragmented in ways that can influence the final data. If the fragmentation is uneven, it may skew results and affect sensitivity.
- Low Abundance RNA: Detecting low abundance transcripts can be akin to finding a needle in a haystack. The sensitivity of the sequencing technology can impact the ability to capture these rare RNAs.
- Contamination Risks: The process requires meticulous handling to avoid contamination, which might introduce extraneous sequences. Even minute traces of unwanted RNA can lead to erroneous interpretations.
These limitations underline the importance of choosing appropriate methodologies and technologies carefully, as every detail can have cascading effects on the integrity of the data generated.
Ethical Considerations in RNA Research
As with any field that involves genetic material, RNA research doesn’t come without its fair share of ethical dilemmas. Researchers must grapple with various ethical considerations while conducting RNA analysis, especially concerning human subjects and animal models.
- Informed Consent: Ensuring that participants understand what their genetic data will be used for is critical. This includes potential implications for personal health and privacy. Many individuals may not realize that their RNA data could lead to insights into not only their health but also that of their family.
- Data Privacy: Protecting the identity of those who provide RNA samples is paramount. With the advancement in methodologies, actionable insights on an individual's health can potentially create misuse risks if data falls into the wrong hands.
- Use of Animal Models: When employing animal models for RNA studies, researchers must adhere to strict ethical guidelines, ensuring that the animals are treated humanely and with the utmost care.
- Dual-Use Concerns: The potential for RNA research to be misappropriated for nefarious purposes poses a significant ethical risk that cannot be overlooked. Researchers ought to consider the possible outcomes of their work well beyond the lab environment.
Thoroughly navigating the ethical landscape in RNA sequence analysis is as important as addressing the technical hurdles. The responsibility lies with the researcher, as the integrity and future of the field depend on it.
By tackling these challenges head-on, researchers can pave the way for reliable, responsible RNA analysis that not only expands our scientific knowledge but does so in an ethical and conscientious manner.
Future Directions in RNA Analysis
The study of RNA sequence analysis is continuously evolving. The future directions in this field hold tremendous potential, serving as a compass for researchers looking to explore complex biological systems. As scientists delve deeper into RNA's roles in cellular regulation and disease pathology, new ideas and innovations emerge that promise significant advancements in health and biotechnology.
Innovations in Sequencing Technologies
Sequencing technologies have come a long way from their early days, and the trajectory indicates an exciting future. Innovations such as nanopore sequencing and advancements in single-molecule sequencing techniques are gaining traction.
- Nanopore Sequencing: This method allows real-time sequencing of RNA and has the potential to produce longer read lengths than traditional technologies. Its ability to sequence direct RNA makes it quite appealing because it captures modifications that can impact RNA stability and function.
- Single-Molecule Real-Time (SMRT) Sequencing: Similar to nanopore technology, SMRT sequencing provides insights into the dynamics of RNA molecules as they exist within the cellular environment. This innovation helps to dissect the complexities of transcriptomic landscapes with unprecedented clarity.
These technologies not only improve the accuracy of sequencing data but also cut down on the time required for sequencing processes. As a result, researchers can explore RNA's diverse functional landscape and its implications in various biological contexts more efficiently than ever.
Emerging Research Trends
In parallel with technological advancements, several emerging research trends are reshaping RNA analysis. Understanding these trends is vital for remaining at the forefront of RNA research. Some notable areas include:
- Integration of Multi-Omics Approaches: Combining RNA analysis with genomics, proteomics, and metabolomics is becoming essential. This integrative methodology enables a holistic view of cellular processes, offering insights that single-dimensional analyses do not.
- Targeted Therapeutics: Increased interest in using RNA-based therapeutics is evident, particularly in gene editing techniques like CRISPR/Cas9. As research progresses, RNA-targeting drugs are being developed to overcome diseases by directly addressing dysregulated RNA pathways.
- Single-Cell RNA Sequencing: As scientists strive to understand cellular heterogeneity, single-cell RNA sequencing is providing a fine-grained look at individual cell transcriptomes. This helps uncover the variability in gene expression and its role in various states of health and disease.
Understanding these emerging trends not only expands the horizons of RNA research but also ensures that scientists remain equipped to tackle the challenges of tomorrow.
The future of RNA analysis is not just in the technology alone, but it is also in the continuous learning and adaptation of researchers to new methodologies and theories. As the landscape changes, so must our approaches, ultimately leading to breakthroughs that could affect everything from fundamental biology to clinical applications.
By keeping an eye on these innovations and trends, researchers can better navigate the shifting terrain of RNA sequence analysis and emerge with impactful discoveries that carry forward the legacy of scientific inquiry.
Ending
As we draw the curtain on this exploration of RNA sequence analysis, it becomes clear that understanding this intricate field is vital for many reasons. First and foremost, RNA analysis serves as a cornerstone in the realms of genomics and molecular biology. Techniques developed over the years have shed light on how genes are expressed and regulated, facilitating breakthroughs that weren't possible a couple of decades ago.
Recap of Key Points
To summarise the insights gathered, several key elements stand out:
- Diverse Techniques: We examined varied RNA sequencing methods, including next-generation sequencing and single-cell analysis. Each method holds its unique value, catering to different research goals.
- Quality of Data: The importance of quality control cannot be overstated. Ensuring that the data collected is accurate and reliable forms the backbone of any subsequent analysis.
- Applications in Research: RNA sequence analysis has shown tremendous implications in disease research, from cancer to infectious diseases, leading to potential new therapies and better understanding of pathology.
- Future Innovations: New technologies and methodologies are emerging constantly, promising greater precision and efficiency in RNA analysis than ever before.
The Future of RNA Sequence Analysis in Science
Looking forward, the future of RNA sequence analysis is brimming with potential. Innovations in sequencing technologies, such as those that promise higher throughput and lower costs, are on the horizon. In parallel, a shift towards integrating artificial intelligence in bioinformatics tools is anticipated to streamline data analysis, making it accessible to a broader audience.
Furthermore, as the world of biology continues to evolve, we may see RNA sequence analysis expand its role not just in academic research, but in clinical settings as well. This shift could enhance personalized medicine approaches, tailoring treatments based on individual RNA profiles.